Machine Learning for Two-Phase Gas-Liquid Flow Regime Evaluation Based on Raw 3D ECT Measurement Data
BULLETIN OF THE POLISH ACADEMY OF SCIENCES-TECHNICAL SCIENCES(2023)
摘要
This paper presents a study on applying machine learning algorithms for classification of two-phase flow regime and its internal structures. This research results may be used in adjusting optimal control of air pressure and liquid flow rate to pipeline and process vessels. To achieve this goal the model of an artificial neural network was built and trained using measurement data acquired from 3D Electrical Capacitance Tomography (ECT) measurement system. Because the set of measurement data collected to build the AI model was insufficient, the new approach dedicated to data augmentation had to be developed. The main goal of the research was to examine the high adaptability of the Artificial Neural Network (ANN) model in the case of emergency state and measurement system errors. Another goal was to test if it can resist unforeseen problems and correctly predict the flow type or detect these failures. It may help to avoid any pernicious damage and finally to compare its accuracy to the fuzzy classifier based on reconstructed tomography images - authors' previous work.
更多查看译文
关键词
neural networks,machine learning,data augmentation,electrical capacitance tomography,industrial process monitoring
AI 理解论文
溯源树
样例
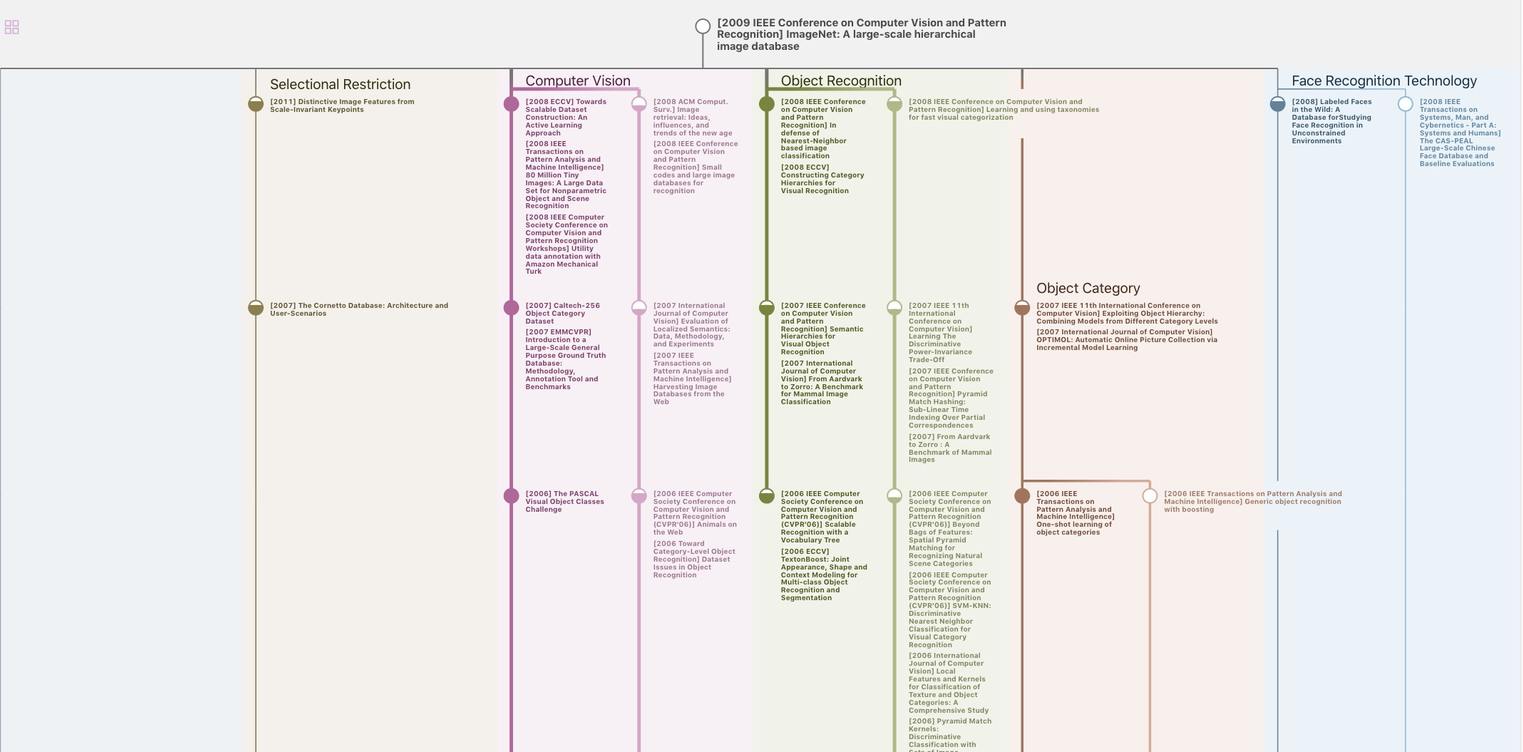
生成溯源树,研究论文发展脉络
Chat Paper
正在生成论文摘要