How recommendation letters affect career Mobility:Evidence from a social networking sites LinkedIn
COMPUTERS IN HUMAN BEHAVIOR(2024)
摘要
Social networking sites (SNSs) provide users with ample opportunities to share their own information and participate in social browsing to get to know others. Drawing upon signaling theory, this paper investigated how and to what extent recommendation letters' content information (breadth and depth) and external information (field and relationship) affect career mobility. Based on the open data from LinkedIn, this paper adopted the Latent Dirichlet Allocation (LDA) method to capture the breadth and depth of the recommendation letter, and used elaboration likelihood model to test our model. The results show that both content information and external information of the recommendation letter have significant positive impacts on the career mobility. In addition, the social network size shown converse moderating effects between recommendation letter content information and career mobility. This paper extends the boundaries of signaling theory by creatively using machine learning methods to measure textual signals (i.e., recommendation letter). The research results provide practical implications for personal career development and firm talent management.
更多查看译文
关键词
Career mobility,Recommendation letter,Signaling theory,ELM model,LDA model,Resume data
AI 理解论文
溯源树
样例
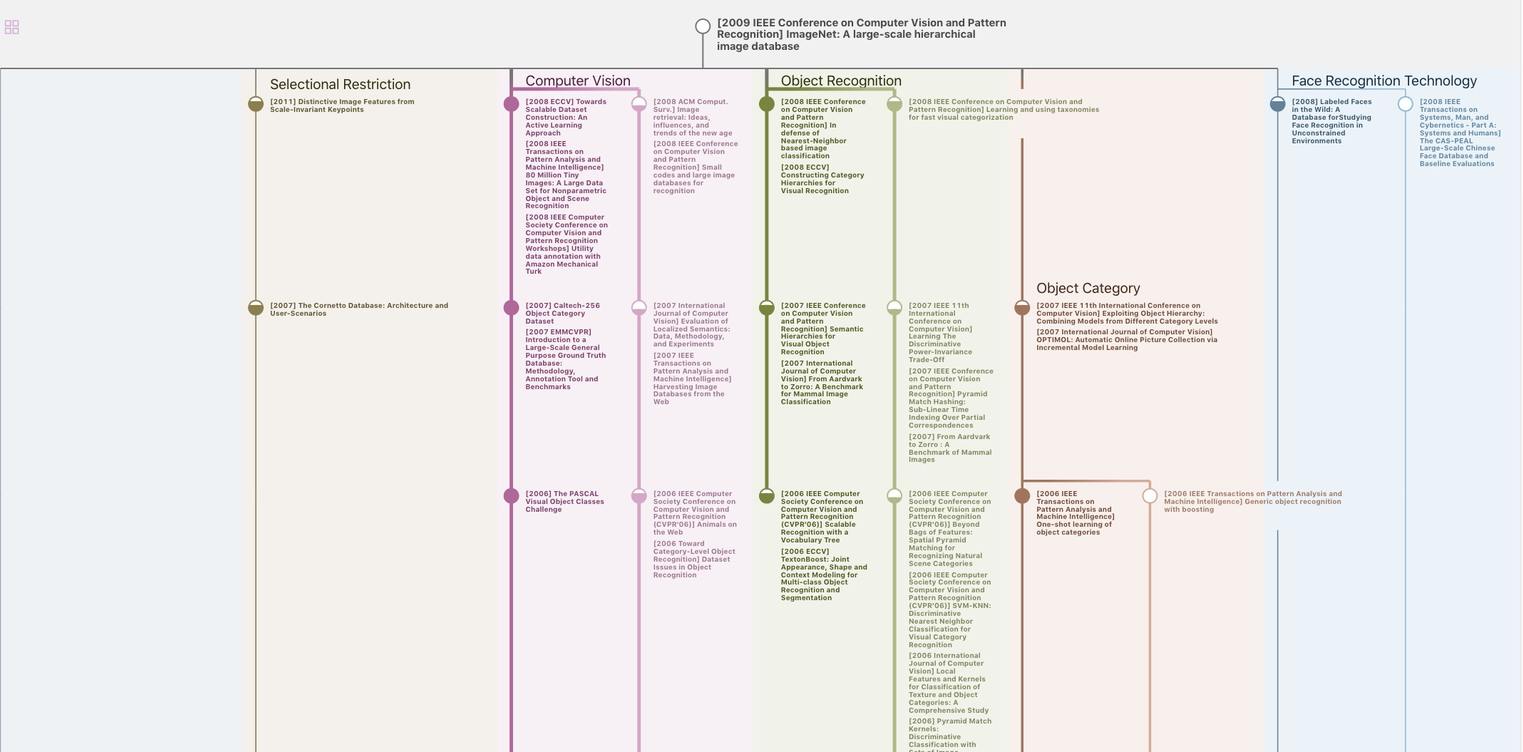
生成溯源树,研究论文发展脉络
Chat Paper
正在生成论文摘要