A Nature-Inspired Method to Mine Top-k Multi-Level High-Utility Itemsets
CYBERNETICS AND SYSTEMS(2023)
摘要
High-Utility Itemset Mining (HUIM) is designed to discover sets of itemsets that can bring high profits from the database. However, HUIM encounters several challenges in picking a suitable minimum utility threshold for each database. A class of algorithms that select the top-k itemsets based on their utility has been proposed to address this issue. Although traditional top-k HUI mining algorithms do not require a specific threshold, they tend to be very time-consuming and memory-intensive when dealing with large datasets. To tackle the combinational complexity involved in HUIM algorithms, nature-inspired methods have been suggested and adopted. Nonetheless, these algorithms have traditionally focused on handling conventional, often overlooking critical data structures like product hierarchies. Consequently, they fail to extract crucial insight from this novel database format. Thus, our research introduces a heuristic-based algorithm designed to leverage top-k itemsets from databases enriched with item taxonomy data. We propose a technique involving the early pruning of unpromising items to enhance mining efficiency. Experimental evaluations are conducted on several datasets to assess the method's performance, both with and without adopting this strategy, demonstrating its effectiveness.
更多查看译文
关键词
Cross-entropy,data mining,high-utility itemset mining,multi-level abstract database,top-k multi-level high-utility itemset
AI 理解论文
溯源树
样例
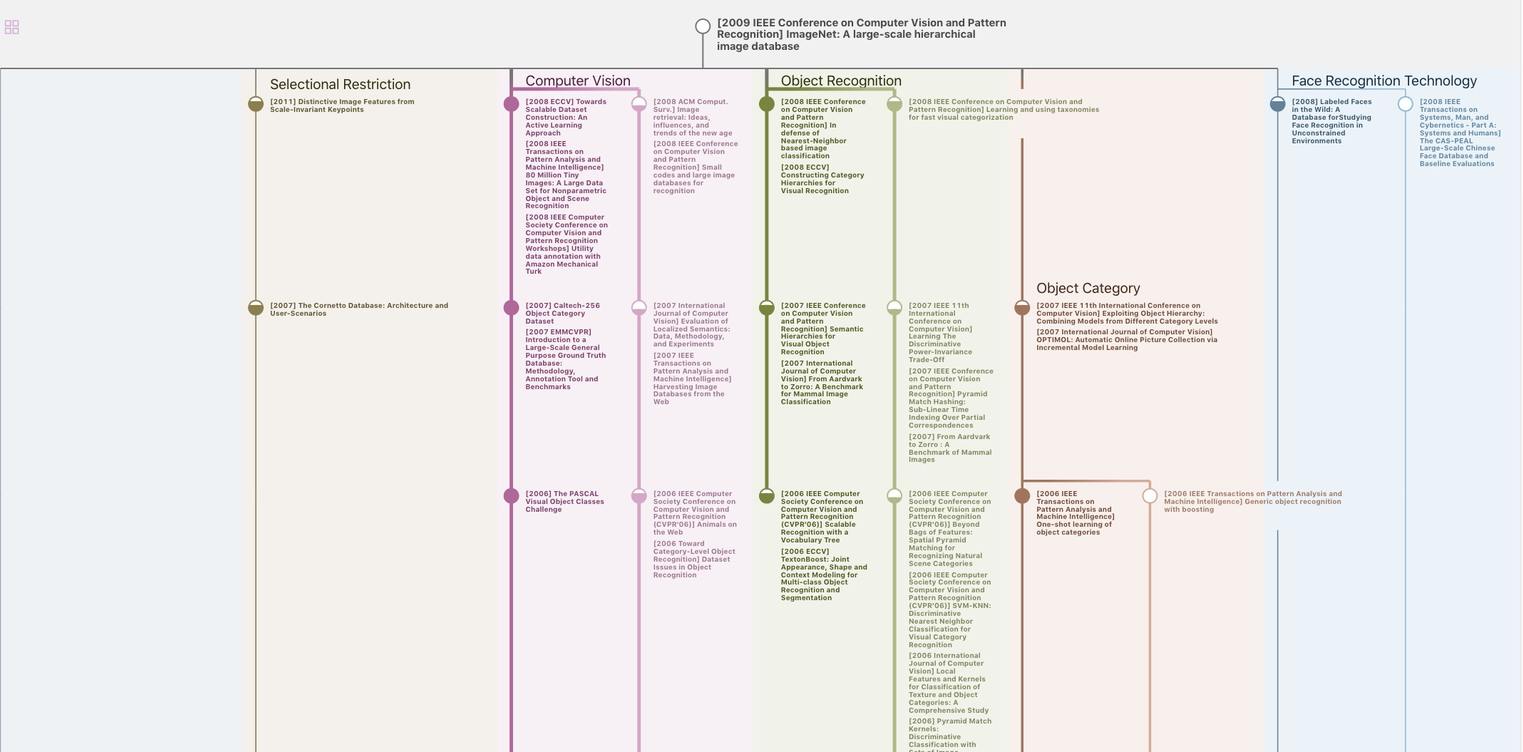
生成溯源树,研究论文发展脉络
Chat Paper
正在生成论文摘要