Revisiting Recurrent Reinforcement Learning with Memory Monoids
CoRR(2024)
摘要
In RL, memory models such as RNNs and transformers address Partially
Observable Markov Decision Processes (POMDPs) by mapping trajectories to latent
Markov states. Neither model scales particularly well to long sequences,
especially compared to an emerging class of memory models sometimes called
linear recurrent models. We discover that the recurrent update of these models
is a monoid, leading us to formally define a novel memory monoid framework. We
revisit the traditional approach to batching in recurrent RL, highlighting both
theoretical and empirical deficiencies. Leveraging the properties of memory
monoids, we propose a new batching method that improves sample efficiency,
increases the return, and simplifies the implementation of recurrent loss
functions in RL.
更多查看译文
AI 理解论文
溯源树
样例
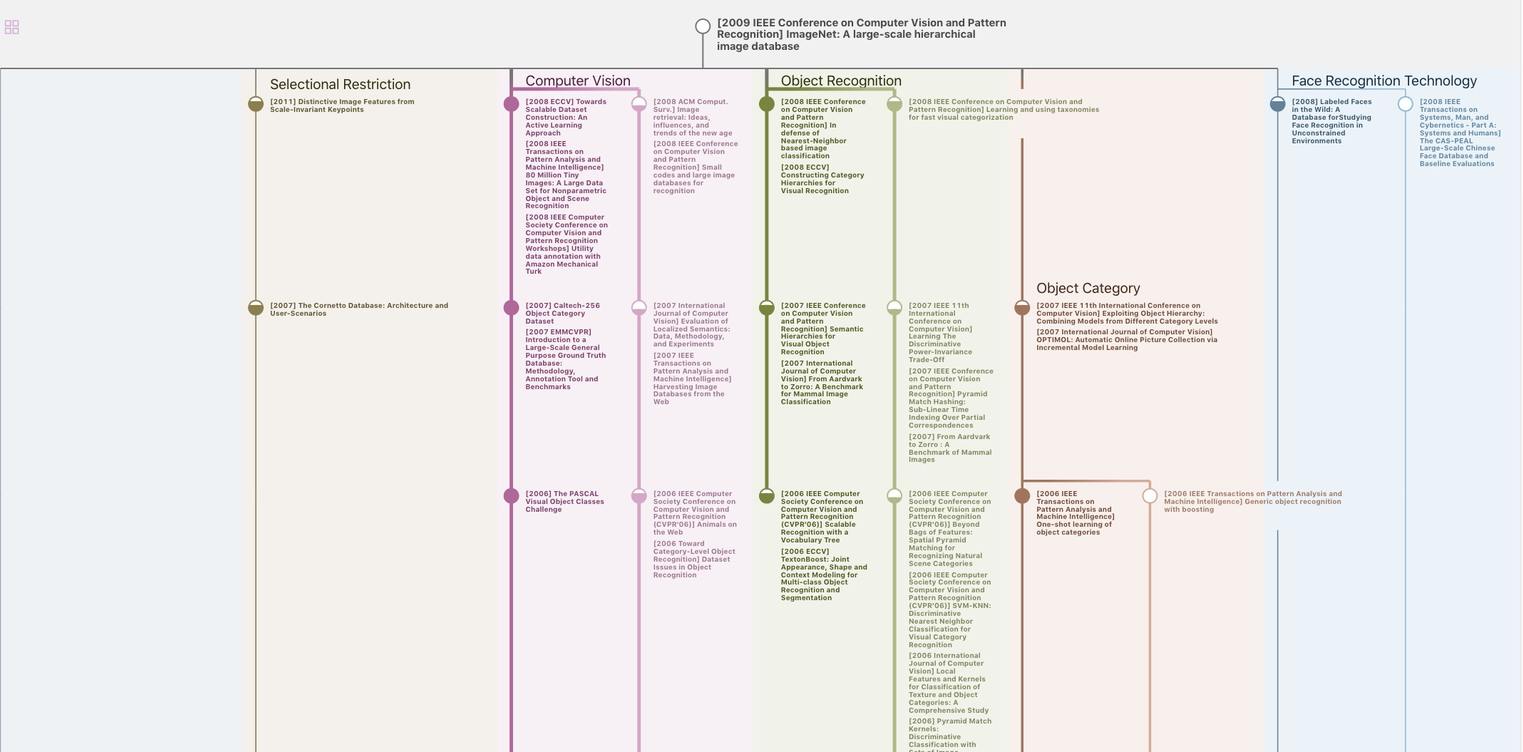
生成溯源树,研究论文发展脉络
Chat Paper
正在生成论文摘要