Enhancing point cloud semantic segmentation in the data-scarce domain of industrial plants through synthetic data
COMPUTER-AIDED CIVIL AND INFRASTRUCTURE ENGINEERING(2024)
摘要
Digitizing existing structures is essential for applying digital methods in architecture, engineering, and construction. However, the adoption of data-driven techniques for transforming point cloud data into useful digital models faces challenges, particularly in the industrial domain, where ground truth datasets for training are scarce. This paper investigates a solution leveraging synthetic data to train data-driven models effectively. In the investigated industrial domain, the complex geometry of building elements often leads to occlusions, limiting the effectiveness of conventional sampling-based synthetic data generation methods. Our approach proposes the automatic generation of realistic and semantically enriched ground truth data using surface-based sampling methods and laser scan simulation on industry-standard 3D models. In the presented experiments, we use a neural network for point cloud semantic segmentation to demonstrate that compared to sampling-based alternatives, simulation-based synthetic data significantly improve mean class intersection over union performance on real point cloud data, achieving up to 7% absolute increase.
更多查看译文
AI 理解论文
溯源树
样例
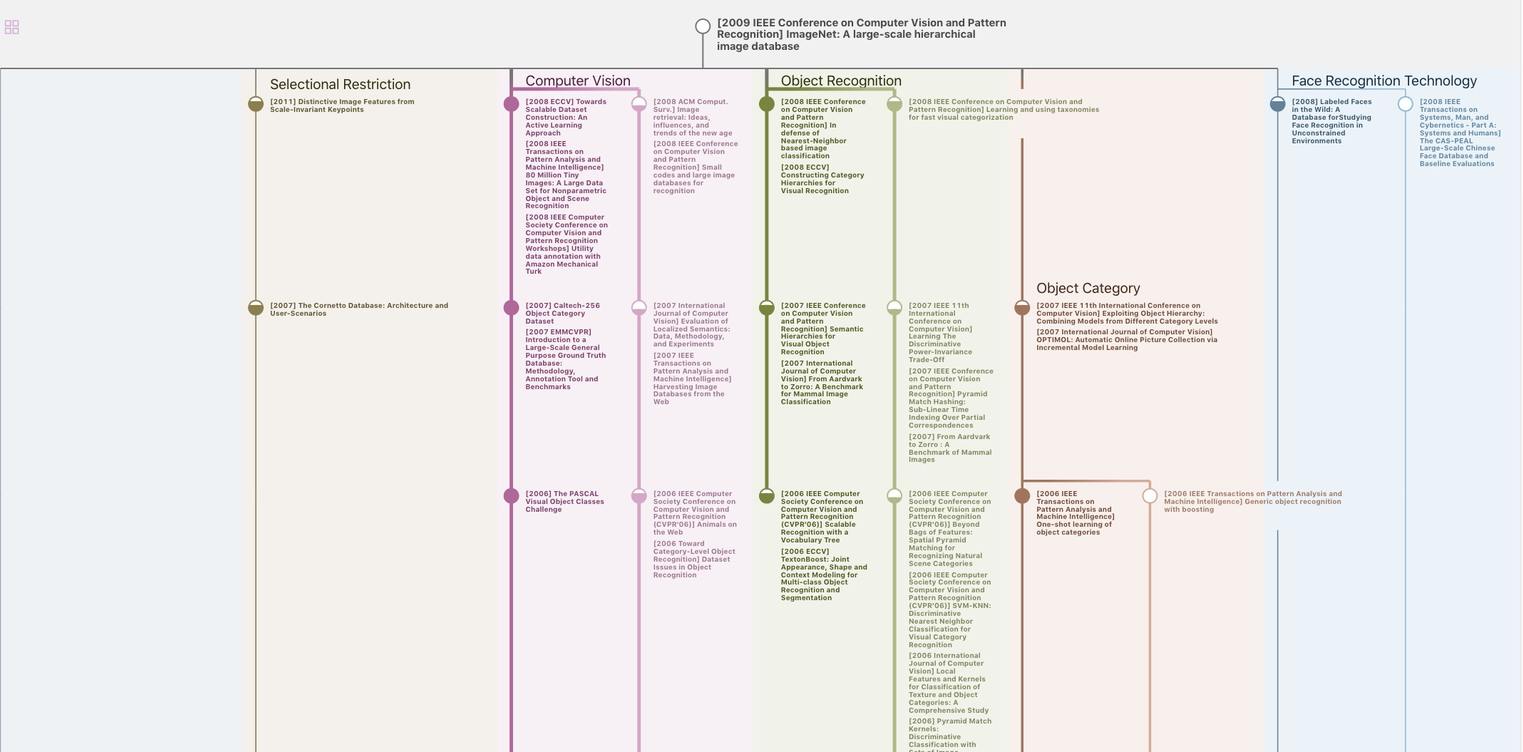
生成溯源树,研究论文发展脉络
Chat Paper
正在生成论文摘要