Positive-unlabeled learning to infer protection status and identify correlates in vaccine efficacy field trials
ISCIENCE(2024)
摘要
Correlates of protection (CoPs) are key guideposts that both support vaccine development and licensure as well as improve our understanding of the attributes of immune responses that may directly provide protection. Unfortunately, factors such as low rate of exposure and low efficacy can result in low power to discover correlates in field trials-making it difficult to identify these guideposts for the pathogens against which there is greatest need for further insights. To address this gap, we examine the ability of positive -unlabeled (PU) learning approaches to use immunogenicity data and infection status outcomes to accurately predict protection status. We report a combination of PU bagging and two-step reliable negative techniques that accurately classify the protection status of unlabeled (uninfected) samples from synthetic and real -world humoral immune response profiles in human trials and animal models and lead to the discovery of CoPs that are "missed"using conventional infection status case -control analysis.
更多查看译文
关键词
Immunology,Computational molecular modeling
AI 理解论文
溯源树
样例
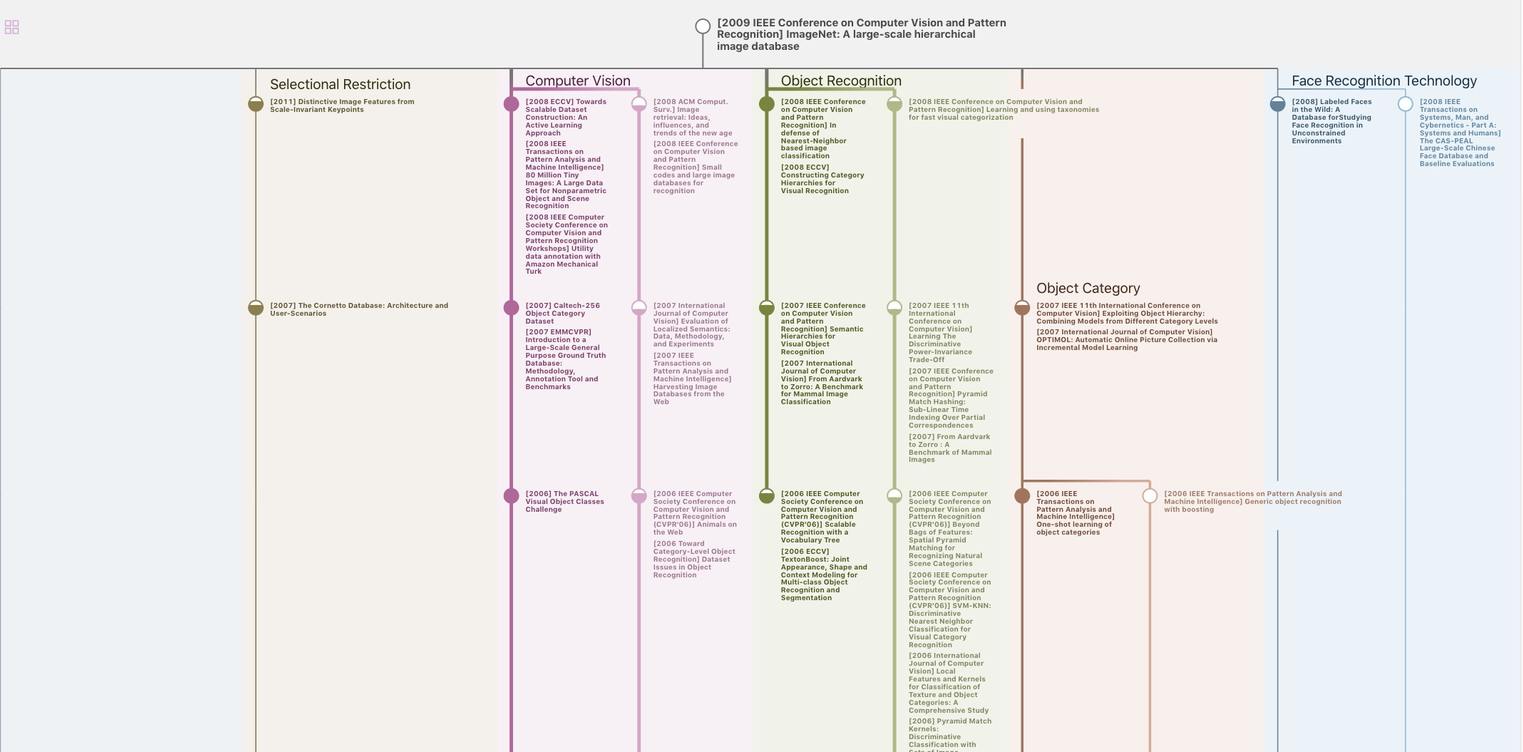
生成溯源树,研究论文发展脉络
Chat Paper
正在生成论文摘要