Robust generalized scalar-on-tensor regression
IISE TRANSACTIONS(2023)
摘要
High-dimensional (HD) data, such as images and profiles, are commonly collected from complex systems and contain significant explanatory and predictive information for effective systems monitoring and control. Therefore, developing accurate and robust predictive models based on HD data is crucial. In the literature, various methods, including linear scalar-on-tensor regression, are developed to model a complex system based on HD data. However, existing estimation techniques ignore the presence of outliers and are prone to biased estimations. This article proposes a robust scalar-on-tensor regression framework that handles multi-dimensional HD input data when the data contain outliers. Our proposed estimation method is constructed using maximum Lq-likelihood estimation instead of the classic maximum likelihood estimation. The asymptotic analysis under the Gaussian distribution assumption and the guideline on choosing the tuning parameter of our proposed method is provided. Several simulations and case studies evaluate the proposed method's efficacy compared to several benchmark methods in the literature.
更多查看译文
关键词
Robust estimator,scalar-on-tensor regression,Lq-likelihood,asset lifetime prediction
AI 理解论文
溯源树
样例
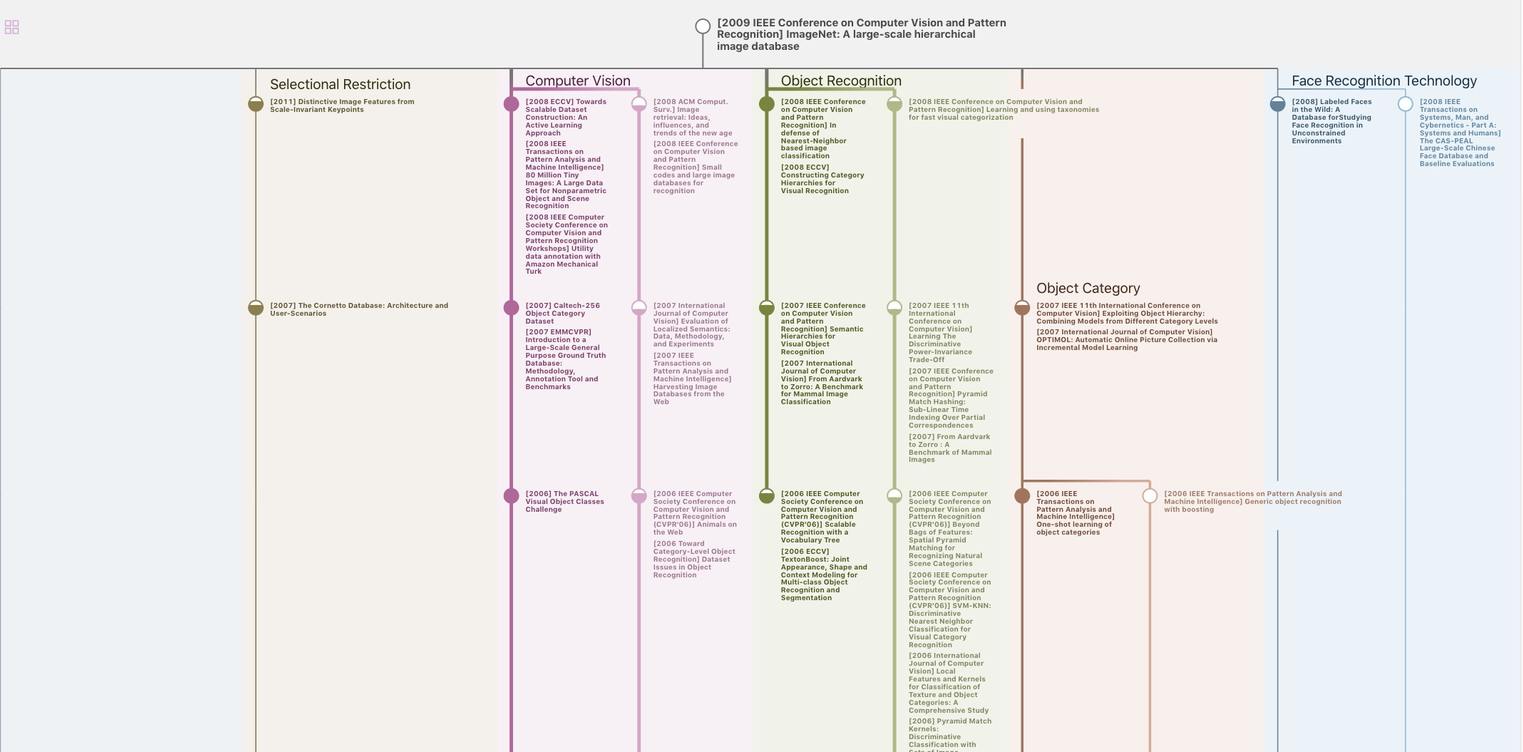
生成溯源树,研究论文发展脉络
Chat Paper
正在生成论文摘要