An innovative generalization method for data-driven models of steering feedback torque
PROCEEDINGS OF THE INSTITUTION OF MECHANICAL ENGINEERS PART D-JOURNAL OF AUTOMOBILE ENGINEERING(2023)
摘要
Accurately modeling steering feedback torque (SFT) is crucial for the performance of both steer-by-wire systems and driving simulators of vehicles. Physics-based methods have poor model accuracy and real-time performance, due to the model complexity and unknown or inaccurate model parameters. Therefore data-driven methods have gained increasing attention in recent years for their advantages on SFT modeling. However, developing satisfactory data-driven models requires a large amount of data with sufficient coverage on various driving conditions, which can be time-consuming and expensive. To address this issue, this paper proposes an innovative generalization method that can transfer an SFT model trained for a specific vehicle to other target vehicles, dramatically reducing the dependence on large amounts of data and the coverage of various driving conditions, thus, saving time and cost. First, a pre-trained SFT model with high accuracy and strong generalization capability is built based on large, full-coverage data from source domain vehicles. Then, a network is designed to fine-tune the pre-trained model with a small amount of data from target domain vehicles with only a few driving conditions. To identify the most suitable driving conditions for training the fine-tuning network, the performance of five networks trained by different driving conditions is compared. Finally, a control network with the same structure as the fine-tuned network is trained based on the same target domain data, and the model accuracy of the transfer network and the controlled network under different conditions is compared and analyzed. The proposed transfer learning method along with the designed transfer network is proved to be effective on improving prediction accuracy of the target domain by 58%-74.7% compared to the pre-trained network.
更多查看译文
关键词
Steering feedback torque,data-driven,generalization,transfer learning
AI 理解论文
溯源树
样例
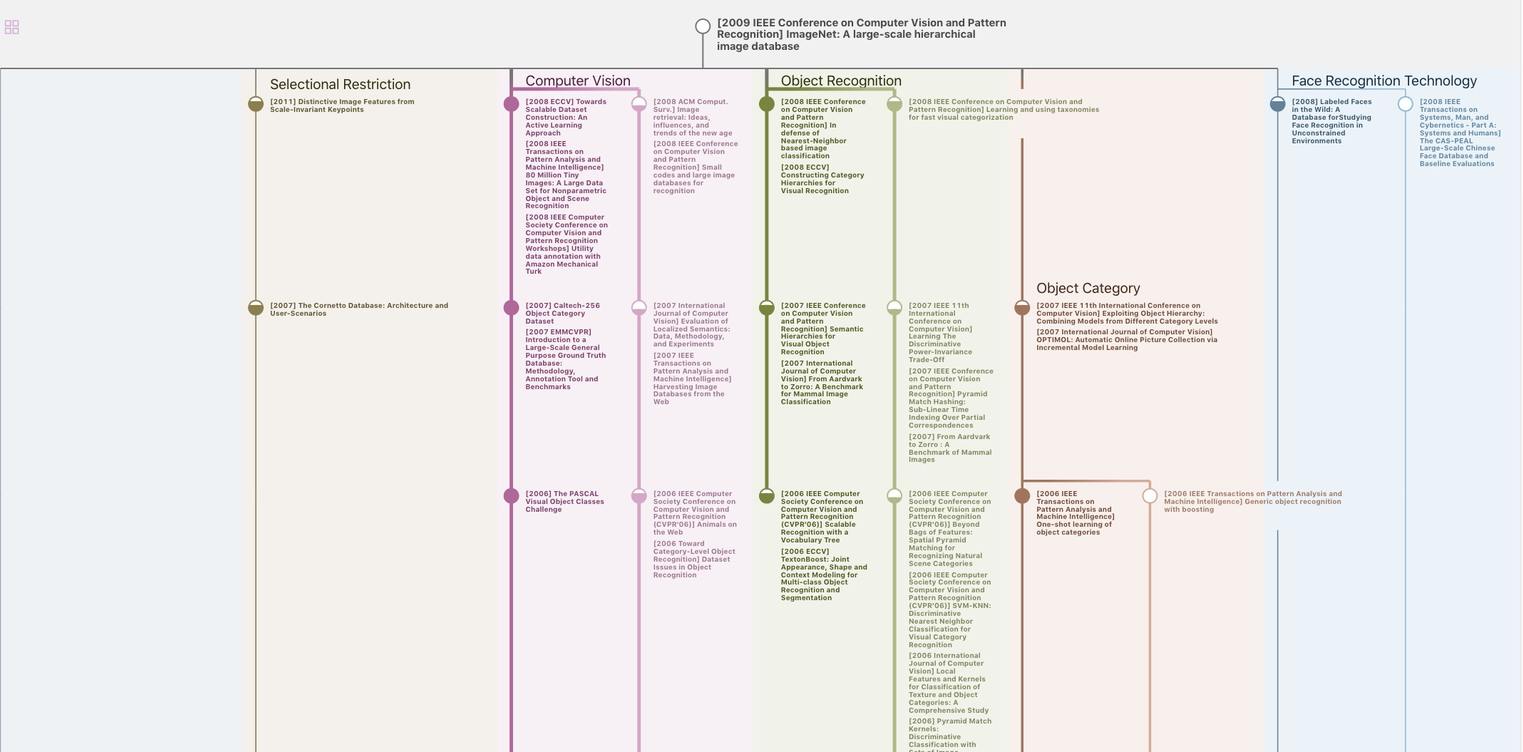
生成溯源树,研究论文发展脉络
Chat Paper
正在生成论文摘要