Velocity Planning for Multi-Vehicle Systems via Distributed Optimization.
2023 IEEE 26th International Conference on Intelligent Transportation Systems (ITSC)(2023)
摘要
This work presents a distributed velocity planning strategy for multi-vehicle cooperation along pre-defined paths. Specifically, we consider a class of tasks where multiple vehicles must navigate given paths with conflict zones (e.g., merging and crossing) as fast as possible without any inner collisions. Given the paths, the biggest challenge for velocity planning is to create collision avoidance constraints without complete spatio-temporal information. To overcome the challenge, a scheme is proposed to project collision-related information from coordinate space to 1-D space with geometry-based safety guarantees. To enhance the ability to deal with medium-and large-scale problems, the alternating direction method of multipliers (ADMM) is introduced. Unlike classic ideas of applying distributed optimization, we formulate ADMM in a semi-centralized, semi-parallel fashion instead of a fully distributed fashion. In this way, a trade-off between overall performance and computational efficiency can be achieved. We evaluate our distributed planning strategy through simulations in multiple cases
1
1
Video of the results is available at https://youtu.be/GR6BwFTLErw. The results demonstrate that our method not only plans collision-free paths but also balances between overall performance and computational load.
更多查看译文
关键词
Multi-vehicle Systems,Velocity Planning,Complete Information,Computational Load,Distribution Strategy,Conflict Zones,Multiple Vehicles,Safety Guarantees,Optimization Problem,Objective Function,Computation Time,Control Efforts,Path Planning,3D Coordinates,Model Predictive Control,Safe Distance,Narrow Space,Dual Problem,Linear Case,Trajectory Planning,Collision-free Trajectory,Total Steps,Nonlinear Path,Collision Zone,Motion Constraints,Augmented Lagrangian Function
AI 理解论文
溯源树
样例
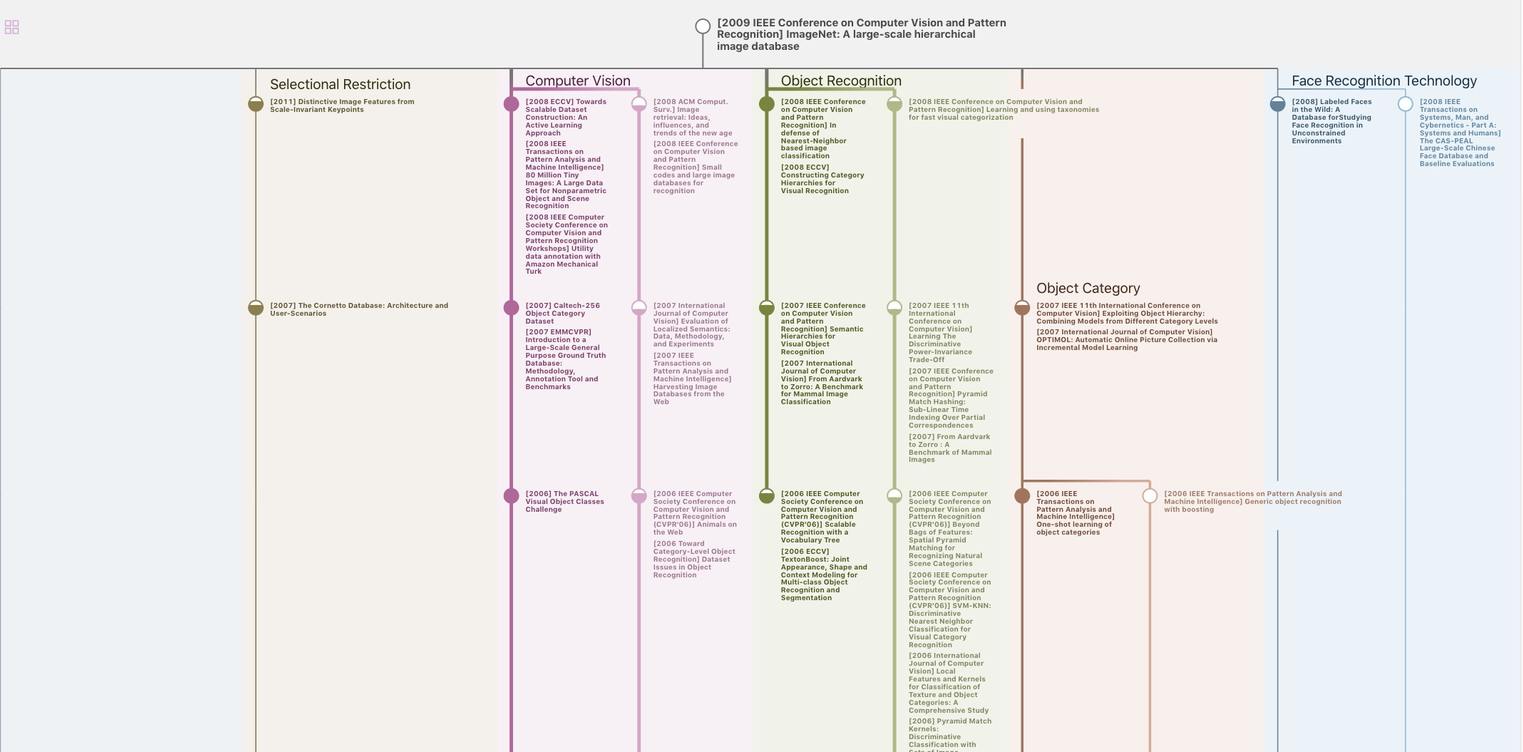
生成溯源树,研究论文发展脉络
Chat Paper
正在生成论文摘要