Deep transformer-based personalized dosimetry from SPECT/CT images: a hybrid approach for [177Lu]Lu-DOTATATE radiopharmaceutical therapy
EUROPEAN JOURNAL OF NUCLEAR MEDICINE AND MOLECULAR IMAGING(2024)
摘要
Purpose Accurate dosimetry is critical for ensuring the safety and efficacy of radiopharmaceutical therapies. In current clinical dosimetry practice, MIRD formalisms are widely employed. However, with the rapid advancement of deep learning (DL) algorithms, there has been an increasing interest in leveraging the calculation speed and automation capabilities for different tasks. We aimed to develop a hybrid transformer-based deep learning (DL) model that incorporates a multiple voxel S-value (MSV) approach for voxel-level dosimetry in [Lu-177]Lu-DOTATATE therapy. The goal was to enhance the performance of the model to achieve accuracy levels closely aligned with Monte Carlo (MC) simulations, considered as the standard of reference. We extended our analysis to include MIRD formalisms (SSV and MSV), thereby conducting a comprehensive dosimetry study. Methods We used a dataset consisting of 22 patients undergoing up to 4 cycles of [Lu-177]Lu-DOTATATE therapy. MC simulations were used to generate reference absorbed dose maps. In addition, MIRD formalism approaches, namely, single S-value (SSV) and MSV techniques, were performed. A UNEt TRansformer (UNETR) DL architecture was trained using five-fold cross-validation to generate MC-based dose maps. Co-registered CT images were fed into the network as input, whereas the difference between MC and MSV (MC-MSV) was set as output. DL results are then integrated to MSV to revive the MC dose maps. Finally, the dose maps generated by MSV, SSV, and DL were quantitatively compared to the MC reference at both voxel level and organ level (organs at risk and lesions). Results The DL approach showed slightly better performance (voxel relative absolute error (RAE) = 5.28 +/- 1.32) compared to MSV (voxel RAE = 5.54 +/- 1.4) and outperformed SSV (voxel RAE = 7.8 +/- 3.02). Gamma analysis pass rates were 99.0 +/- 1.2%, 98.8 +/- 1.3%, and 98.7 +/- 1.52% for DL, MSV, and SSV approaches, respectively. The computational time for MC was the highest (similar to 2 days for a single-bed SPECT study) compared to MSV, SSV, and DL, whereas the DL-based approach outperformed the other approaches in terms of time efficiency (3 s for a single-bed SPECT). Organ-wise analysis showed absolute percent errors of 1.44 +/- 3.05%, 1.18 +/- 2.65%, and 1.15 +/- 2.5% for SSV, MSV, and DL approaches, respectively, in lesion-absorbed doses. Conclusion A hybrid transformer-based deep learning model was developed for fast and accurate dose map generation, outperforming the MIRD approaches, specifically in heterogenous regions. The model achieved accuracy close to MC gold standard and has potential for clinical implementation for use on large-scale datasets
更多查看译文
关键词
Radiation dosimetry,Radionuclide therapy,Deep learning,Monte Carlo simulation,[Lu-177]Lu-DOTATATE
AI 理解论文
溯源树
样例
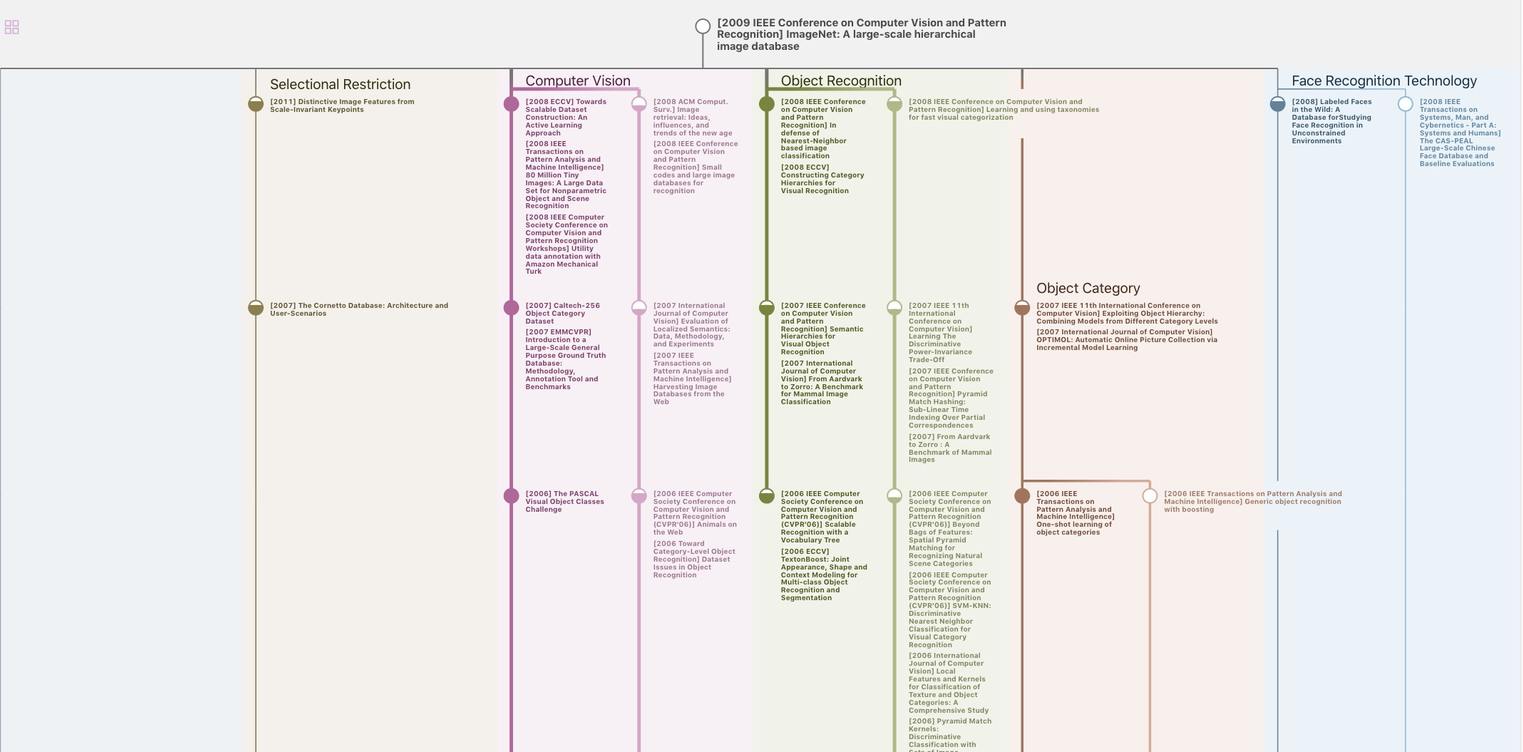
生成溯源树,研究论文发展脉络
Chat Paper
正在生成论文摘要