DECVAE: Data augmentation via conditional variational auto-encoder with distribution enhancement for few-shot fault diagnosis of mechanical system
MEASUREMENT SCIENCE AND TECHNOLOGY(2024)
摘要
Conditional variational autoencoder (CVAE) has the potential for few-sample fault diagnosis of mechanical systems. Nevertheless, the scarcity of faulty samples leads the augmented samples generated using CVAE suffer from limited diversity. To address the issue, a novel CVAE variant namely CVAE with distribution augmentation (DECVAE) is developed, to generate a set of high-quality augmented samples that are different but share very similar characteristics and categories with the corresponding real samples. First, DECVAE add a new sample distribution distance loss into the optimization objective of traditional CVAE. Amplifying this loss in training process can make the augmented samples cover a larger space, thereby improving diversity. Second, DECVAE introduces an auxiliary classifier into traditional CVAE to enhance the sensitivity to category information, keeping the augmented samples class invariance. Furthermore, to ensure that the information of edge-distributed samples can be fully learned and make augmented samples representative and authentic, a novel multi-model independent fine-tuning strategy is designed to train the DECVAE, which utilizes multiple independent models to fairly focus on all samples of the minority class during DECVAE training. Finally, the effectiveness of the developed DECVAE in few-shot fault diagnosis of mechanical systems is verified on a series of comparative experiments.
更多查看译文
关键词
data augmentation,conditional variational auto-encoder,deep learning,few-shot fault diagnosis
AI 理解论文
溯源树
样例
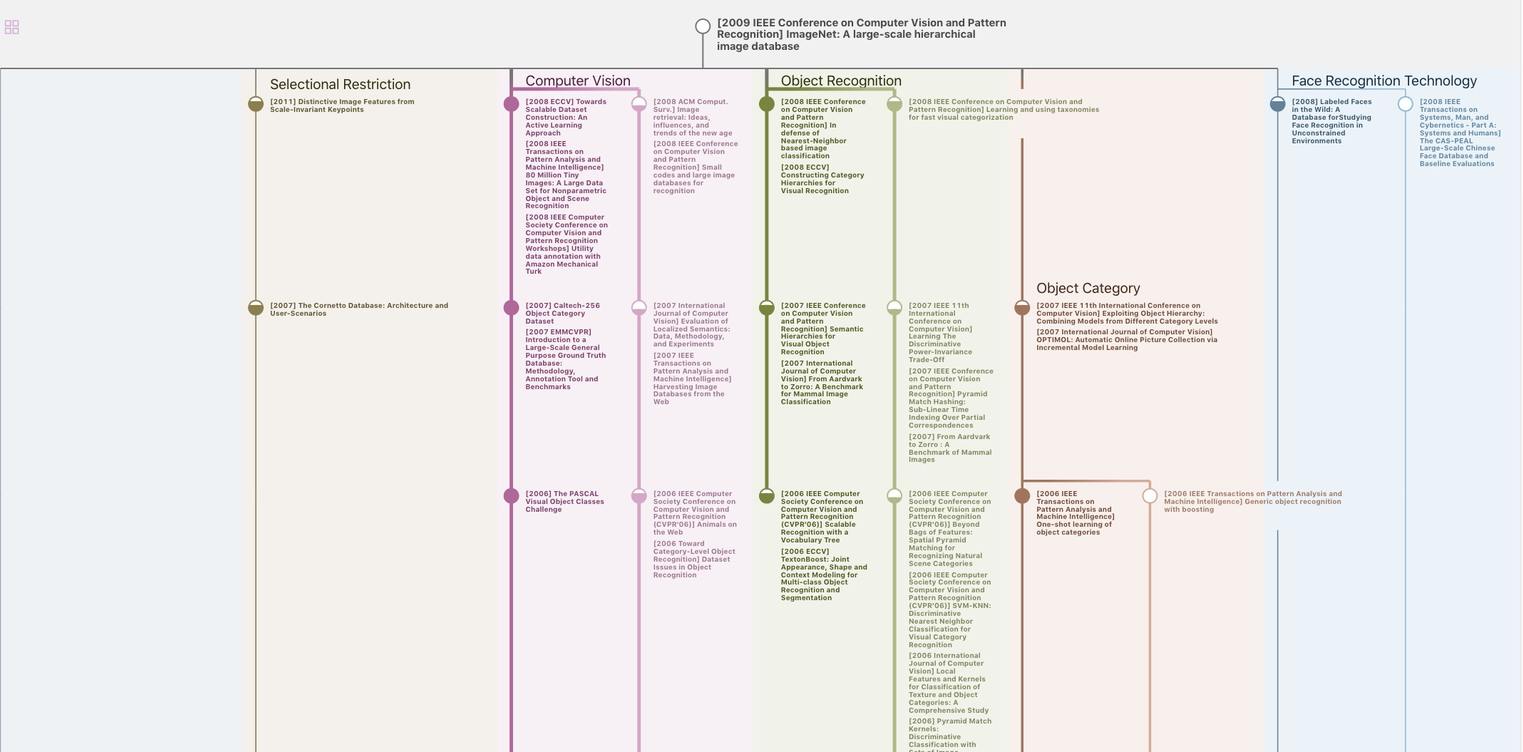
生成溯源树,研究论文发展脉络
Chat Paper
正在生成论文摘要