Hypergraph Transformer for Knowledge Tracing.
Computing and Communication Workshop and Conference(2024)
摘要
The intelligent education can provide students with suitable exercises based on their knowledge state, greatly improving their learning efficiency. In the past decade, many advanced knowledge tracking models have been proposed to estimate the level of knowledge of students for different concepts. These models fail to effectively capture the interrelations between learning interactions and struggle to incorporate historical contextual information when making predictions. In this paper, we propose a knowledge tracking model based on Hypergraph Transformer: Hypergraph Transformer Network for Knowledge Tracing (HTNKT). The key advantage of HTNKT is its capability to capture the relationships between interactions using Hypergraph Convolution. Additionally, it incorporates an improved transformer module to effectively capture timing information. This combination enables HTNKT to provide more accurate knowledge tracing. The ASSIST and EdNet dataset experiments show that the HTNKT performs better than the state-of-the-art baselines.
更多查看译文
关键词
Intelligent Education,Knowledge Tracing,Deep Learning,Transformer,hypergraph
AI 理解论文
溯源树
样例
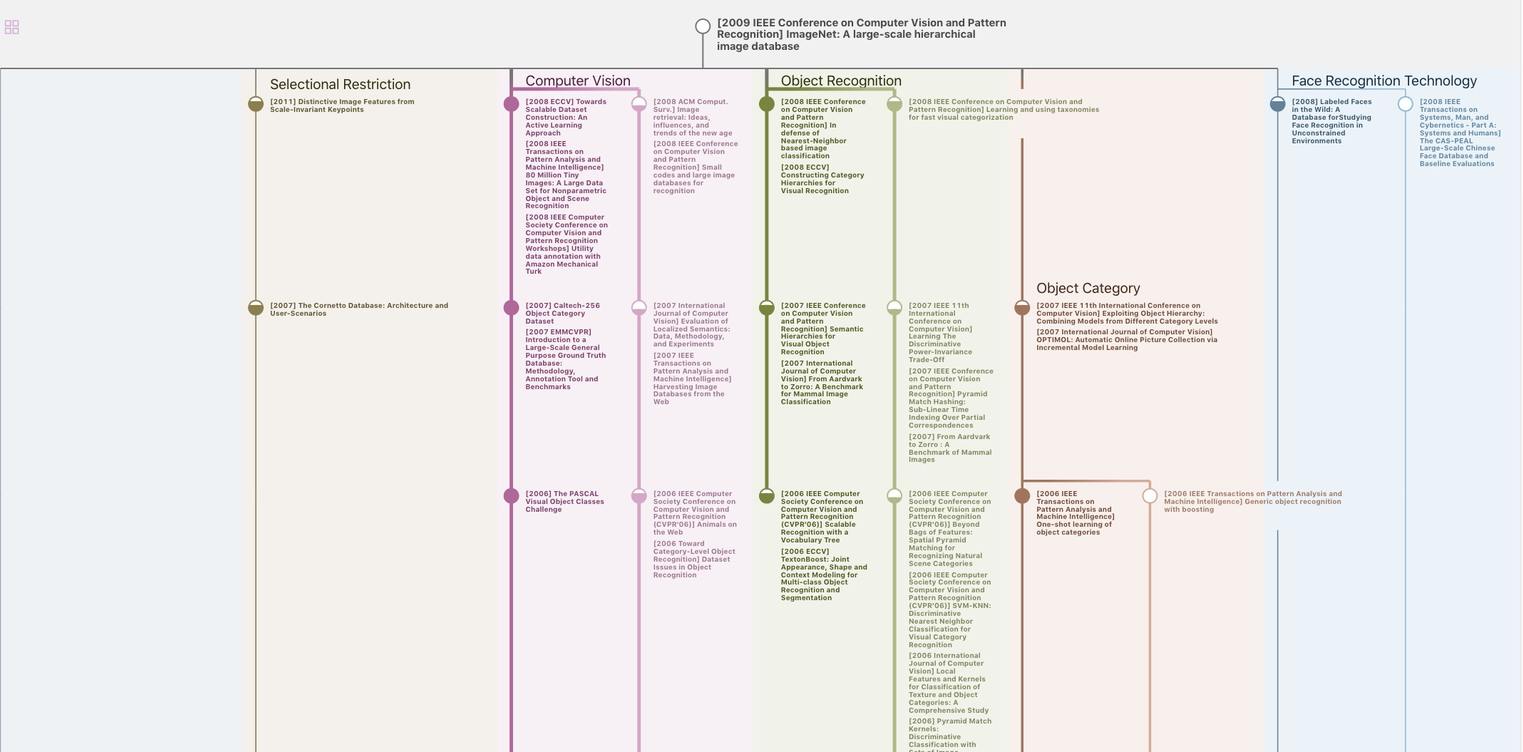
生成溯源树,研究论文发展脉络
Chat Paper
正在生成论文摘要