Personalized Causal Factor Generalization for Subjective Risky Scene Understanding with Vision Transformer.
2023 IEEE 26th International Conference on Intelligent Transportation Systems (ITSC)(2023)
摘要
In this study, we use the Vision Transformer (ViT) to pull out key features from driving video clips, aiming to understand how different participants perceive risks during driving. We then apply Counterfactual Causal Models to see how these features affect subjective driving risk among different individuals. By testing our approach with 10 participants, we found that it can personally identify which driving situations they find risky. By combining this causal analysis with the ViT's ability to understand scenes, our method showed better accuracy in detecting subjective risky situations.
更多查看译文
关键词
Scene Understanding,Vision Transformer,Risk Perception,Video Clips,Causal Model,Causal Analysis,Effective Interventions,Indirect Effect,Individual Differences,Personality Traits,Structural Equation Modeling,Risk Level,Natural Language,Contextual Information,Environmental Characteristics,Causal Inference,Levels In Subjects,Subjective Perception,Transformer Model,Traffic Light,Left Lane,Level Of Risk Perception,Road Safety,Attention Map,Intelligent Transportation Systems,Natural Language Descriptions,Lane Change,Subjective Factors,Risk Score,Extract Feature Information
AI 理解论文
溯源树
样例
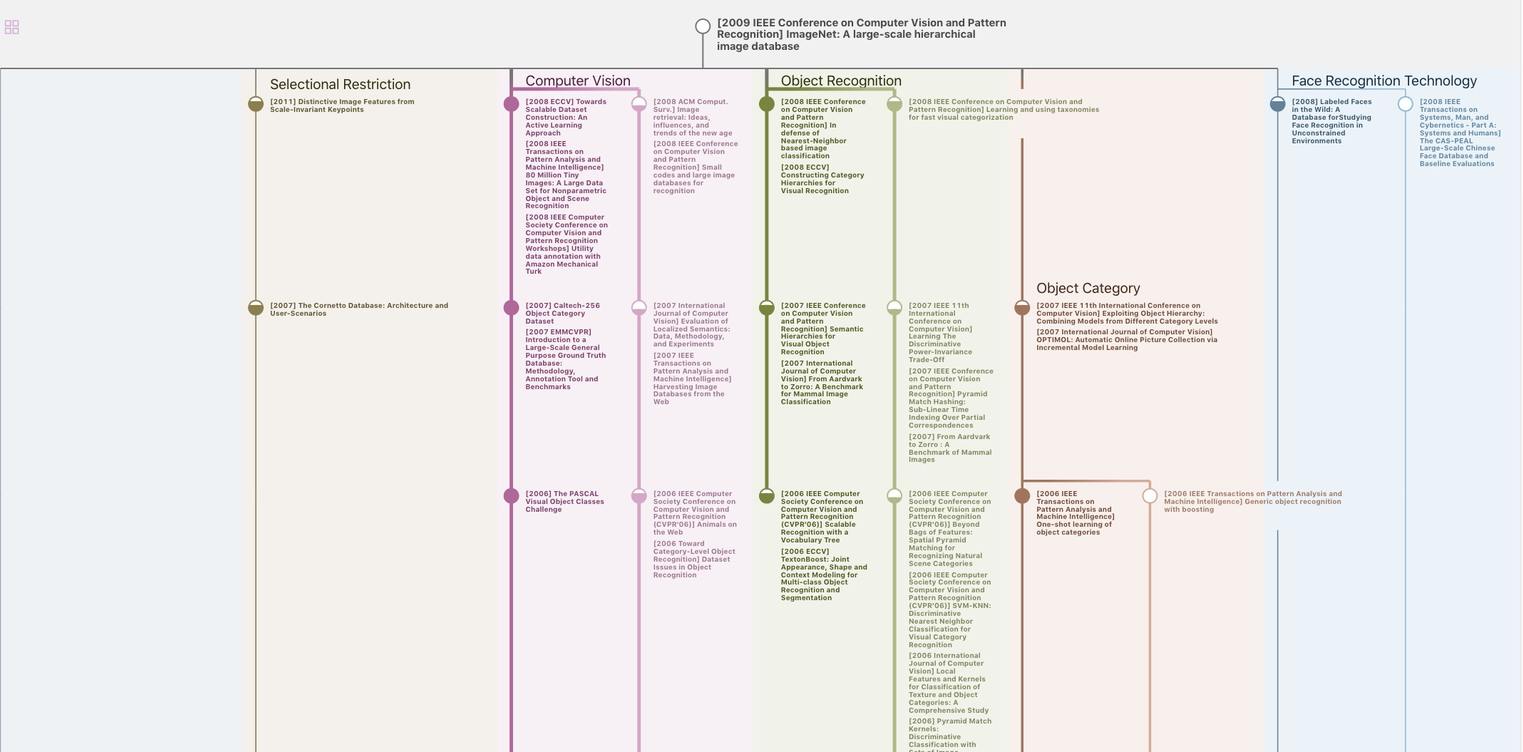
生成溯源树,研究论文发展脉络
Chat Paper
正在生成论文摘要