Reinforcement Learning for Solving Stochastic Vehicle Routing Problem with Time Windows
CoRR(2024)
摘要
This paper introduces a reinforcement learning approach to optimize the
Stochastic Vehicle Routing Problem with Time Windows (SVRP), focusing on
reducing travel costs in goods delivery. We develop a novel SVRP formulation
that accounts for uncertain travel costs and demands, alongside specific
customer time windows. An attention-based neural network trained through
reinforcement learning is employed to minimize routing costs. Our approach
addresses a gap in SVRP research, which traditionally relies on heuristic
methods, by leveraging machine learning. The model outperforms the Ant-Colony
Optimization algorithm, achieving a 1.73
uniquely integrates external information, demonstrating robustness in diverse
environments, making it a valuable benchmark for future SVRP studies and
industry application.
更多查看译文
AI 理解论文
溯源树
样例
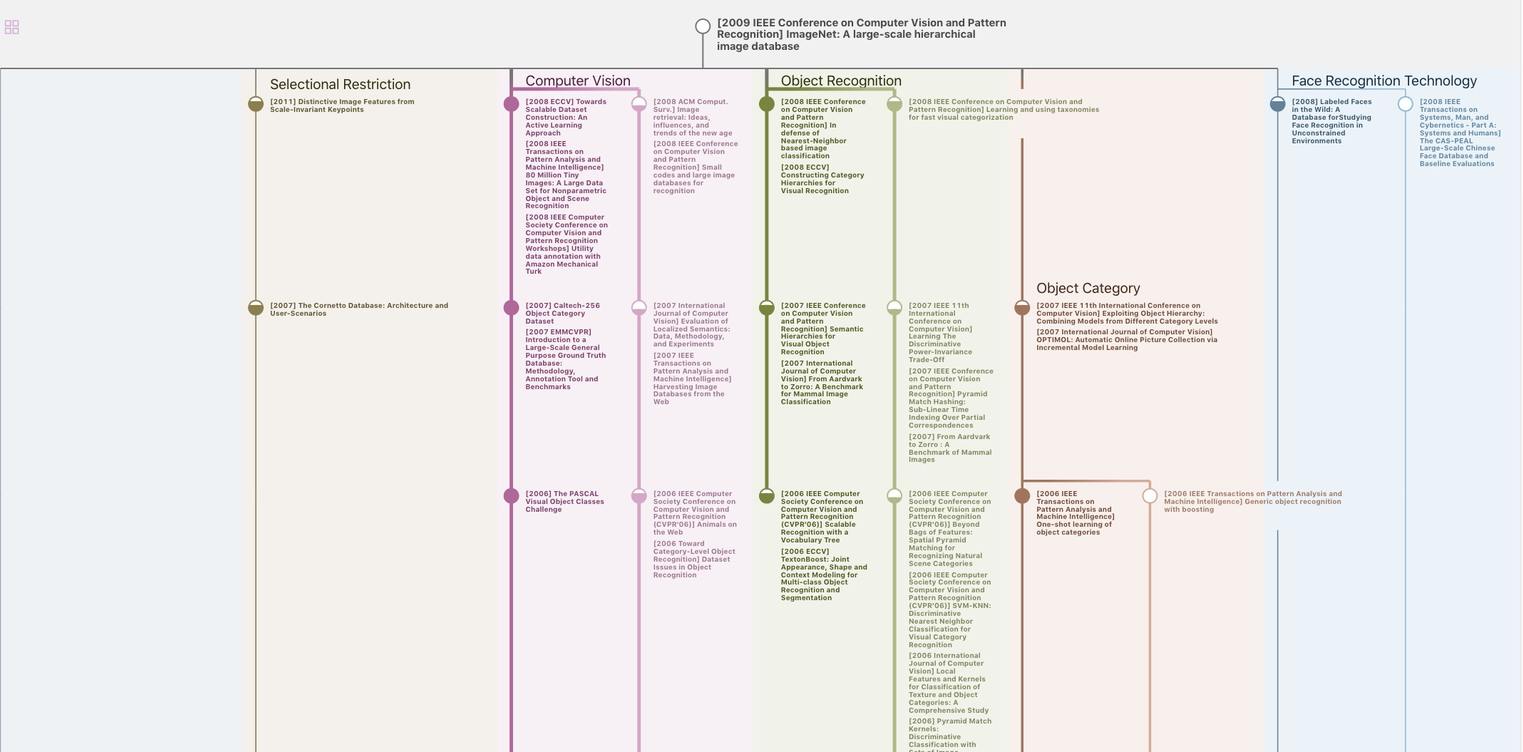
生成溯源树,研究论文发展脉络
Chat Paper
正在生成论文摘要