Joint learning of multi-level dynamic brain networks for autism spectrum disorder diagnosis
COMPUTERS IN BIOLOGY AND MEDICINE(2024)
摘要
Graph convolutional networks (GCNs), with their powerful ability to model non-Euclidean graph data, have shown advantages in learning representations of brain networks. However, considering the complexity, multilayeredness, and spatio-temporal dynamics of brain activities, we have identified two limitations in current GCNbased research on brain networks: 1) Most studies have focused on unidirectional information transmission across brain network levels, neglecting joint learning or bidirectional information exchange among networks. 2) Most of the existing models determine node neighborhoods by thresholding or simply binarizing the brain network, which leads to the loss of edge weight information and weakens the model's sensitivity to important information in the brain network. To address the above issues, we propose a multi -level dynamic brain network joint learning architecture based on GCN for autism spectrum disorder (ASD) diagnosis. Specifically, firstly, constructing different -level dynamic brain networks. Then, utilizing joint learning based on GCN for interactive information exchange among these multi -level brain networks. Finally, designing an edge self -attention mechanism to assign different edge weights to inter -node connections, which allows us to pick out the crucial features for ASD diagnosis. Our proposed method achieves an accuracy of 81.5 %. The results demonstrate that our method enables bidirectional transfer of high -order and low -order information, facilitating information complementarity between different levels of brain networks. Additionally, the use of edge weights enhances the representation capability of ASD-related features.
更多查看译文
关键词
Dynamic brain network,Joint learning,Edge self-attention mechanism,Autism spectrum disorder,Graph convolutional network
AI 理解论文
溯源树
样例
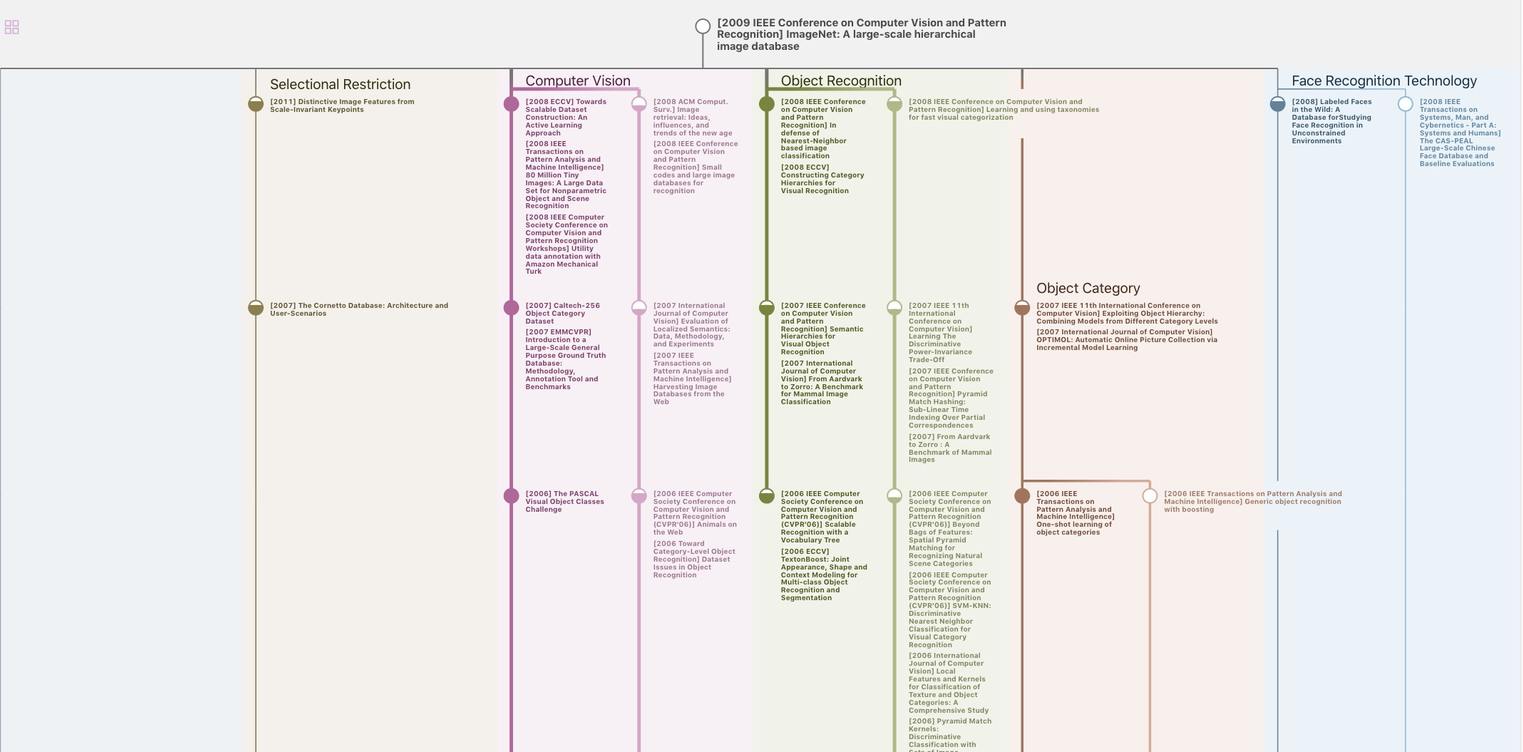
生成溯源树,研究论文发展脉络
Chat Paper
正在生成论文摘要