Crafting a Good Prompt or Providing Exemplary Dialogues? A Study of In-Context Learning for Persona-based Dialogue Generation
CoRR(2024)
摘要
Previous in-context learning (ICL) research has focused on tasks such as
classification, machine translation, text2table, etc., while studies on whether
ICL can improve human-like dialogue generation are scarce. Our work fills this
gap by systematically investigating the ICL capabilities of large language
models (LLMs) in persona-based dialogue generation, conducting extensive
experiments on high-quality real human Chinese dialogue datasets. From
experimental results, we draw three conclusions: 1) adjusting prompt
instructions is the most direct, effective, and economical way to improve
generation quality; 2) randomly retrieving demonstrations (demos) achieves the
best results, possibly due to the greater diversity and the amount of effective
information; counter-intuitively, retrieving demos with a context identical to
the query performs the worst; 3) even when we destroy the multi-turn
associations and single-turn semantics in the demos, increasing the number of
demos still improves dialogue performance, proving that LLMs can learn from
corrupted dialogue demos. Previous explanations of the ICL mechanism, such as
n-gram induction head, cannot fully account for this phenomenon.
更多查看译文
AI 理解论文
溯源树
样例
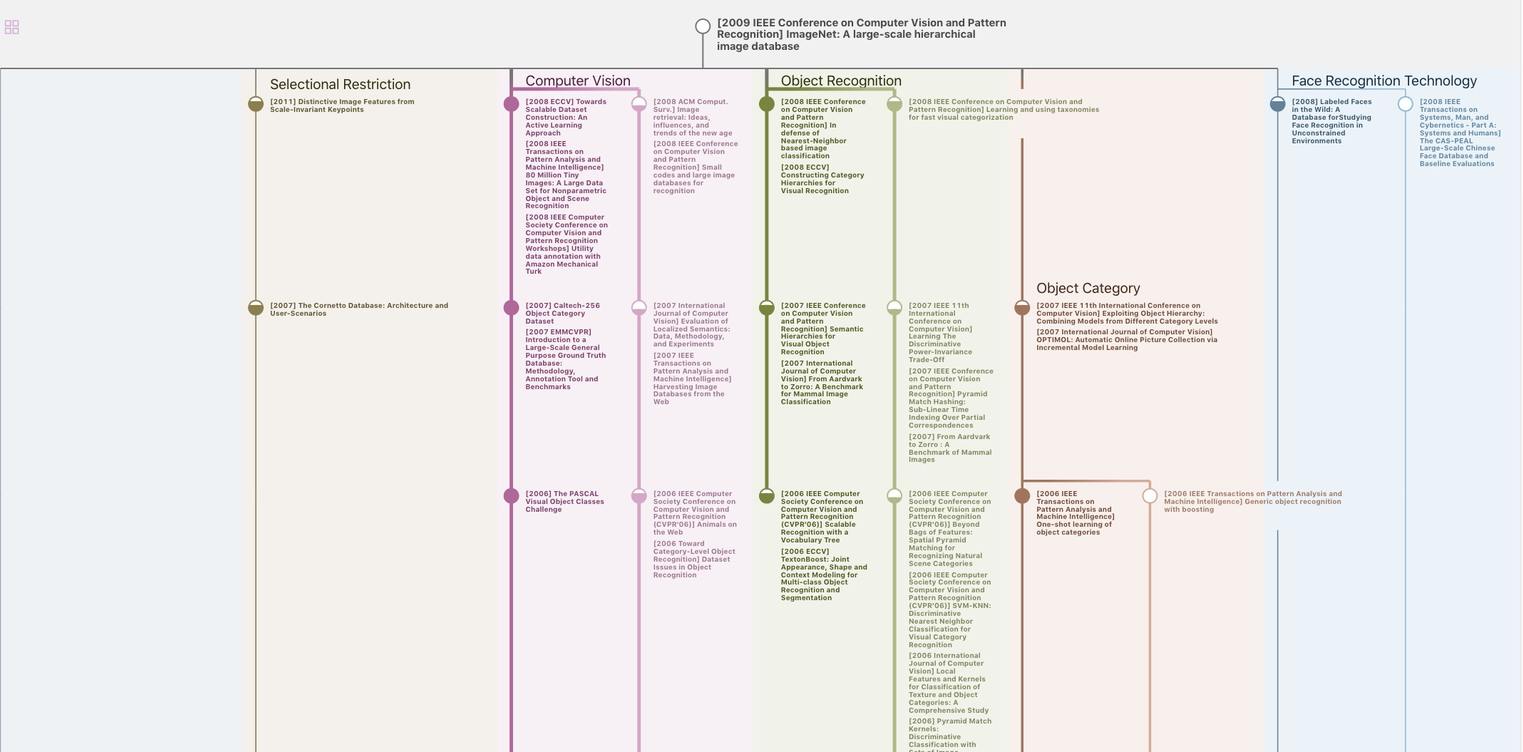
生成溯源树,研究论文发展脉络
Chat Paper
正在生成论文摘要