Fast Multiview Anchor-Graph Clustering
IEEE TRANSACTIONS ON NEURAL NETWORKS AND LEARNING SYSTEMS(2024)
摘要
Due to its high computational complexity, graph-based methods have limited applicability in large-scale multiview clustering tasks. To address this issue, many accelerated algorithms, especially anchor graph-based methods and indicator learning-based methods, have been developed and made a great success. Nevertheless, since the restrictions of the optimization strategy, these accelerated methods still need to approximate the discrete graph-cutting problem to a continuous spectral embedding problem and utilize different discretization strategies to obtain discrete sample categories. To avoid the loss of effectiveness and efficiency caused by the approximation and discretization, we establish a discrete fast multiview anchor graph clustering (FMAGC) model that first constructs an anchor graph of each view and then generates a discrete cluster indicator matrix by solving the discrete multiview graph-cutting problem directly. Since the gradient descent-based method makes it hard to solve this discrete model, we propose a fast coordinate descent-based optimization strategy with linear complexity to solve it without approximating it as a continuous one. Extensive experiments on widely used normal and large-scale multiview datasets show that FMAGC can improve clustering effectiveness and efficiency compared to other state-of-the-art baselines.
更多查看译文
关键词
Learning systems,Optimization,Clustering algorithms,Computational complexity,Tensors,Standards,Computational modeling,Anchor graph,fast clustering,large-scale data,multiview data
AI 理解论文
溯源树
样例
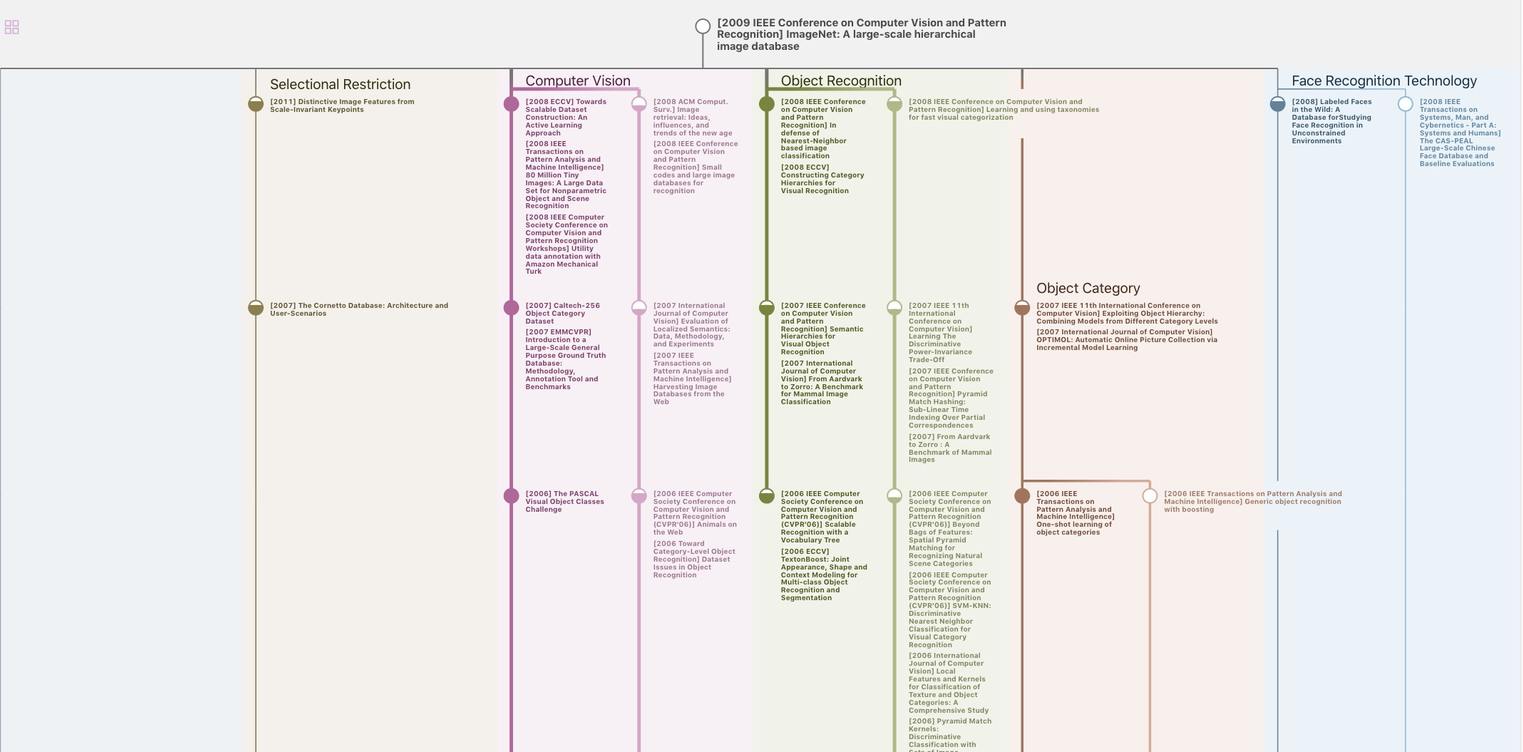
生成溯源树,研究论文发展脉络
Chat Paper
正在生成论文摘要