A hybrid combination of CNN Attention with optimized random forest with grey wolf optimizer to discriminate between Arabic hateful, abusive tweets
JOURNAL OF KING SAUD UNIVERSITY-COMPUTER AND INFORMATION SCIENCES(2024)
摘要
Arabic hateful speech recognition has long been a major area of focus in Natural Language Processing (NLP) research. In light of recent advancements in transformer models and deep learning, researchers are now turning to transfer learning techniques based on existing models such as BERT for Arabic hateful speech recognition. To detect Arabic hateful contexts, using advanced machine learning algorithms and NLP techniques is essential. These techniques can help to detect different forms of hateful contexts in Arabic by analyzing the text for lexical, semantic, and syntactic features. In this research, we proposed a new hybrid approach that combines deep and machine learning models to detect hateful and abusive content in Arabic. The proposed model consists of a combination of convolutional neural networks and attention layers that are trained to differentiate between normal, abusive, and hateful contexts in Arabic. In the first step, we used a pre-trained model to extract features from the hateful Arabic context. After that, we used an optimized random forest combined with particle swarm optimization and grey wolf optimizer to classify the extracted features. Finally, we evaluated the performance of the model to detect hateful Arabic contexts. To evaluate the proposed method we used 5846 and 6023 tweets with 3 categories of hateful, abusive, and normal Arabic contexts. The experimental result indicates 97.16% accuracy, 97.15% F1 -score, 97.17% precision, and 97.13% sensitivity using CNN Attention + optimized random forest by the grey wolf optimizer on 5846 tweets. 97.83% accuracy, 97.83% F1 -score, 97.84% precision, and 97.83% sensitivity have been reported CNN Attention + optimized random forest by the grey wolf optimizer on 6023 tweets.
更多查看译文
关键词
Arabic natural language processing,Deep learning,Machine learning,Grey wolf optimizer
AI 理解论文
溯源树
样例
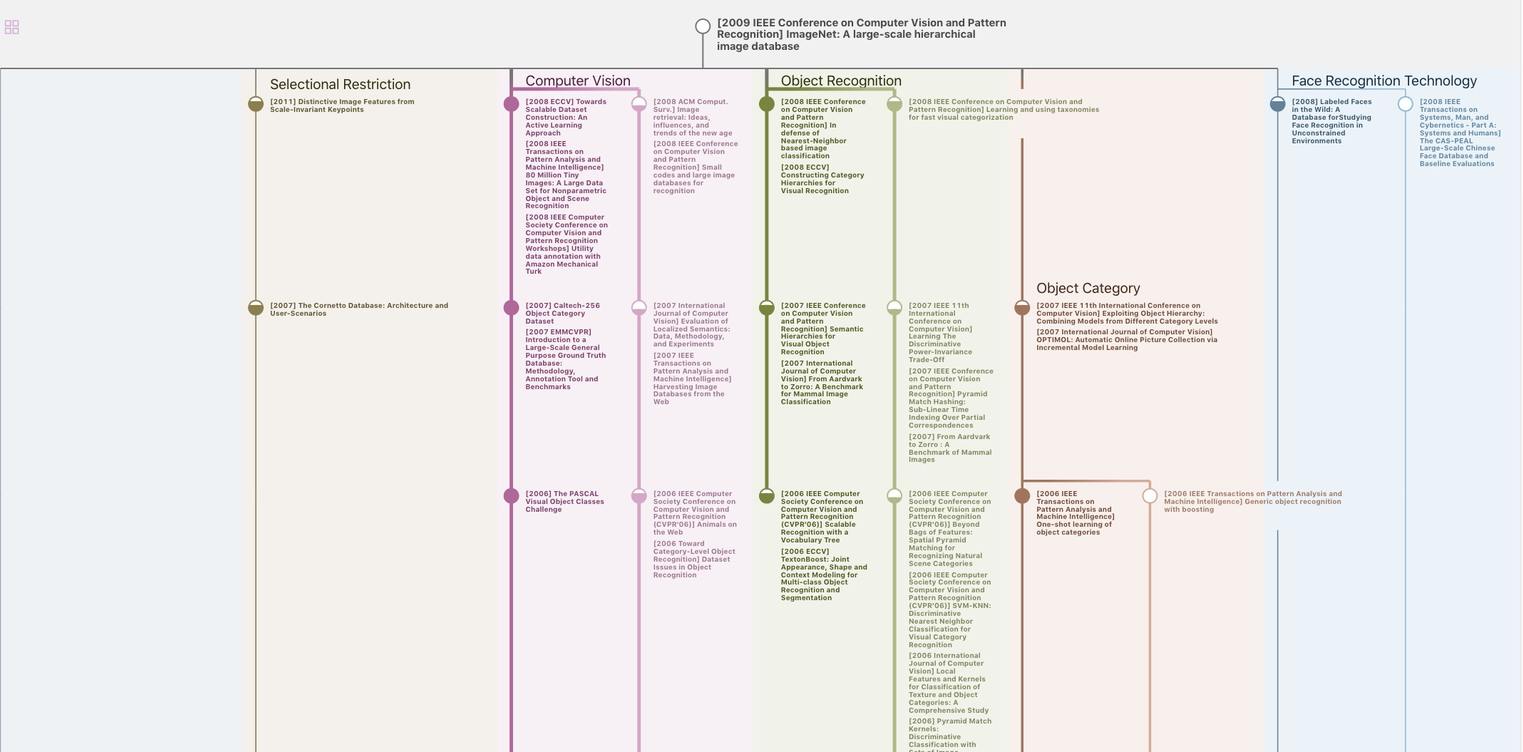
生成溯源树,研究论文发展脉络
Chat Paper
正在生成论文摘要