Prediction of transient emission characteristic from diesel engines based on CNN-GRU model optimized by PSO algorithm
ENERGY SOURCES PART A-RECOVERY UTILIZATION AND ENVIRONMENTAL EFFECTS(2024)
摘要
High-performance and low-cost emission characteristic prediction is very crucial for diesel engine design optimization and emission aftertreatment control and diagnosis. In this paper, a novel hybrid model that combines Convolutional Neural Network (CNN) and Gated Recurrent Unit Network (GRU) was proposed to predict the emission characteristic from diesel engines, encompassing CO, THC, CO2, NOx, exhaust temperature and exhaust pressure. Nine operating parameters from WHTC and WHSC cycles, including speed, torque, intake pressure, intake flow, intake temperature, oil pressure, fuel rate, oil temperature, water temperature, were considered as inputs. Firstly, the importance of each variable is evaluated by Random Forests algorithm to determine the optimal inputs for each emission characteristic parameter and reduce redundancy. Then the effect of different hyperparameters on the model performance was discussed in detail and PSO algorithm was used to obtain the optimal hyperparameters. Finally, the CNN-GRU hybrid model was assessed for its generalization and compared with ANN, LSTM and GRU models. The result demonstrates that the CNN-GRU hybrid model with PSO optimization has excellent prediction performance in either the training dataset or the validation dataset. The average value of R2 is 0.993 in the training dataset and 0.985 on the validation dataset. In the test dataset, the average R2 is 0.961, showing a minor decrease of 3.19% and 2.47% compared to the training and validation dataset, respectively. This indicates that the CNN-GRU hybrid model has strong generalization ability. Compared with other algorithms in the test dataset, the CNN-GRU hybrid model exhibits better comprehensive performance, with the average R2 value exceeding that of ANN, LSTM and only GRU by 5.96%, 2.69% and 3.23%, respectively.
更多查看译文
关键词
Convolutional neural networks,diesel engine,emission characteristic prediction,gated recurrent unit, random forests
AI 理解论文
溯源树
样例
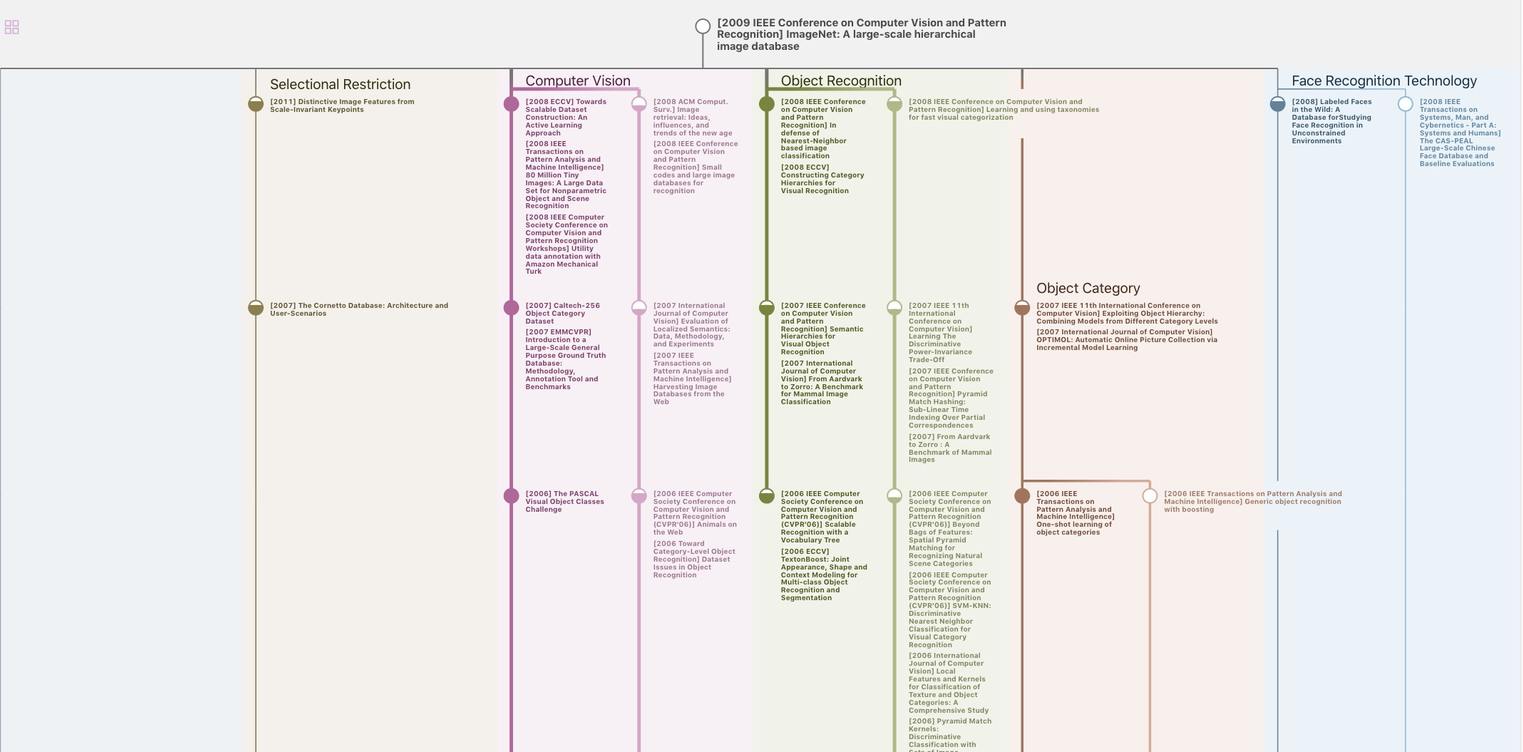
生成溯源树,研究论文发展脉络
Chat Paper
正在生成论文摘要