A Mask R-CNN Network for Wide-Area Mining Subsidence Automatic Detection With InSAR Observations
IEEE TRANSACTIONS ON GEOSCIENCE AND REMOTE SENSING(2024)
摘要
Land subsidence caused by mining activity is one of the most serious anthropogenic geohazards. The rapid detection and continuous monitoring of mining subsidence facilitate the swift detection of geohazards. The traditional methods of monitoring mining subsidence have shortcomings, such as offering only a limited coverage and being time consuming. Interferometric Synthetic Aperture Radar (InSAR) has been proven to be a powerful tool to identify mining subsidence hazards from unwrapped interferograms, but this method can be complex and inefficient, particularly for wide areas. In this article, a mask R-CNN model is presented to automatically detect mining subsidence and monitor the surface activity over wide areas using original SAR interferograms to avoid the time-consuming and error-prone phase unwrapping procedure. Using Sentinel-1 wrapped interferograms as the real dataset and simulated wrapped interferograms generated with the Gaussian surface function combined with Generic Atmospheric Correction Online Service (GACOS) for InSAR as the simulated dataset, the mask R-CNN deep neural network was used to train the mining subsidence detection model. It turned out that the accuracy of the detection model was 91.48%, while the precision was 96.44%, the recall rate was 93.88%, and the F1 index was 0.949. The detection model was then utilized to detect mining subsidence in southwestern Shanxi, China, between 2016 and 2022. A total of 152 land subsidence points were detected and long-term monitoring results were also obtained. An analysis of the state of land subsidence in the mining areas was carried out to obtain land subsidence activity during the monitoring period.
更多查看译文
关键词
InSAR,mask R-CNN,mining subsidence,surface activity,time series detection
AI 理解论文
溯源树
样例
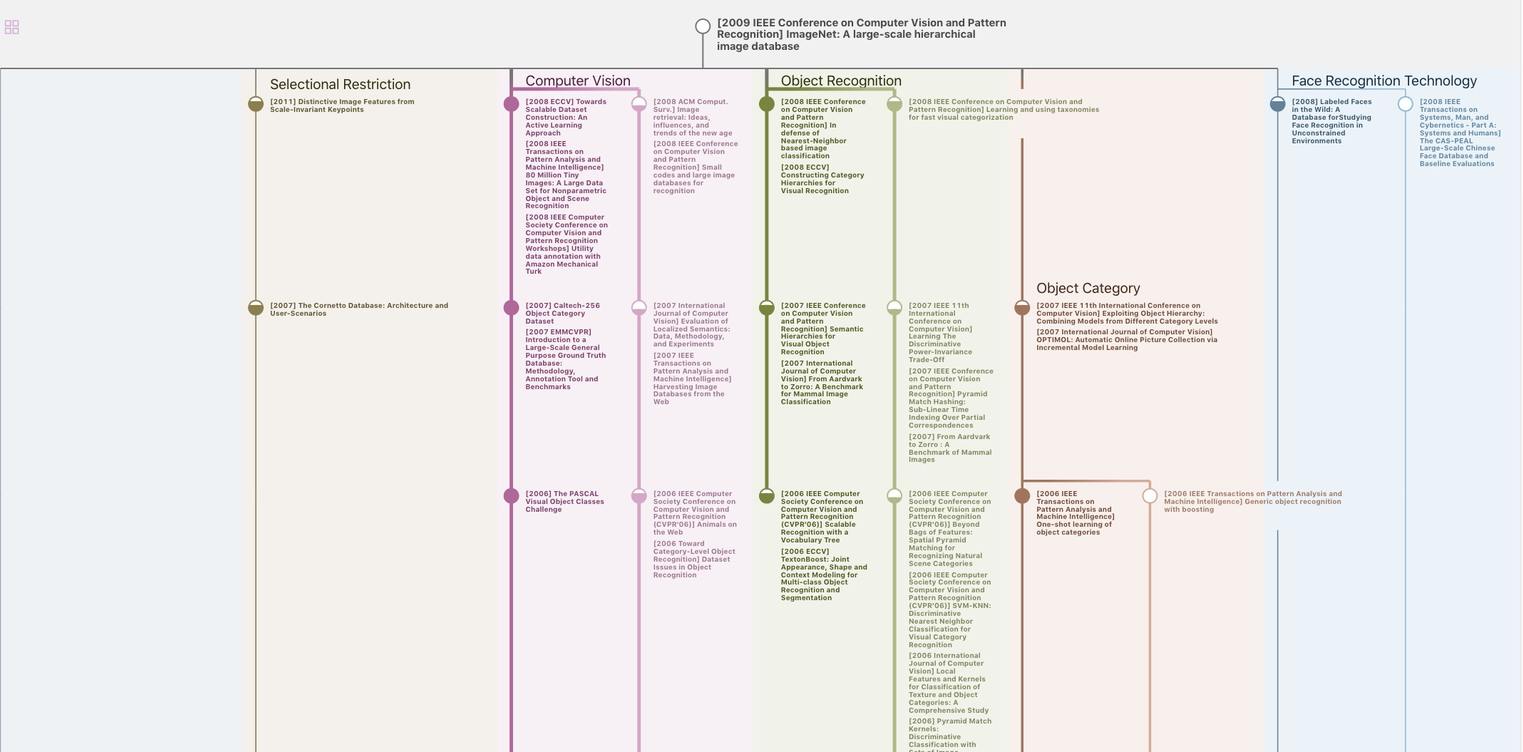
生成溯源树,研究论文发展脉络
Chat Paper
正在生成论文摘要