SuperPCA and 2-D compact variational mode decomposition for feature extraction from hyperspectral images
INTERNATIONAL JOURNAL OF REMOTE SENSING(2024)
摘要
The 2-D compact variational mode decomposition (2-D-C-VMD) has potential research significance in data mining of hyperspectral images (HSIs). The paper proposes a method of concatenation of features. First, the 2-D-C-VMD technique is used to decompose the HSIs to obtain smooth and clear boundary sub-band images when the 2-D-C-VMD takes the most preferred a priori parameter K x . Second, the optimal low-frequency component features of the image and the optimal high-frequency component constructed features are concatenated first and last, and the structure of the concatenation is constructed into a new fused data. Finally, in order to avoid the redundancy of the fused data band information from affecting the classification result, combine a recently developed simple and practical superpixelwise principal component analysis (SuperPCA) dimensionality reduction method to effectively reduce the dimensionality of the fused data. By comparing the analysis with relying only on the optimal low-frequency image feature information and with the direct linear superposition fusion that has been employed, it is verified that the concatenation of features approach proposed in this paper can obtain better recognition results.
更多查看译文
关键词
2-D-C-VMD,hyperspectral images (HSIs),concatenation,dimensionality reduction,SuperPCA
AI 理解论文
溯源树
样例
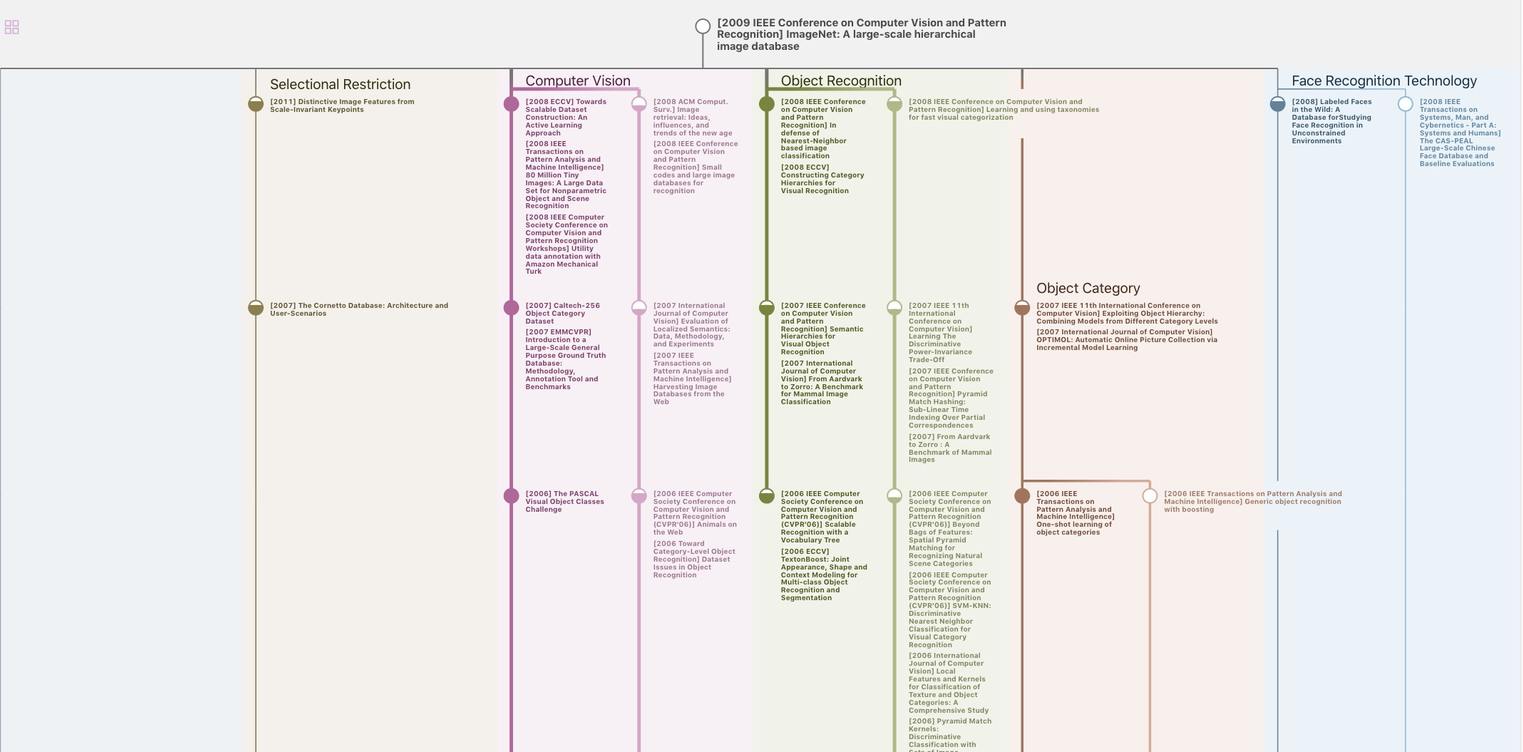
生成溯源树,研究论文发展脉络
Chat Paper
正在生成论文摘要