Decision Making for Driving Agent in Traffic Simulation via Adversarial Inverse Reinforcement Learning.
2023 IEEE 26th International Conference on Intelligent Transportation Systems (ITSC)(2023)
摘要
Traffic simulation has the potential to facilitate the development and testing of autonomous vehicles, as a supplement to road testing. Since autonomous vehicles will coexist with human drivers in the transportation system for a period of time, it is important to have intelligent driving agents in traffic simulation to interact with them just like human drivers. Directly learning from human drivers' driving behavior is an attractive solution with potential. In this study, Adversarial Inverse Reinforcement Learning (AIRL) is applied to learn decision-making policies in complex and interactive traffic simulation environments with high traffic density. Bird's Eye View (BEV) is proposed as an observation model for driving agents, providing effective information for the agents' decision-making. Results show that compared with Behavioral Cloning (BC) and Proximal Policy Optimization (PPO), the driving agents generated by AIRL demonstrate higher levels of safety and robustness and they are capable of imitating the car-following and lane-changing characteristics from expert demonstrations. The results further confirm that different driving characteristics can be learned based on AIRL method.
更多查看译文
关键词
Generative Adversarial Networks,Traffic Simulation,Inverse Reinforcement Learning,Adversarial Inverse Reinforcement Learning,Autonomous Vehicles,Bird’s Eye,Human Drivers,Traffic Environment,Proximal Policy Optimization,High Traffic Density,Expert Demonstrations,Neural Network,Value Function,Optimization Algorithm,Related Information,Human Experts,Reward Function,Observation Space,Training Framework,Lane Change,Traffic Scenarios,Hellinger Distance,One-dimensional Vector,Imitation Learning,Capabilities Of Agents,State-action Pair,Top-down Perspective,Trained Agent,Interaction Scenarios,Position Index
AI 理解论文
溯源树
样例
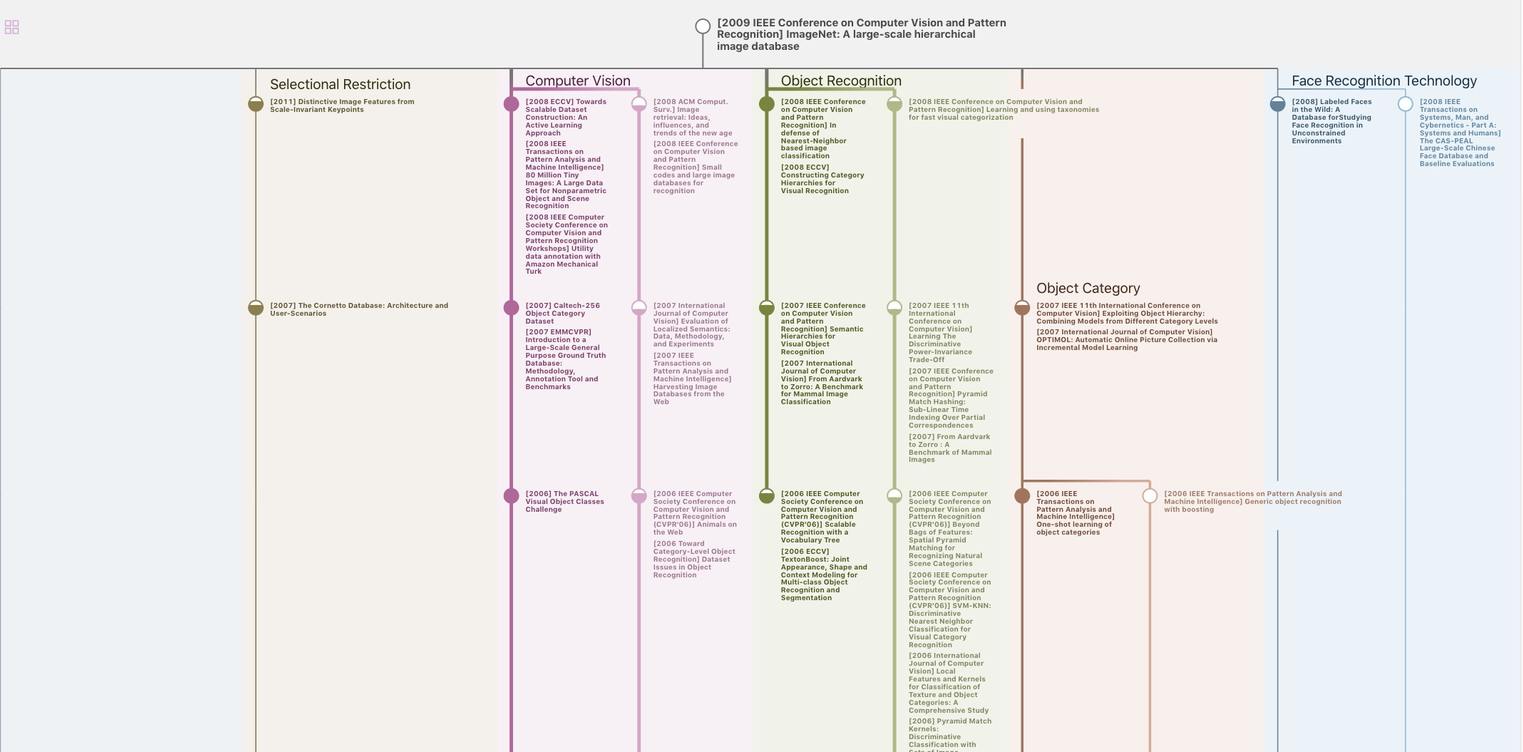
生成溯源树,研究论文发展脉络
Chat Paper
正在生成论文摘要