iMove: Exploring Bio-impedance Sensing for Fitness Activity Recognition
CoRR(2024)
摘要
Automatic and precise fitness activity recognition can be beneficial in
aspects from promoting a healthy lifestyle to personalized preventative
healthcare. While IMUs are currently the prominent fitness tracking modality,
through iMove, we show bio-impedence can help improve IMU-based fitness
tracking through sensor fusion and contrastive learning.To evaluate our
methods, we conducted an experiment including six upper body fitness activities
performed by ten subjects over five days to collect synchronized data from
bio-impedance across two wrists and IMU on the left wrist.The contrastive
learning framework uses the two modalities to train a better IMU-only
classification model, where bio-impedance is only required at the training
phase, by which the average Macro F1 score with the input of a single IMU was
improved by 3.22 % reaching 84.71 % compared to the 81.49 % of the IMU
baseline model. We have also shown how bio-impedance can improve human activity
recognition (HAR) directly through sensor fusion, reaching an average Macro F1
score of 89.57 % (two modalities required for both training and inference)
even if Bio-impedance alone has an average macro F1 score of 75.36 %, which is
outperformed by IMU alone. In addition, similar results were obtained in an
extended study on lower body fitness activity classification, demonstrating the
generalisability of our approach.Our findings underscore the potential of
sensor fusion and contrastive learning as valuable tools for advancing fitness
activity recognition, with bio-impedance playing a pivotal role in augmenting
the capabilities of IMU-based systems.
更多查看译文
关键词
bio-impedance sensing,human activity recognition,contrastive learning,sensor fusion
AI 理解论文
溯源树
样例
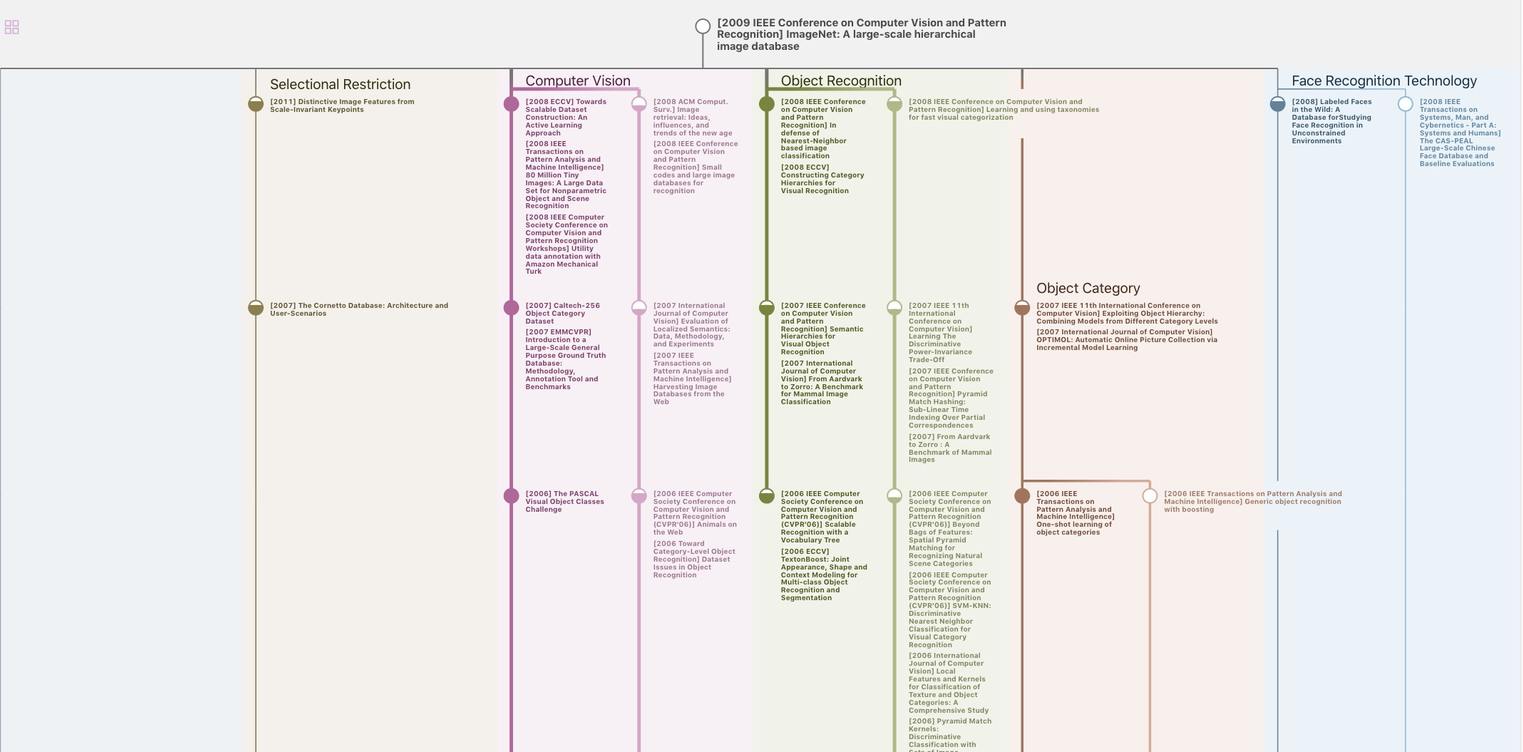
生成溯源树,研究论文发展脉络
Chat Paper
正在生成论文摘要