GNSS Feature Map Aided RTK Positioning in Urban Trenches.
2023 IEEE 26th International Conference on Intelligent Transportation Systems (ITSC)(2023)
摘要
Accurate localization in urban environments plays a crucial role in many applications and emerging technologies, such as autonomous driving or pedestrian navigation. The demand for high accuracy and high integrity positioning models using the Global Navigation Satellite System (GNSS) sensor is increasing due to the fact that the GNSS sensor is the only one providing absolute positioning information. The main error source for GNSS-based positioning in urban environments is multipath and non-line-of-sight (NLOS) signal reception caused by high-raised buildings in the vicinity of the antenna, leading to ranging errors which can reach up to several hundreds of meters. In kinematic urban GNSS applications, the main error sources have a complex spatio-temporal behaviour. Hence, many studies focus on multipath mitigation methods based on ray tracing algorithms in conjunction with 3D city models. Performing epoch-wise ray tracing to exclude NLOS satellites is computationally intensive and requires special computation techniques for real-time applicability. In this contribution, we generate a fully populated GNSS Feature Map based on collected real GNSS data containing information on potential pseudorange errors. This map is integrated into a GNSS real-time kinematic (RTK) positioning algorithm to adapt the weighting model and consequently improving the positioning solution without the need of high computation load and external city model information. By applying our proposed approach, root mean squared errors of horizontal position deviations are improved by 90.5 % and the maximum horizontal position error is reduced from 2.45 m to 0.52 m. The increased accuracy of the positioning solution also leads to more nominal operation modes (95.8 % compared to 77.4 %) and misleading information and hazardous operations epochs are minimized.
更多查看译文
关键词
Global Navigation Satellite System,Real-time Kinematic,Root Mean Square Error,Mean Square Error,Misinformation,Urban Environments,Localization Accuracy,Need For Information,Horizontal Position,External Information,Signal Reception,Load Model,Ray Tracing,City Model,Main Source Of Error,Horizontal Error,Horizontal Deviation,Accurate Demand,Role In Many Applications,Urban Applications,Extended Kalman Filter,Longitudinal Direction,Static Phase,Covariance Matrix,Inertial Measurement Unit,Lateral Direction,Satellite Position,Update Time,Carrier Phase,Reference Trajectory
AI 理解论文
溯源树
样例
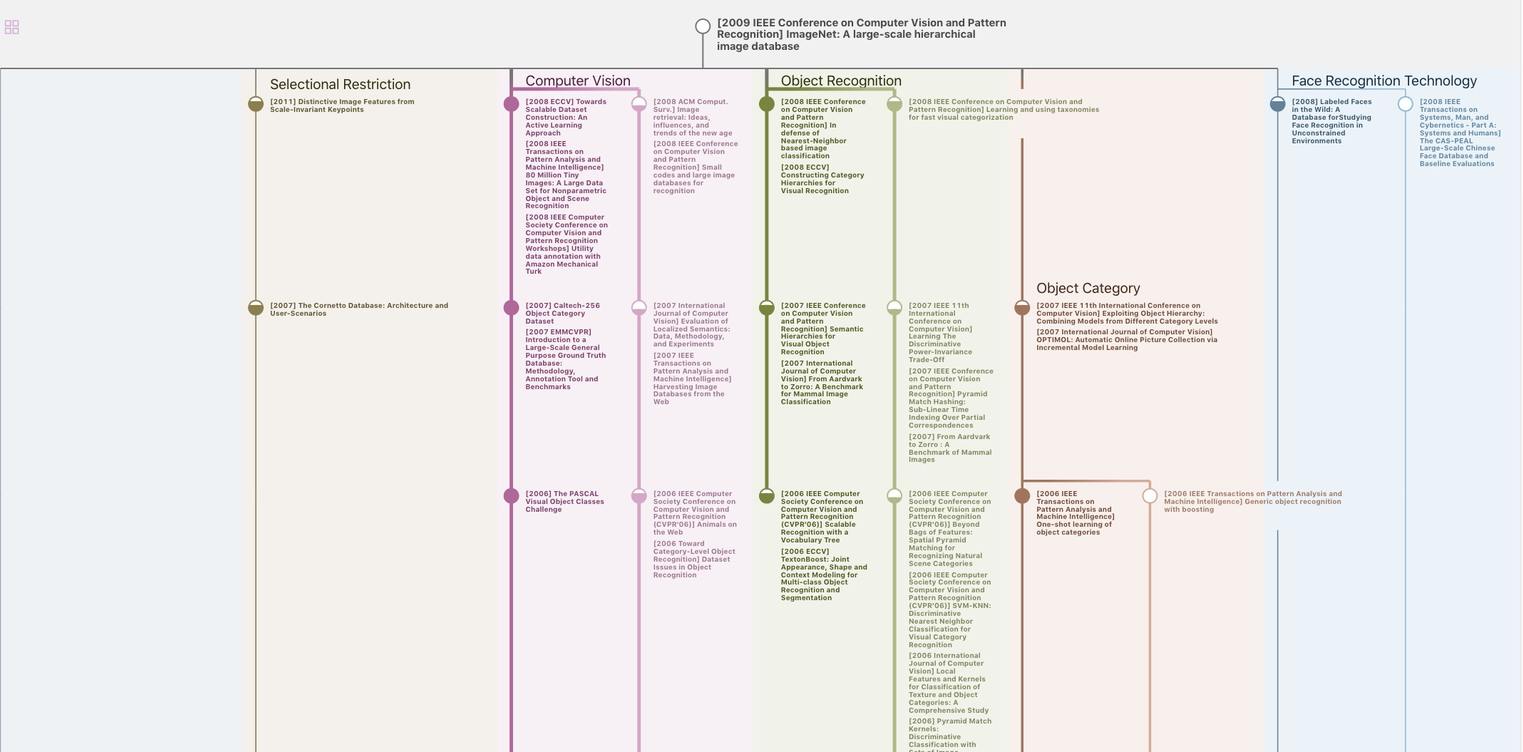
生成溯源树,研究论文发展脉络
Chat Paper
正在生成论文摘要