Machine learning in legal metrology-detecting breathalyzers' failures
MEASUREMENT SCIENCE AND TECHNOLOGY(2024)
摘要
Breathalyzers used at sobriety checkpoints undergo strict quality control by metrological institutes or police departments to ensure the accuracy of the results, thus avoiding measurement inaccuracies. This paper presents a new approach to instrument evaluation using machine learning algorithms that are capable of preemptively detecting failures. Our objective was to predict instrument failures before they occur. These faults may be errors or standard deviations that exceed the allowable limits defined by technical regulations. To predict these failures, we employed historical instrument measurement data and applied classification techniques to later label instruments as suitable or unsuitable. This was based on the instrument's potential not to fail or fail during its operation or before subsequent checks. To increase the reliability of failure prediction, we conducted fuel cell experiments to identify which instruments have cells that could compromise measurement results. To this end, we used the K-means clustering model, which identified two clusters based on the response signals during the ethanol redox reaction. The study concluded with a wear simulation on low-performance electrochemical cells to understand whether an adjustment to the calibration curve on instruments with these cells would not compromise the instrument's accuracy until the next check.
更多查看译文
关键词
breathalyzer,legal metrology,classification,clustering
AI 理解论文
溯源树
样例
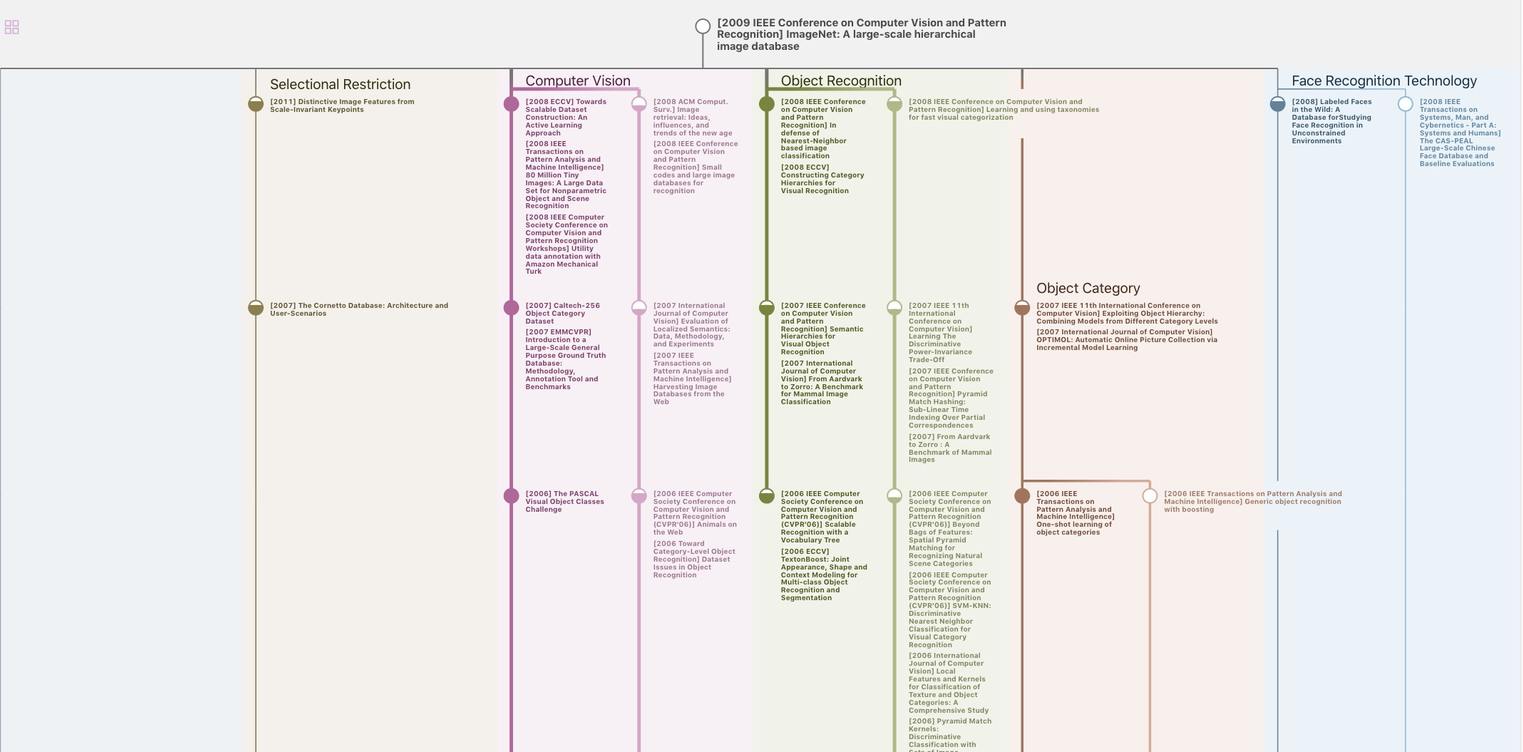
生成溯源树,研究论文发展脉络
Chat Paper
正在生成论文摘要