Adaptive Multi-Sensor Integrated Navigation System Aided by Continuous Error Map from RSU for Autonomous Vehicles in Urban Areas.
2023 IEEE 26th International Conference on Intelligent Transportation Systems (ITSC)(2023)
摘要
There has been a widespread study on multi-sensor integration to achieve precise and robust odometry for autonomous vehicles (AVs) in urban areas. LiDAR odometry and visual odometry can be affected by structureless scenarios and numerous dynamic objects. GNSS positioning can be degenerated due to the multipath and non-line-of-sight signals by buildings. Therefore, selecting appropriate weighing for heterogeneous sensors is a challenge for multi-sensor fusion. With the advancements in cellular vehicle-to-everything (C-V2X) and intelligent roadside units (RSUs), vehicles and the RSUs can collaborate to deliver reliable service. Inspired by this, this paper investigates continuous error maps for available sensors under different time conditions (noon, sunset, and night) to improve the positioning performance of surrounding AVs in complex urban environments. In particular, this paper presents an error-map-aided multi-sensor integrated system, which benefits from the error information collected by a sensor-rich AV. Then the error information is uploaded to the RSUs which is then distributed to the AVs. A smaller weight is assigned if a larger error is queried from the error map. To validate our approach, experiments were performed using the realistic CARLA simulator and our self-developed GNSS RUMS simulator. To benefit the research community, we open-sourced the implementation on our project page
3
3
https://sites.google.com!view/v2x-cooperative-navigation..
更多查看译文
关键词
Urban Areas,Autonomous Vehicles,Error Map,Roadside Units,Integrated Navigation System,Vehicles In Urban Areas,Light Detection And Ranging,Realistic Simulation,Global Navigation Satellite System,Dynamic Objects,Error Information,Visual Odometry,Area Of The Vehicle,Complex Urban Environments,Point Cloud,Unmanned Aerial Vehicles,Time Slot,Position Error,Inertial Measurement Unit,Consecutive Frames,Visual-inertial Odometry,Simultaneous Localization And Mapping,Integration Of Sensors,Weighted Least Squares,Factor Graph,Pose Estimation,World Coordinate,Different Types Of Sensors,Vision-based Methods
AI 理解论文
溯源树
样例
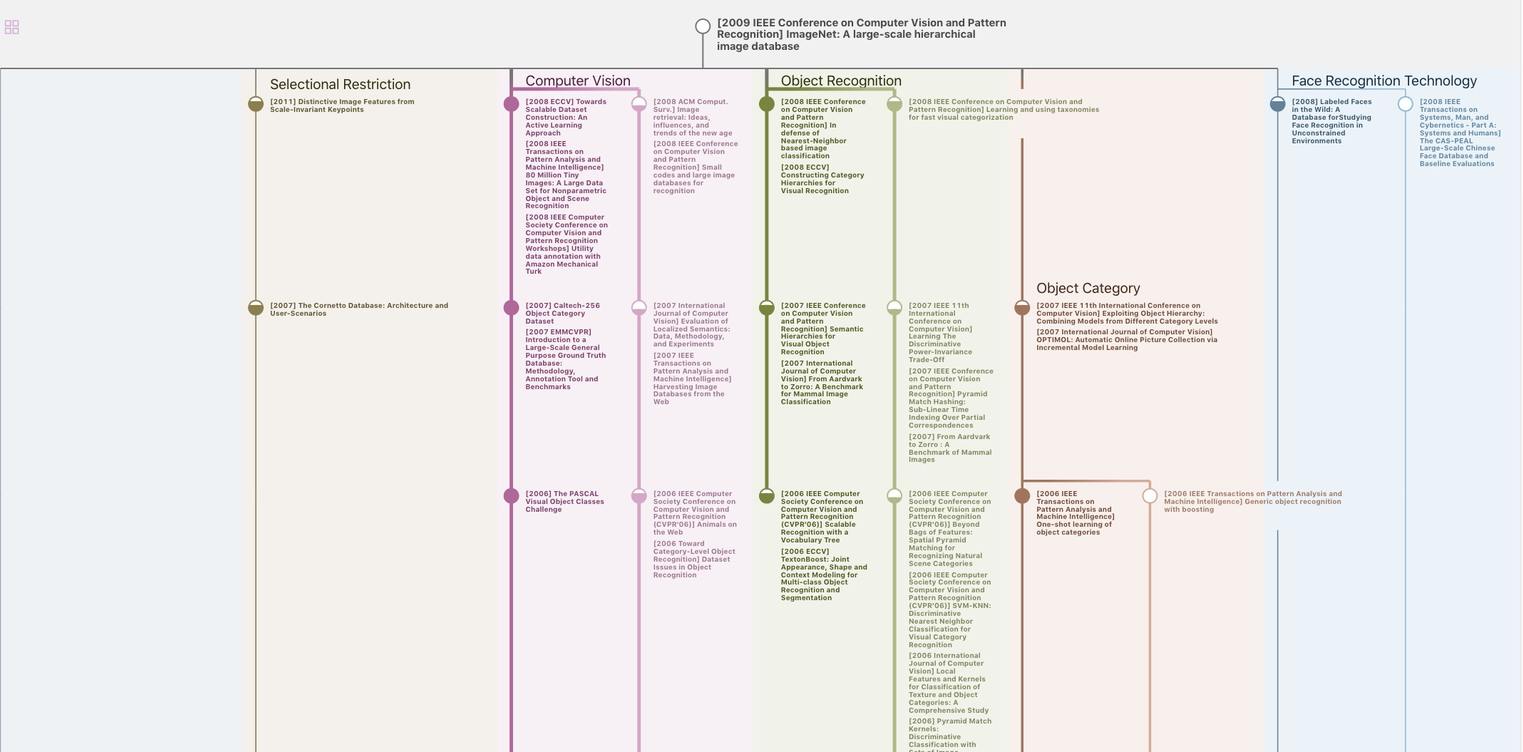
生成溯源树,研究论文发展脉络
Chat Paper
正在生成论文摘要