Interpretable Machine Learning Approaches for Forecasting and Predicting Air Pollution: A Systematic Review
AEROSOL AND AIR QUALITY RESEARCH(2024)
摘要
Many studies use machine learning to predict atmospheric pollutant levels, prioritizing accuracy over interpretability. This systematic review will focus on reviewing studies that have utilized interpretable machine learning models to enhance interpretability while maintaining high accuracy for air pollution prediction. The search terms "air pollution," "machine learning," and "interpretability" were used to identify relevant studies published between 2011 and 2023 from PubMed, Scopus, Web of Science, Science Direct, and JuSER. The included studies were assessed for quality based on an ecological checklist for maximizing reproducibility of ecological niche models. Among the 5,396 identified studies, 480 focused on air pollution prediction, with 56 providing model interpretations. Among the studies, 20 methods were identified: 8 model-agnostic methods, 4 model-specific methods, and 8 hybrid models. Shapley additive explanations was the most commonly used method (46.4%), followed by partial dependence plots (17.4%), both of which are model-agnostic methods. These methods identify important atmospheric features, enhancing researchers' understanding and making machine learning outcomes more accessible to non-experts. This can enhance prediction and prevention of adverse weather events and air pollution, benefiting public health.
更多查看译文
关键词
Air quality prediction,Deep learning,Supervised learning
AI 理解论文
溯源树
样例
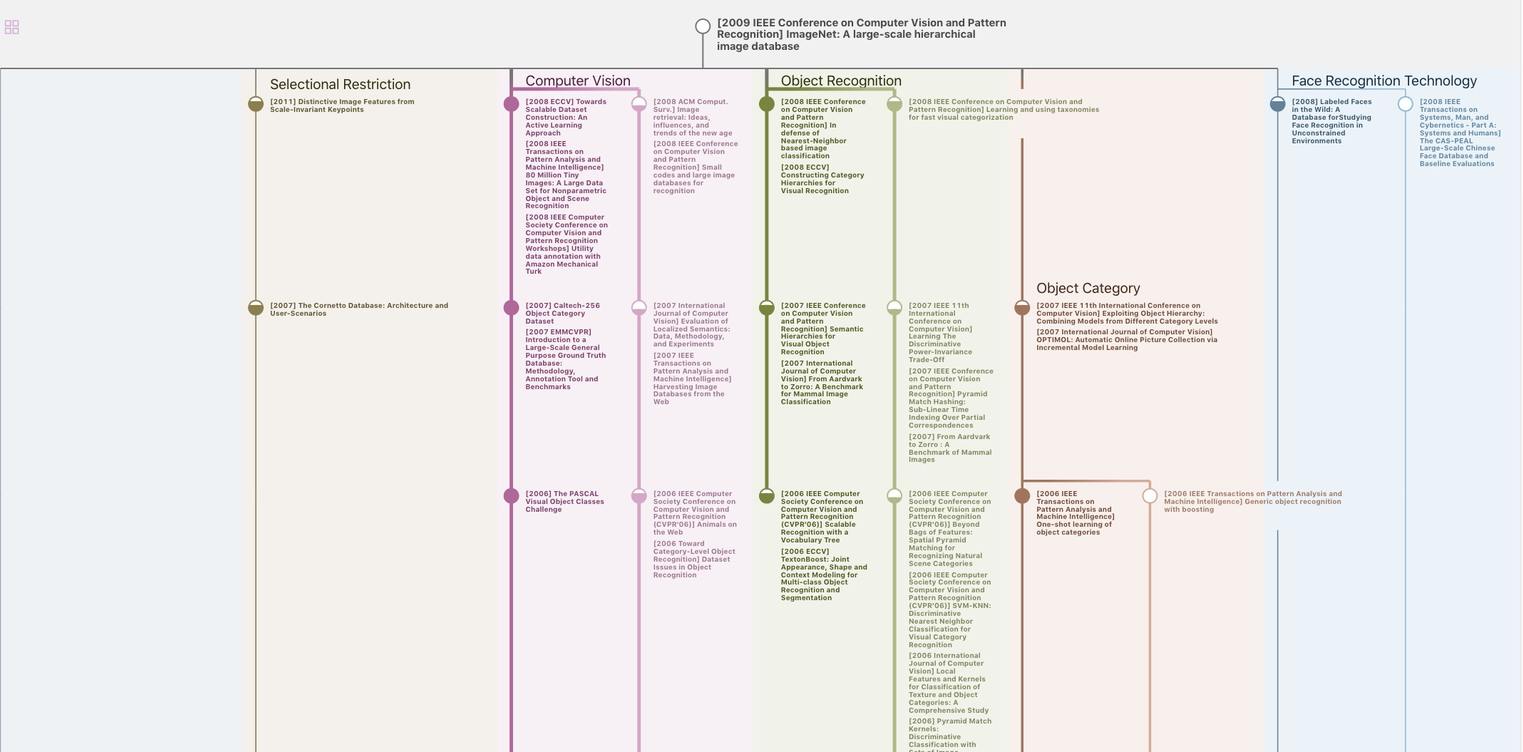
生成溯源树,研究论文发展脉络
Chat Paper
正在生成论文摘要