Latent Autoregressive Student-t Prior Process Models to Assess Impact of Interventions in Time Series
FUTURE INTERNET(2024)
摘要
With the advent of the "Internet of Things" (IoT), insurers are increasingly leveraging remote sensor technology in the development of novel insurance products and risk management programs. For example, Hartford Steam Boiler's (HSB) IoT freeze loss program uses IoT temperature sensors to monitor indoor temperatures in locations at high risk of water-pipe burst (freeze loss) with the goal of reducing insurances losses via real-time monitoring of the temperature data streams. In the event these monitoring systems detect a potentially risky temperature environment, an alert is sent to the end-insured (business manager, tenant, maintenance staff, etc.), prompting them to take remedial action by raising temperatures. In the event that an alert is sent and freeze loss occurs, the firm is not liable for any damages incurred by the event. For the program to be effective, there must be a reliable method of verifying if customers took appropriate corrective action after receiving an alert. Due to the program's scale, direct follow up via text or phone calls is not possible for every alert event. In addition, direct feedback from customers is not necessarily reliable. In this paper, we propose the use of a non-linear, auto-regressive time series model, coupled with the time series intervention analysis method known as causal impact, to directly evaluate whether or not a customer took action directly from IoT temperature streams. Our method offers several distinct advantages over other methods as it is (a) readily scalable with continued program growth, (b) entirely automated, and (c) inherently less biased than human labelers or direct customer response. We demonstrate the efficacy of our method using a sample of actual freeze alert events from the freeze loss program.
更多查看译文
关键词
Gaussian process regression,Student-t process,impact of interventions,IoT sensor data,time series analysis,variational inference
AI 理解论文
溯源树
样例
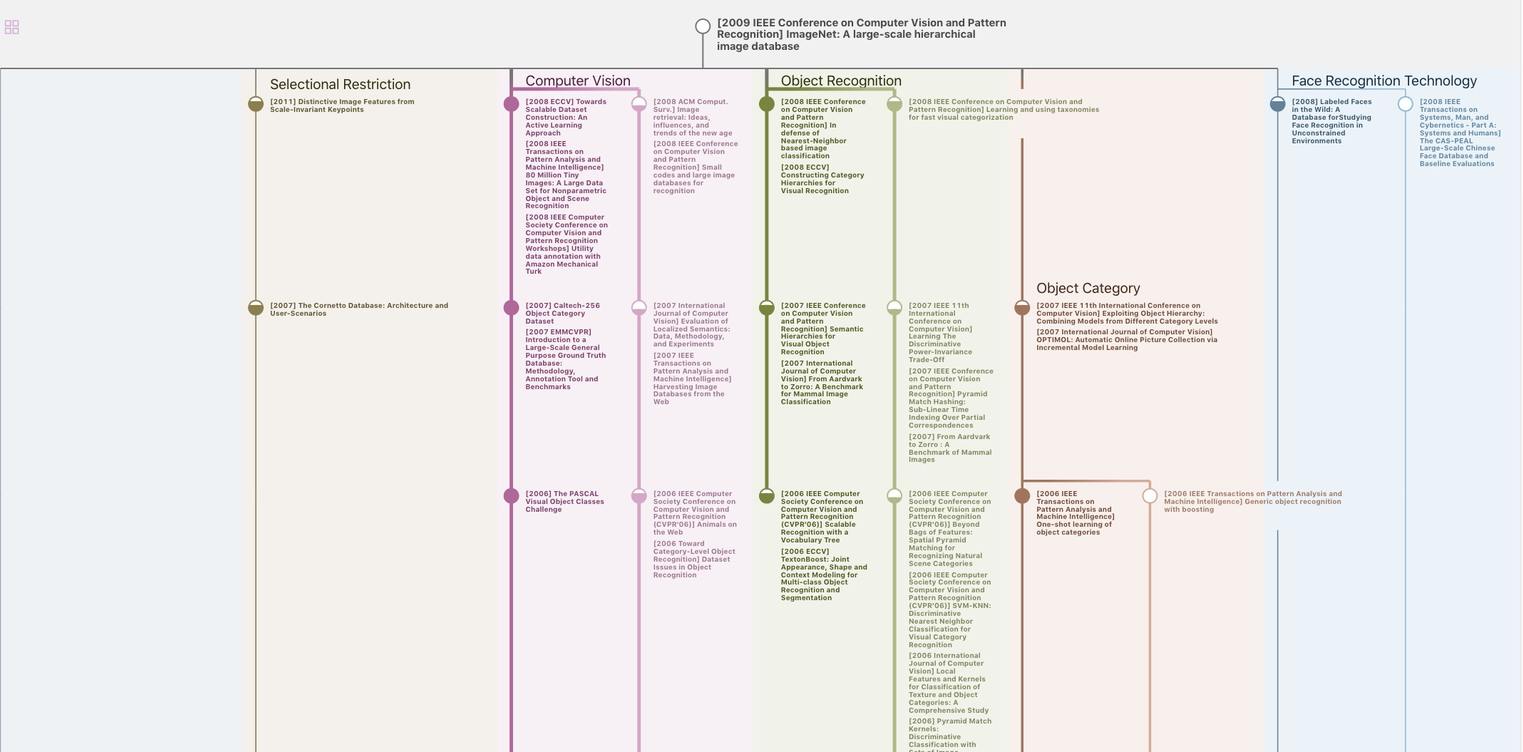
生成溯源树,研究论文发展脉络
Chat Paper
正在生成论文摘要