A Novel Adversarial Detection Method for UAV Vision Systems via Attribution Maps
DRONES(2023)
摘要
With the rapid advancement of unmanned aerial vehicles (UAVs) and the Internet of Things (IoTs), UAV-assisted IoTs has become integral in areas such as wildlife monitoring, disaster surveillance, and search and rescue operations. However, recent studies have shown that these systems are vulnerable to adversarial example attacks during data collection and transmission. These attacks subtly alter input data to trick UAV-based deep learning vision systems, significantly compromising the reliability and security of IoTs systems. Consequently, various methods have been developed to identify adversarial examples within model inputs, but they often lack accuracy against complex attacks like C&W and others. Drawing inspiration from model visualization technology, we observed that adversarial perturbations markedly alter the attribution maps of clean examples. This paper introduces a new, effective detection method for UAV vision systems that uses attribution maps created by model visualization techniques. The method differentiates between genuine and adversarial examples by extracting their unique attribution maps and then training a classifier on these maps. Validation experiments on the ImageNet dataset showed that our method achieves an average detection accuracy of 99.58%, surpassing the state-of-the-art methods.
更多查看译文
关键词
UAVs vision systems,adversarial examples detection,deep learning,attribution map
AI 理解论文
溯源树
样例
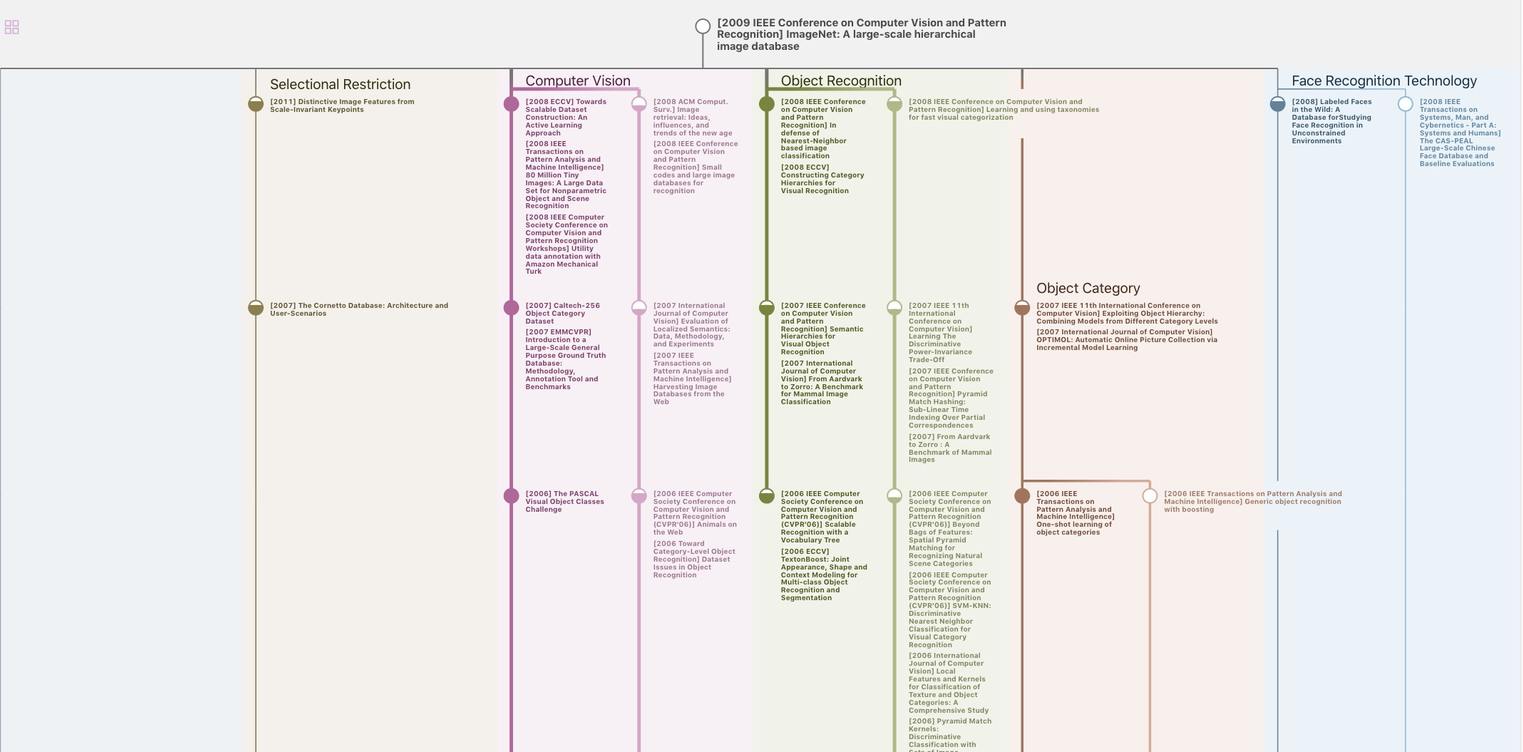
生成溯源树,研究论文发展脉络
Chat Paper
正在生成论文摘要