Leveraging cough sounds to optimize chest x-ray usage in low-resource settings
CoRR(2024)
摘要
Chest X-ray is a commonly used tool during triage, diagnosis and management
of respiratory diseases. In resource-constricted settings, optimizing this
resource can lead to valuable cost savings for the health care system and the
patients as well as to and improvement in consult time. We used
prospectively-collected data from 137 patients referred for chest X-ray at the
Christian Medical Center and Hospital (CMCH) in Purnia, Bihar, India. Each
patient provided at least five coughs while awaiting radiography. Collected
cough sounds were analyzed using acoustic AI methods. Cross-validation was done
on temporal and spectral features on the cough sounds of each patient. Features
were summarized using standard statistical approaches. Three models were
developed, tested and compared in their capacity to predict an abnormal result
in the chest X-ray. All three methods yielded models that could discriminate to
some extent between normal and abnormal with the logistic regression performing
best with an area under the receiver operating characteristic curves ranging
from 0.7 to 0.78. Despite limitations and its relatively small sample size,
this study shows that AI-enabled algorithms can use cough sounds to predict
which individuals presenting for chest radiographic examination will have a
normal or abnormal results. These results call for expanding this research
given the potential optimization of limited health care resources in low- and
middle-income countries.
更多查看译文
AI 理解论文
溯源树
样例
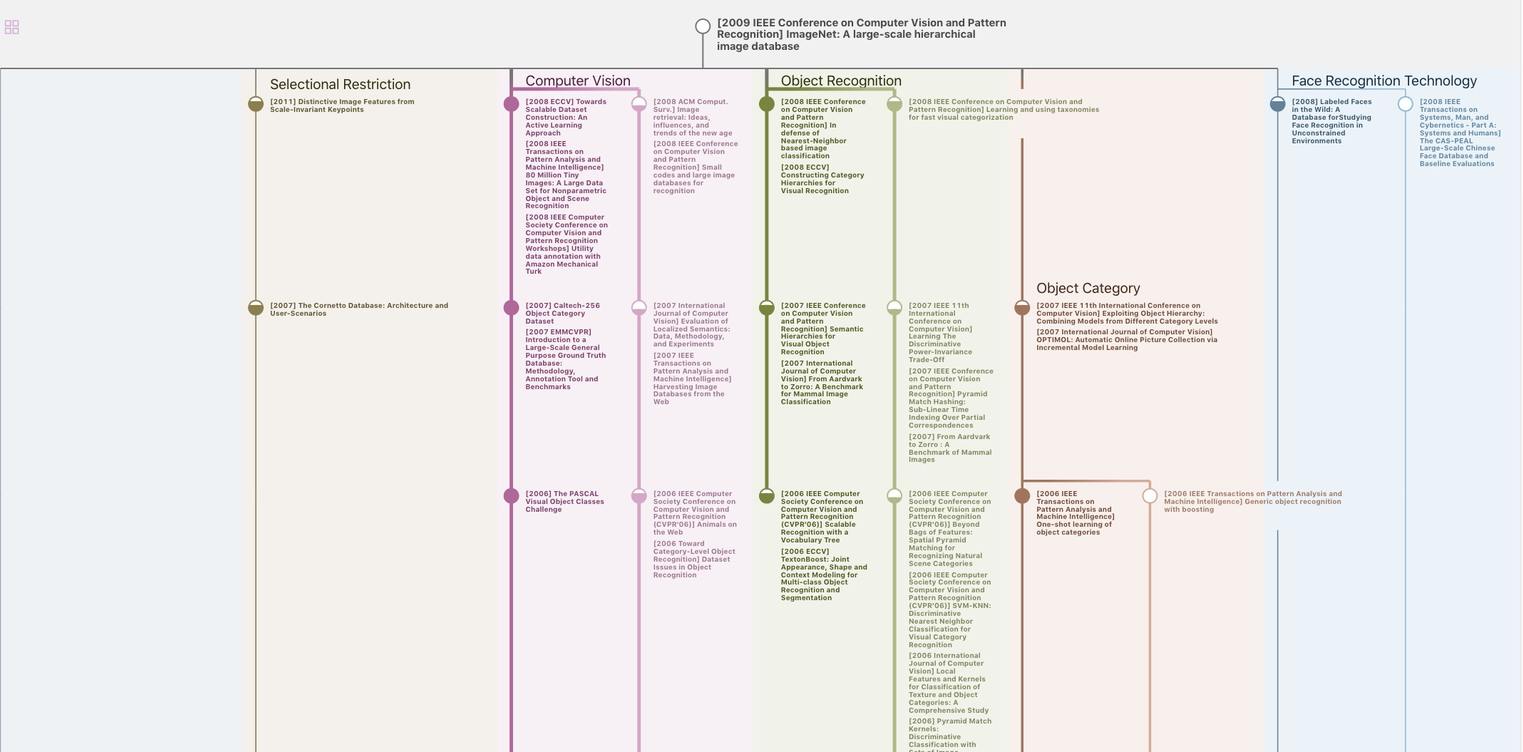
生成溯源树,研究论文发展脉络
Chat Paper
正在生成论文摘要