DE-COP: Detecting Copyrighted Content in Language Models Training Data
CoRR(2024)
摘要
How can we detect if copyrighted content was used in the training process of
a language model, considering that the training data is typically undisclosed?
We are motivated by the premise that a language model is likely to identify
verbatim excerpts from its training text. We propose DE-COP, a method to
determine whether a piece of copyrighted content was included in training.
DE-COP's core approach is to probe an LLM with multiple-choice questions, whose
options include both verbatim text and their paraphrases. We construct
BookTection, a benchmark with excerpts from 165 books published prior and
subsequent to a model's training cutoff, along with their paraphrases. Our
experiments show that DE-COP surpasses the prior best method by 9.6
detection performance (AUC) on models with logits available. Moreover, DE-COP
also achieves an average accuracy of 72
black-box models where prior methods give ≈ 4
datasets are available at https://github.com/avduarte333/DE-COP_Method
更多查看译文
AI 理解论文
溯源树
样例
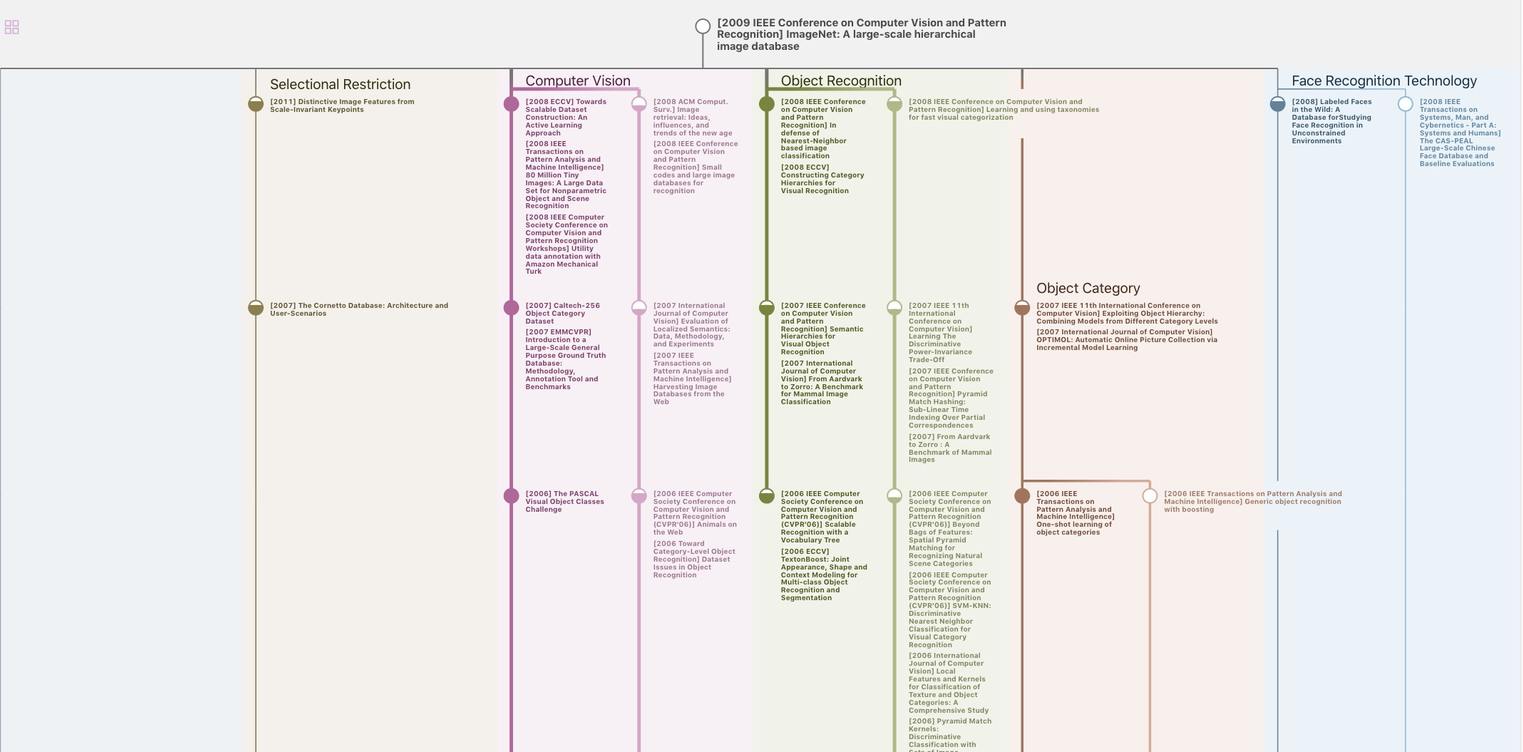
生成溯源树,研究论文发展脉络
Chat Paper
正在生成论文摘要