A multi-input parallel convolutional attention network for tool wear monitoring
INTERNATIONAL JOURNAL OF COMPUTER INTEGRATED MANUFACTURING(2023)
摘要
Effective tool wear monitoring is of great importance for machining process. With existing deep learning-based methods, the end-to-end model is often combined with sensor data to predict the state of the tool wear. These methods lack an effective mechanism to identify and highlight the important tool wear information in different sensor monitoring data, which limits the monitoring accuracy of the model. Therefore, a new deep prediction framework, called a multi-input parallel convolutional attention network (MPCAN), is proposed in this paper and used for intelligent tool wear monitoring. A multi-input parallel convolutional (MPC) network structure is developed to extract multi-scale features from monitoring data of various sensors. Efficient channel attention (ECA) is used to assign different attention weights to the tool wear information. A bidirectional long short-term memory (Bi-LSTM) is used to obtain the time series features related to tool wear. Finally, the predicted tool wear value is output through the fully connected network. An experimental analysis has been undertaken to illustrate, the effectiveness and superiority of the proposed method in improving the accuracy of the tool wear.
更多查看译文
关键词
Tool wear monitoring,deep learning,multi-input parallel convolution,bidirectional long short-term memory,attention mechanism
AI 理解论文
溯源树
样例
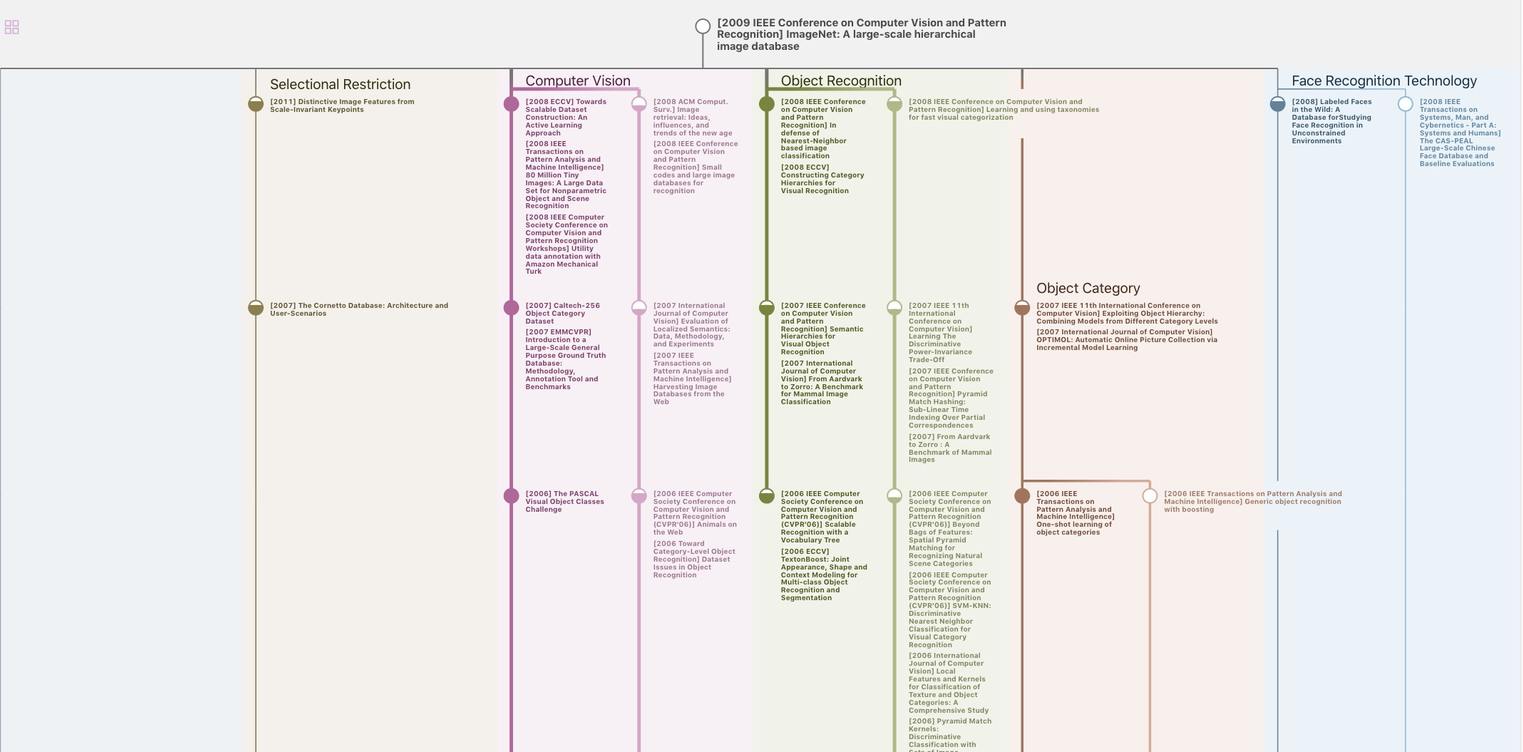
生成溯源树,研究论文发展脉络
Chat Paper
正在生成论文摘要