Driving Pattern Classification for Wheel Loaders in Different Material Handling Using Machine Learning.
2023 IEEE 26th International Conference on Intelligent Transportation Systems (ITSC)(2023)
摘要
The present paper discusses a new method of classifying kinds of material and driving stages and styles of Volvo wheel loaders (WLO). This is achieved by indirectly monitoring relevant, but usually latent variables, based on directly monitored sensors of WLOs. The continuous classifications will support Volvo's actual objectives such as, e.g., maximizing the remaining useful life of components, fuel efficiency, and productivity. To this end, a set of WLO machines was equipped with extra sensors and collected a limited dataset with richer information. Based on this limited dataset, different machine learning (ML) methods were tested to derive and to verify the classifications. It showed that support vector machines (SVM) produced the best results: the driving styles could be classified with a test accuracy of 77% (resp, 99.5%) in the loading (resp. unloading) driving stage. Further the SVM model is also verified both theoretically to enhance the confidence in the model and experimentally with a set of additional test drivers.
更多查看译文
关键词
Machine Learning,Wheel Loader,Support Vector Machine,Latent Variables,Machine Learning Methods,Kind Of Material,Support Vector Machine Model,Actual Object,Life Of Components,Classification Model,Test Data,Artificial Neural Network,Type Material,Machine Learning Models,Machine Learning Approaches,Sensor Data,Recurrent Neural Network,Short Cycle,State Machine,Loading And Unloading,Loading Stage,Different Kinds Of Materials,Hydraulic Pressure,Driver Behavior,Young Drivers,Key Sensor,User Testing,Cycle Count,Induction Motor,Accuracy Of Model
AI 理解论文
溯源树
样例
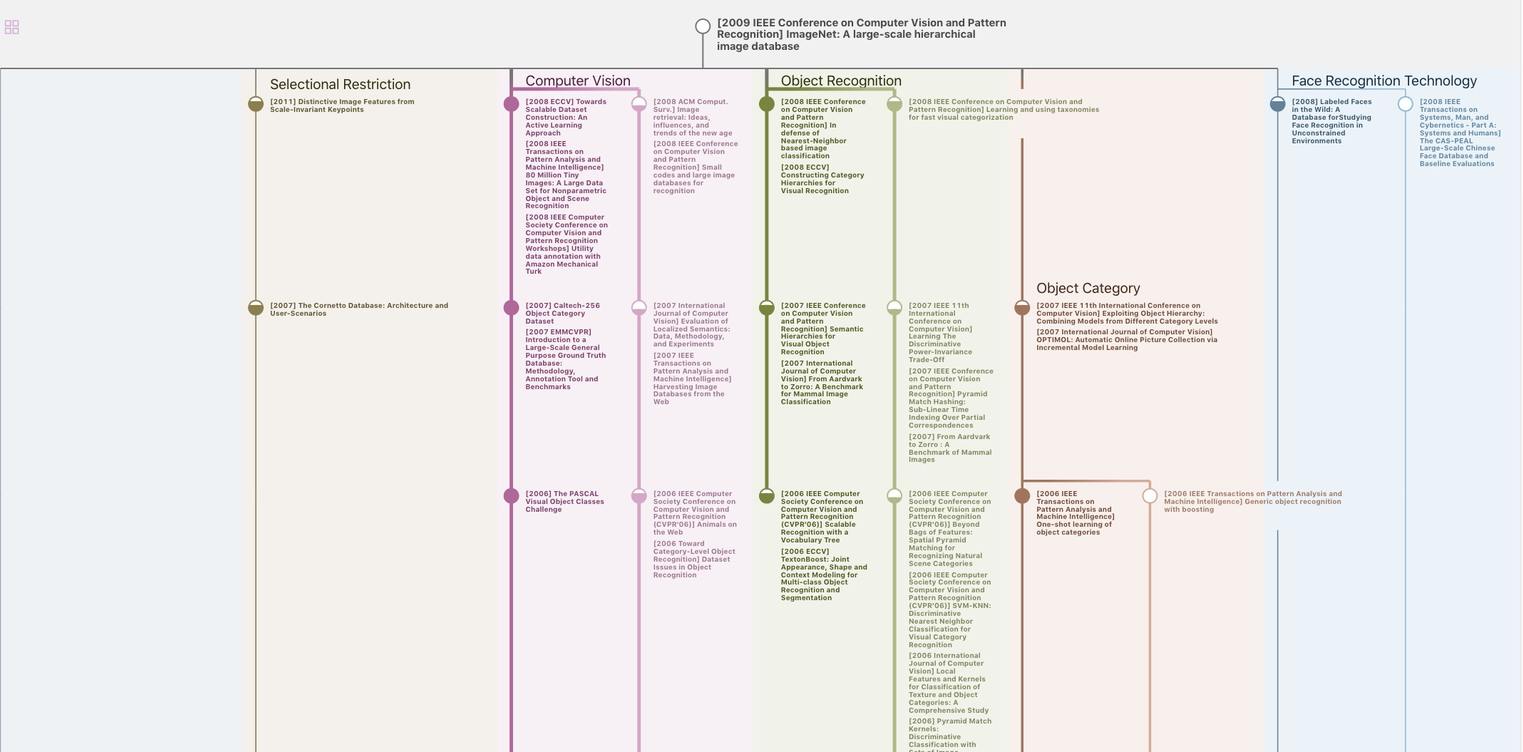
生成溯源树,研究论文发展脉络
Chat Paper
正在生成论文摘要