AirFusion: A Machine Learning Framework for Balancing Air Traffic Demand and Airspace Capacity Through Dynamic Airspace Sectorization.
2023 IEEE 26th International Conference on Intelligent Transportation Systems (ITSC)(2023)
摘要
Balancing air traffic demand and airspace capacity is a key challenge in airspace management. This task requires situational awareness among air traffic controllers, necessitating the use of interpretable traffic forecasts and visual tools to facilitate well-informed decision-making processes. This paper proposed AirFusion, a machine learning framework designed to balance airspace demand and capacity through Dynamic Airspace Sectorization (DAS). DAS is a concept that involves the dynamic change of the sectors configuration in response to fluctuations in traffic demand. The proposed framework includes four key components: (i) demand and capacity prediction, leveraging the Temporal Fusion Transformer (TFT) - a high-performing multi-horizon prediction model that offers interpretable insights into temporal dynamics, enabling traffic demand and airspace sector capacity prediction with a 4-hour look ahead and 6-hour look back window; (ii) major flow identification, using the Density- Based Spatial Clustering of Applications with Noise (DBSCAN) algorithm to effectively learn traffic patterns and identify major traffic flows; (iii) DAS, optimizing airspace sector capacity by employing graph-based partitioning method to split the sector when predicted demand exceeds capacity; and (iv) visual interface, providing an interactive platform that presents the sector splitting boundary and key influencers for demand and capacity predictions, enabling well-informed timely DAS for air traffic controllers. To validate the proposed AirFusion framework, air traffic data from four selected sectors of Singapore Flight Information Region (FIR) in December 2019 is used for training and evaluation. The experimental results demonstrate the model's high accuracy, with a mean absolute error of 0.0234 for traffic demand prediction and 0.0291 for airspace sector capacity prediction. Furthermore, the R-squared values indicate high predictive performance, with an average of 0.9133 for traffic demand and 0.9605 for airspace sector capacity.
更多查看译文
关键词
Demand Capacity,Traffic Demand,Airspace Capacity,Air Traffic Demand,December 2019,Mean Absolute Error,Traffic Flow,R-squared Values,Situational Awareness,Key Influence,Air Control,Traffic Patterns,Visual Interface,Traffic Prediction,Demand Prediction,Time Step,Longitude,Long Short-term Memory,Attention Mechanism,Grid Cells,Flight Trajectory,Hausdorff Distance,Demand Sectors,Aircraft Type,Squared Euclidean Distance,Attention Heads,30-minute Intervals,Mean Absolute Error Values,Future Input,Past Inputs
AI 理解论文
溯源树
样例
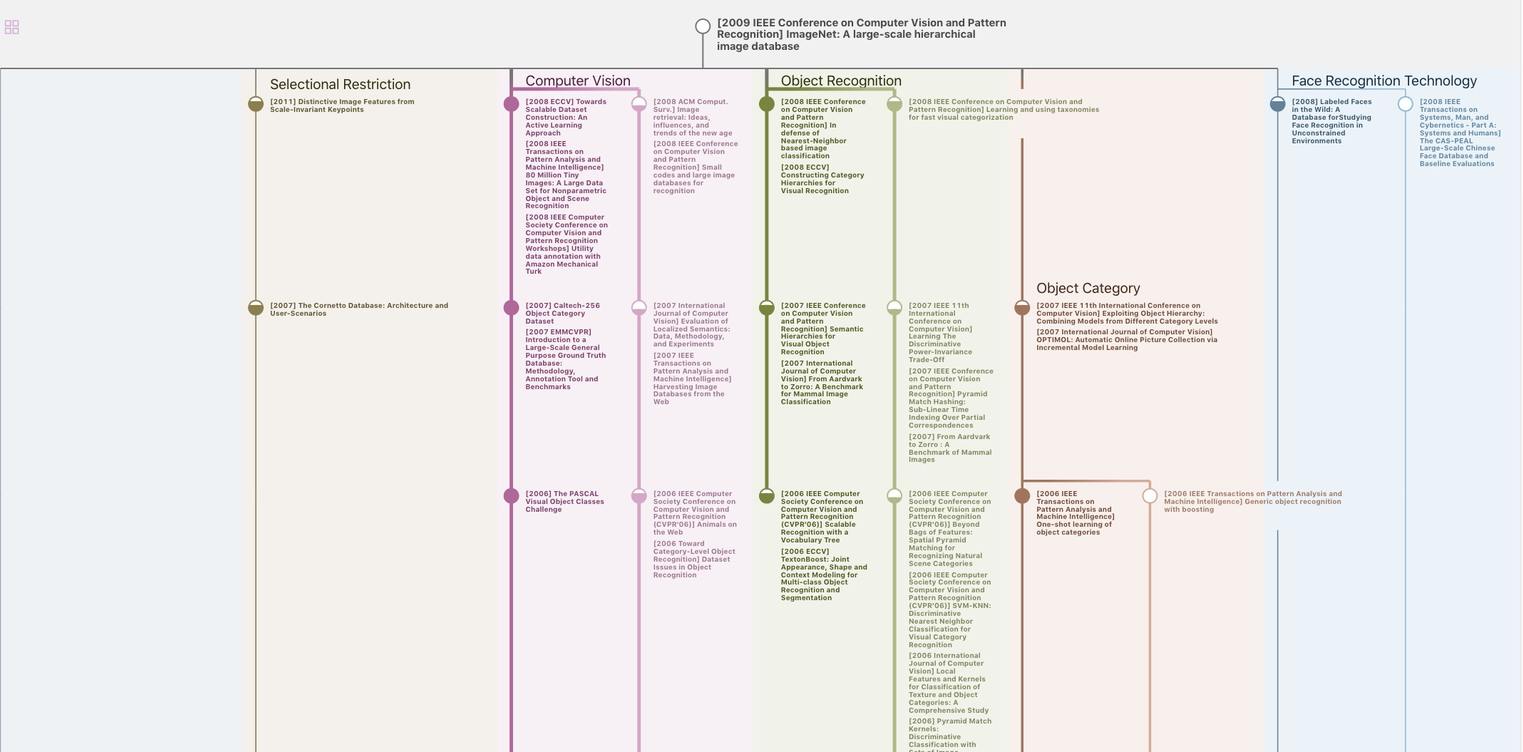
生成溯源树,研究论文发展脉络
Chat Paper
正在生成论文摘要