Score-ranking smooth average precision loss for remote sensing image retrieval
JOURNAL OF SPATIAL SCIENCE(2024)
摘要
In remote sensing image retrieval task, deep metric learning loss is an effective method for learning semantic similarity relationships between images. However, most deep metric learning losses treat different ranked images equally. Therefore, we propose a novel score-ranking smooth average precision (SRSAP) loss to improve the retrieval performance. Firstly, the score-ranking (SR) loss builds an ensemble-based similarity structure by using all images. Secondly, we utilize smooth AP to assign more weight to higher-ranked images. Finally, the joint loss function is utilized to fine-tune the network. The experiments show that SRSAP loss is distinctly superior to usual deep metric losses.
更多查看译文
关键词
Remote sensing image retrieval,deep metric learning,score-ranking smooth AP loss
AI 理解论文
溯源树
样例
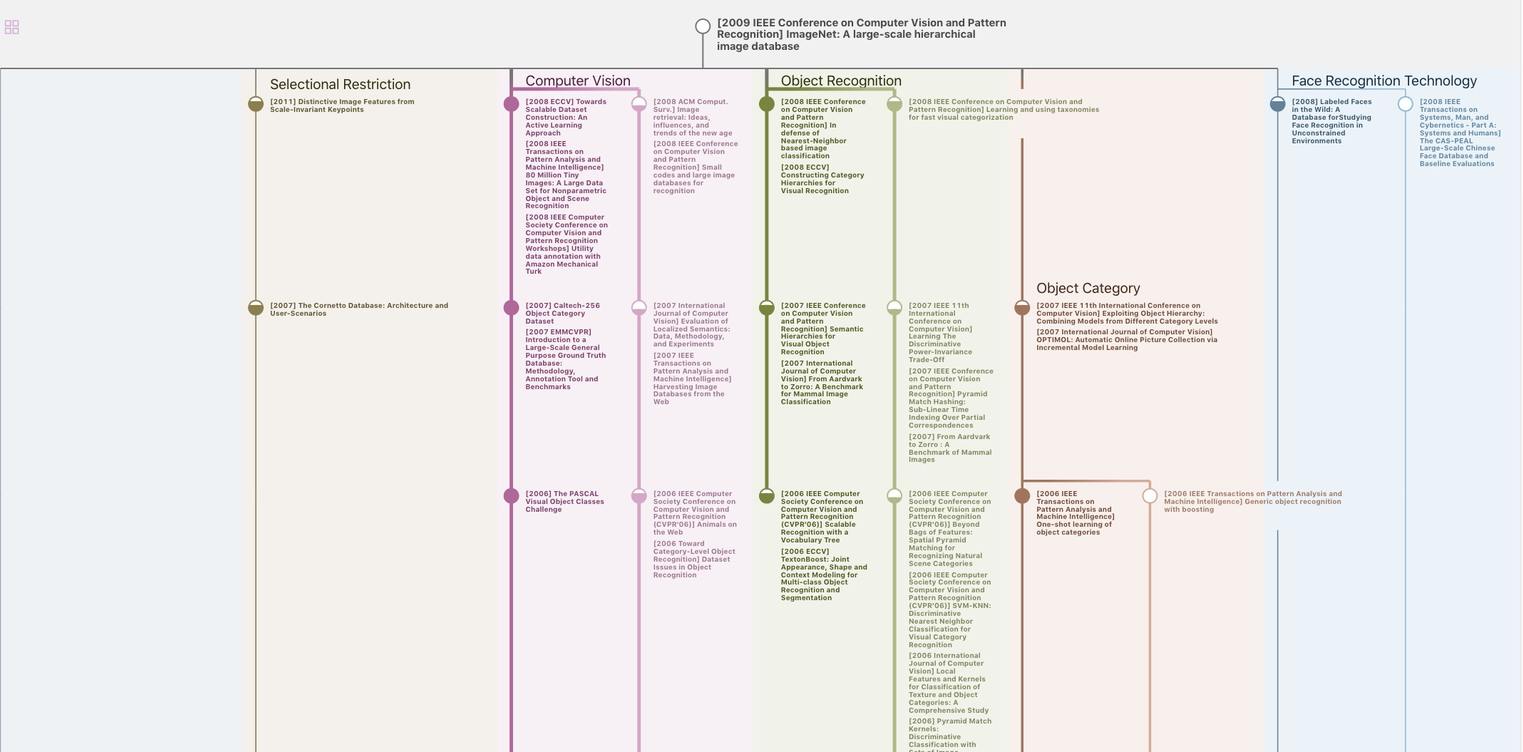
生成溯源树,研究论文发展脉络
Chat Paper
正在生成论文摘要