Intelligent Environment-Adaptive GNSS/INS Integrated Positioning with Factor Graph Optimization
REMOTE SENSING(2024)
摘要
Global navigation satellite systems (GNSSs) applied to intelligent transport systems in urban areas suffer from multipath and non-line-of-sight (NLOS) effects due to the signal reflections from high-rise buildings, which seriously degrade the accuracy and reliability of vehicles in real-time applications. Accordingly, the integration between GNSS and inertial navigation systems (INSs) could be utilized to improve positioning performance. However, the fixed GNSS solution uncertainty of the conventional integration method cannot determine the fluctuating GNSS reliability in fast-changing urban environments. This weakness becomes solvable using a deep learning model for sensing the ambient environment intelligently, and it can be further mitigated using factor graph optimization (FGO), which is capable of generating robust solutions based on historical data. This paper mainly develops the adaptive GNSS/INS loosely coupled system on FGO, along with the fixed-gain Kalman filter (KF) and adaptive KF (AKF) being taken as comparisons. The adaptation is aided by a convolutional neural network (CNN), and the feasibility is verified using data from different grades of receivers. Compared with the integration using fixed-gain KF, the proposed adaptive FGO (AFGO) maintains the 100% positioning availability and reduces the overall 2D positioning error by up to 70% in the aspects of both root mean square error (RMSE) and standard deviation (STD).
更多查看译文
关键词
GNSS,urban canyon,deep learning,multipath effect,factor graph optimization (FGO)
AI 理解论文
溯源树
样例
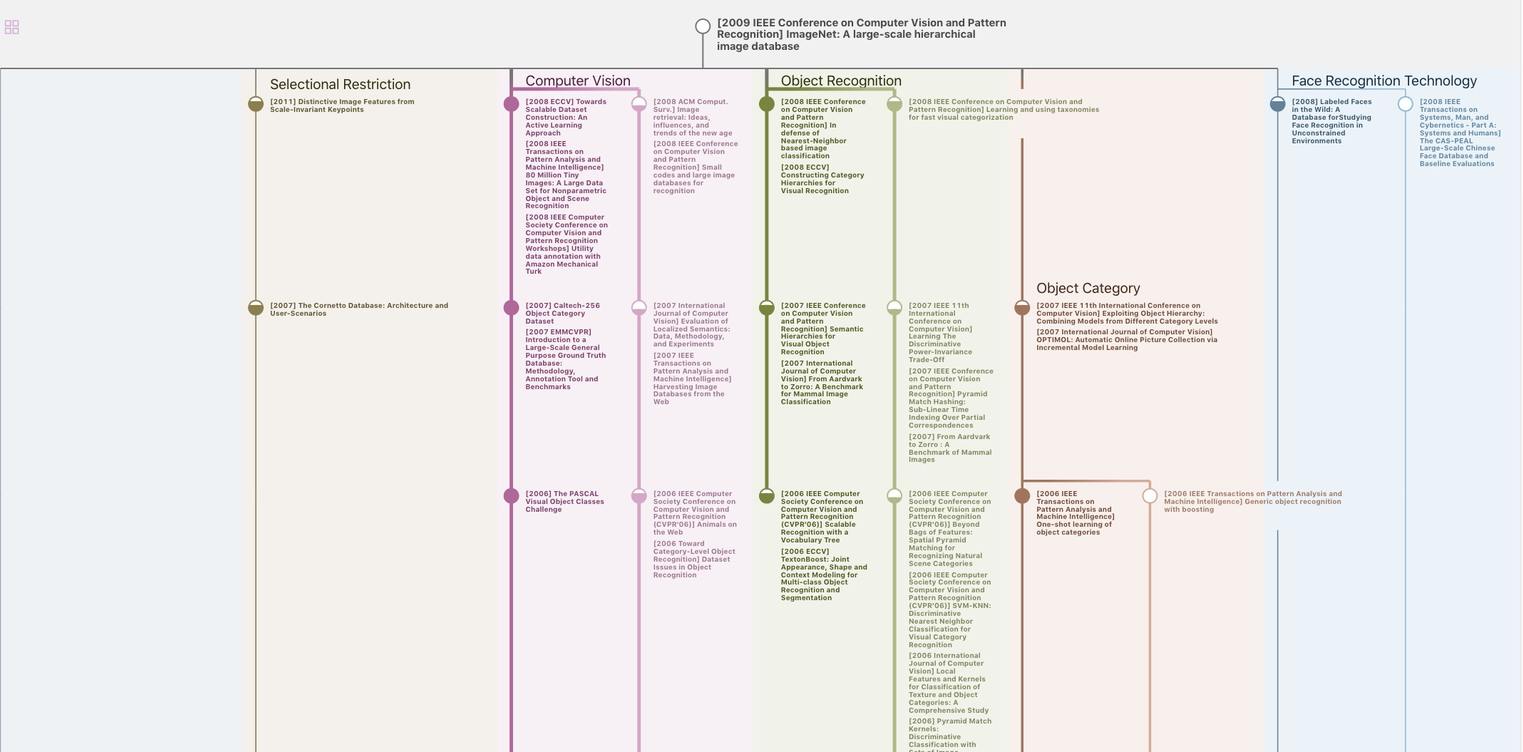
生成溯源树,研究论文发展脉络
Chat Paper
正在生成论文摘要