An adaptive sampling algorithm for reduced-order models using Isomap
INTERNATIONAL JOURNAL FOR NUMERICAL METHODS IN ENGINEERING(2024)
摘要
The use of reduced-order models (ROMs) in physics-based modeling and simulation is a popular tool for drastically lowering the computational cost associated with high-fidelity simulations. ROMs use training data from a set of computed high-fidelity simulations with different design parameters that control physical and geometric properties of the full-order model. The quality of the training data dictates the performance of the ROM, making the choice of training design parameters important. A widely used method for generating training parameters for ROMs is Latin hypercube sampling (LHS), a statistical method that aims to maximize the distance and minimize the correlation amongst produced samples. However, LHS fails to account for the physics of the full-order model and can lead to an over-representation of some physical regimes in the training data while neglecting others. In this work, we present a computationally efficient adaptive sampling method for ROMs using Isomap, a versatile algorithm for nonlinear dimensionality reduction. Using an initial number of samples, the adaptive sampling algorithm iteratively generates samples based on a low-dimensional manifold of the training data. When applied to two external aerodynamics problems, the proposed adaptive sampling algorithm offers significantly improved performance in predicting physical fields and coefficients of lift and drag for a given computational budget for both non-intrusive and projection-based ROMs.
更多查看译文
关键词
adaptive sampling,model reduction,non-intrusive,nonlinear dimensionality reduction,projection-based,proper orthogonal decomposition
AI 理解论文
溯源树
样例
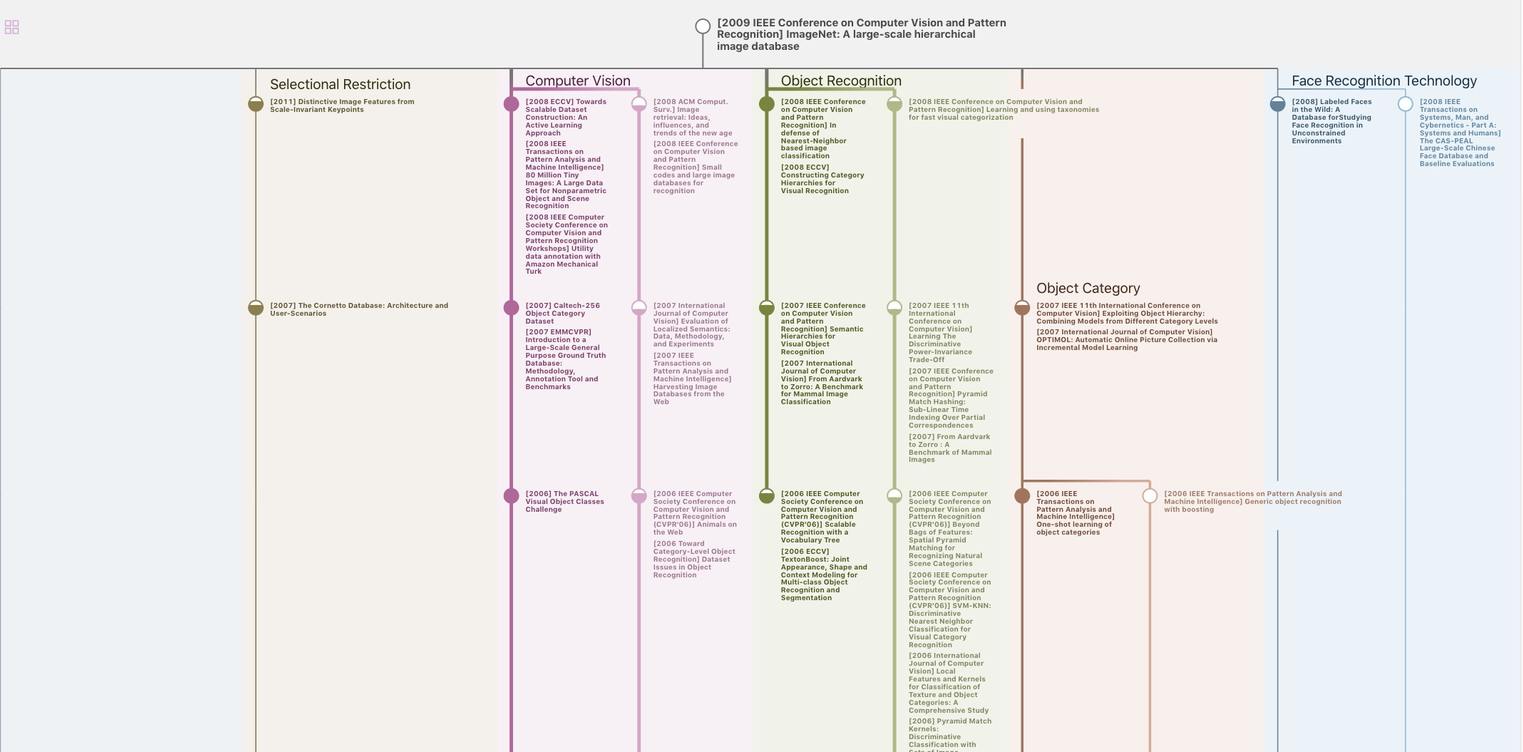
生成溯源树,研究论文发展脉络
Chat Paper
正在生成论文摘要