FEDGKD: Toward Heterogeneous Federated Learning via Global Knowledge Distillation
IEEE TRANSACTIONS ON COMPUTERS(2024)
摘要
Federated learning, as one enabling technology of edge intelligence, has gained substantial attention due to its efficacy in training deep learning models without data privacy and network bandwidth concerns. However, due to the heterogeneity of the edge computing system and data, many methods suffer from the "client-drift" issue that could considerably impede the convergence of global model training: local models on clients can drift apart, and the aggregated model can be different from the global optimum. To tackle this issue, one intuitive idea is to guide the local model training by global teachers, i.e., past global models, where each client learns the global knowledge from past global models via adaptive knowledge distillation techniques. Inspired by these insights, we propose a novel approach for heterogeneous federated learning, FedGKD, which fuses the knowledge from historical global models and guides local training to alleviate the "client-drift" issue. In this paper, we evaluate FedGKD through extensive experiments across various CV and NLP datasets (i.e., CIFAR-10/100, Tiny-ImageNet, AG News, SST5) under different heterogeneous settings. The proposed method is guaranteed to converge under common assumptions and outperforms the state-of-the-art baselines in the non-IID federated setting.
更多查看译文
关键词
Heterogeneous federated learning,non-IID,knowledge distillation,edge intelligence
AI 理解论文
溯源树
样例
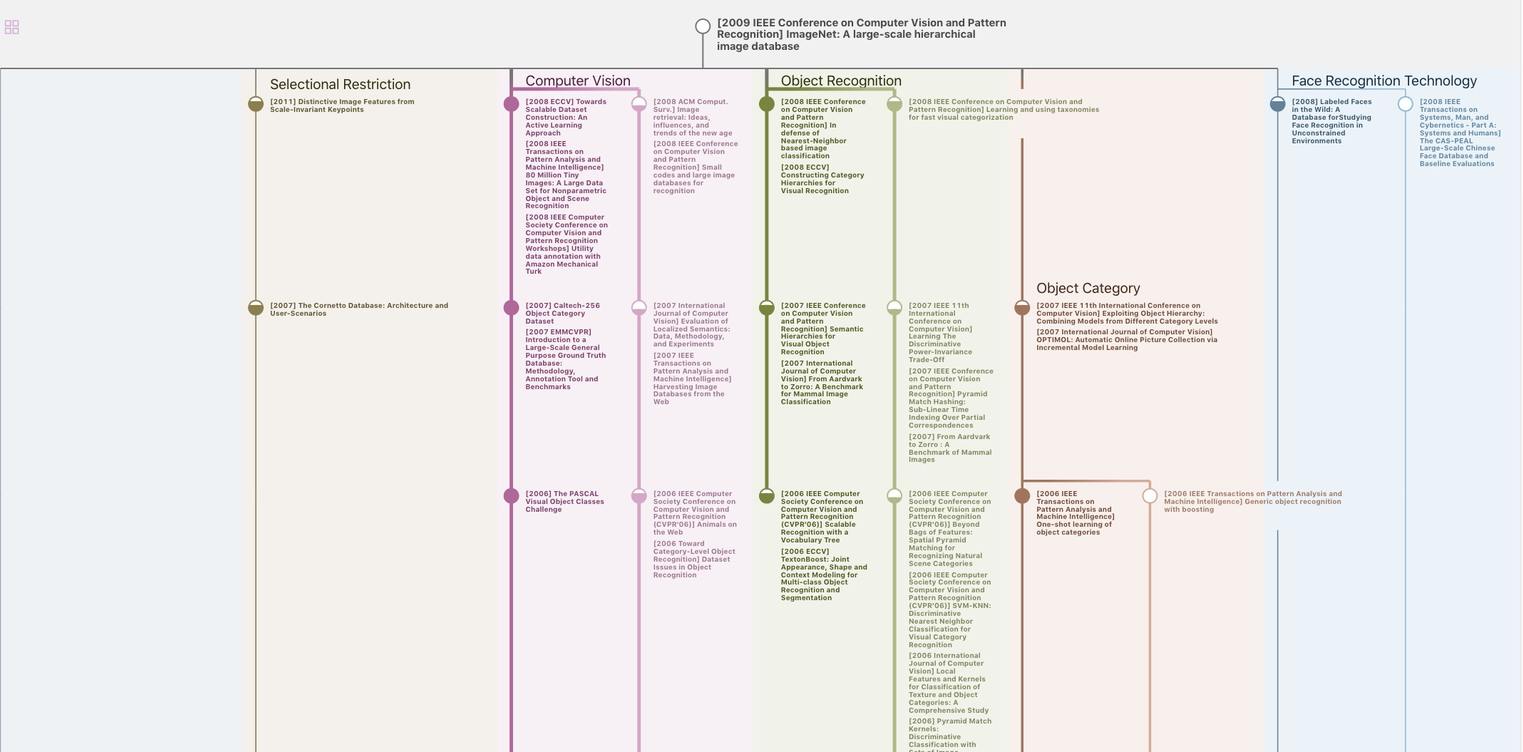
生成溯源树,研究论文发展脉络
Chat Paper
正在生成论文摘要