Collaborative Utilization of Sentinel-1/2 and DEM Data for Mapping the Soil Organic Carbon in Forested Areas Based on the Random Forest
FORESTS(2024)
摘要
Optical remote sensing data are widely used for constructing soil organic carbon (SOC) mapping models. However, it is challenging to map SOC in forested areas because atmospheric water vapor affects the results derived from optical remote sensing data. To address this issue, we utilized Sentinel-1, Sentinel-2, and digital elevation model (DEM) data to obtain a comprehensive feature set (including S1-based textural indices, S2-based spectral indices, and DEM-derived indices) to map the SOC content in forested areas. The features set were the predictor variables, and the measured SOC content was the dependent variable. The random forest algorithm was used to establish the SOC model. The ratio of performance to inter-quartile range (RPIQ) was 2.92 when the S2-based spectral indices were used as predictor variables. When the comprehensive feature set was utilized as the model input, the model achieved an RPIQ of 4.13 (R-2 = 0.91, root mean square error (RMSE) = 9.18), representing a 41.44% improvement in model accuracy. The average SOC content in the Greater Khingan Mountains was 43.75 g kg(-1). The northern and southwestern parts had higher SOC contents (>54.93 g kg(-1)), while the southeastern and northwestern parts had lower contents (<39.83 g kg(-1)). This discrepancy was primarily attributed to agricultural activities. The results indicate that using a comprehensive feature set and the random forest algorithm is a reliable approach for estimating the spatial distribution of the SOC content in forested areas and is suitable for forest ecology and carbon management studies.
更多查看译文
关键词
soil organic carbon,Sentinel-1,Sentinel-2,spatial distribution,random forest,forested area
AI 理解论文
溯源树
样例
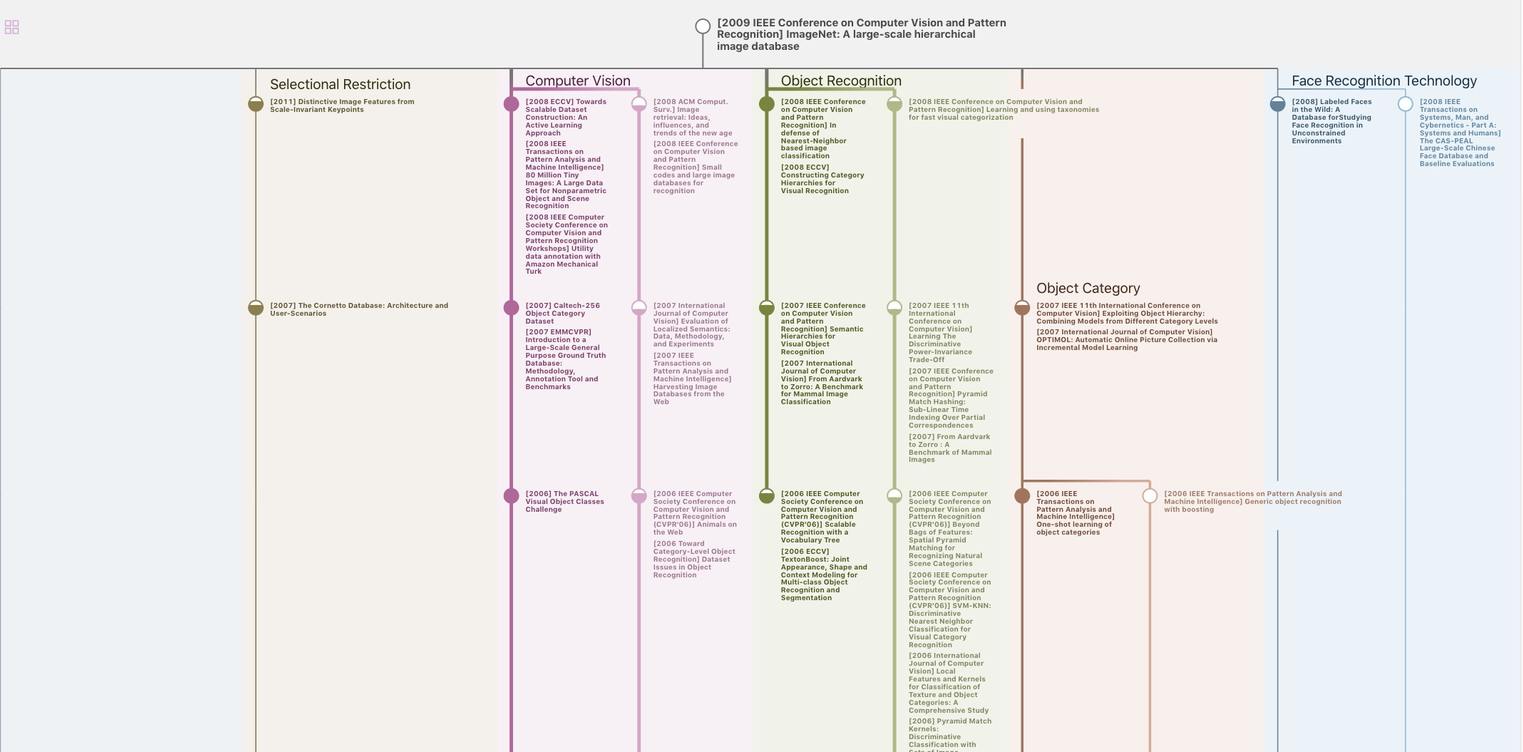
生成溯源树,研究论文发展脉络
Chat Paper
正在生成论文摘要