Optimistic Thompson Sampling for No-Regret Learning in Unknown Games
CoRR(2024)
摘要
This work tackles the complexities of multi-player scenarios in unknown
games, where the primary challenge lies in navigating the uncertainty of the
environment through bandit feedback alongside strategic decision-making. We
introduce Thompson Sampling (TS)-based algorithms that exploit the information
of opponents' actions and reward structures, leading to a substantial reduction
in experimental budgets – achieving over tenfold improvements compared to
conventional approaches. Notably, our algorithms demonstrate that, given
specific reward structures, the regret bound depends logarithmically on the
total action space, significantly alleviating the curse of multi-player.
Furthermore, we unveil the Optimism-then-NoRegret (OTN) framework, a
pioneering methodology that seamlessly incorporates our advancements with
established algorithms, showcasing its utility in practical scenarios such as
traffic routing and radar sensing in the real world.
更多查看译文
AI 理解论文
溯源树
样例
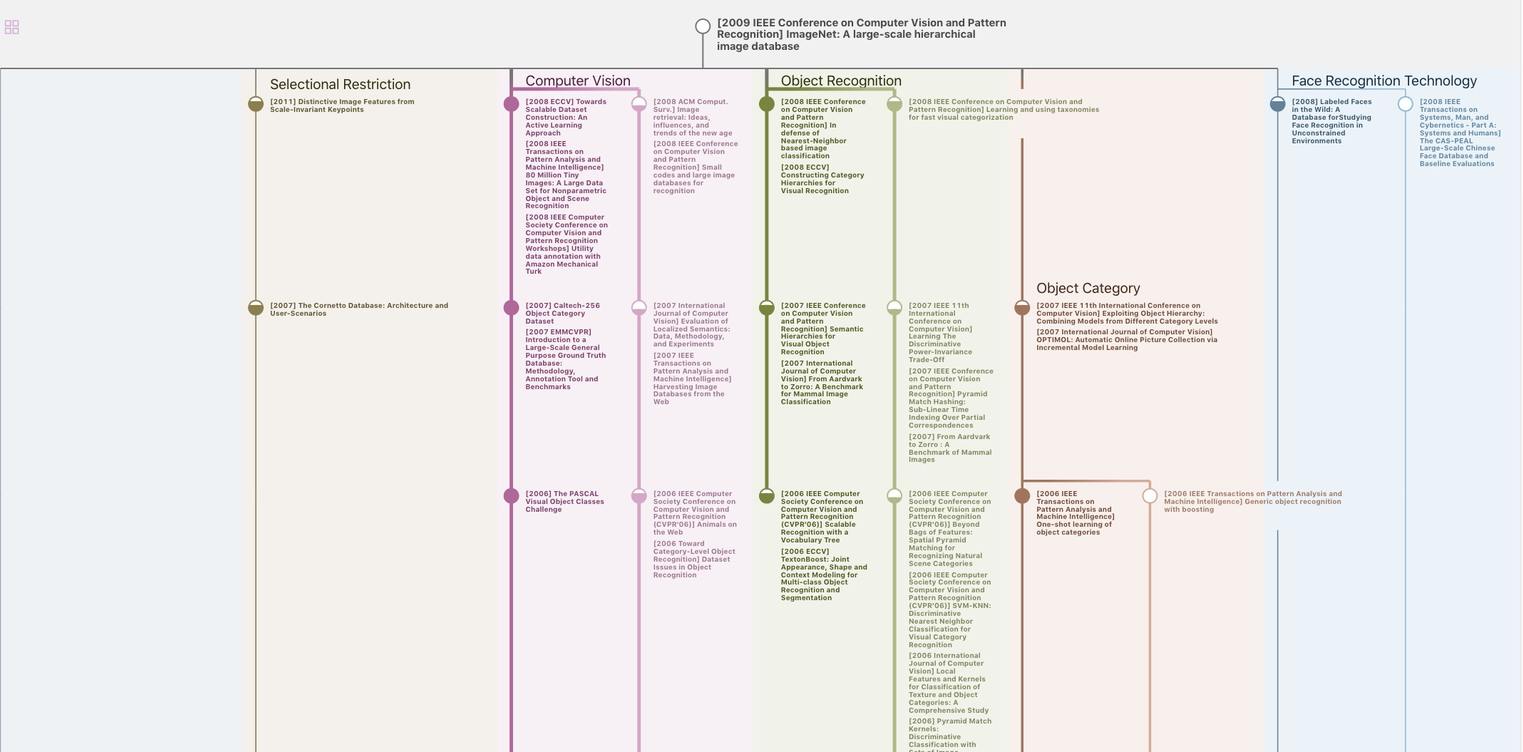
生成溯源树,研究论文发展脉络
Chat Paper
正在生成论文摘要