An adaptive federated learning framework for intelligent road surface classification.
2023 IEEE 26th International Conference on Intelligent Transportation Systems (ITSC)(2023)
摘要
The type of road surface directly influences the way vehicles are driven. The quality of the road surface also directly impacts on the comfort and especially on the safety of road users. Additionally, the type and the quality of the road surface affect fuel costs and vehicle maintenance. Following the above, the study of road surface is critical in improving traffic safety and fuel efficiency. Existing road surface classifications systems mainly process data on clouds, however, they are not able to warn drivers or/and other road users timely due to the long latency. In this paper, an intelligent road surface classification analysis is proposed via the implementation of a novel federated edge-cloud learning-based framework. That minimizes the risks associated with misuse of sensitive user information. This fosters trust in the system and ensures that user data is protected while still benefiting from the collaborative learning process. The proposed framework is called FLRSC and the federated learning strategy is based on the model learning from different edges with limited datasets. Simulation results demonstrate that FLRSC achieves a high detection performance and provides fast responses while covering a wider area, even when some edges have limited data.
更多查看译文
关键词
neural network,machine learning,federated learning,road surface classification
AI 理解论文
溯源树
样例
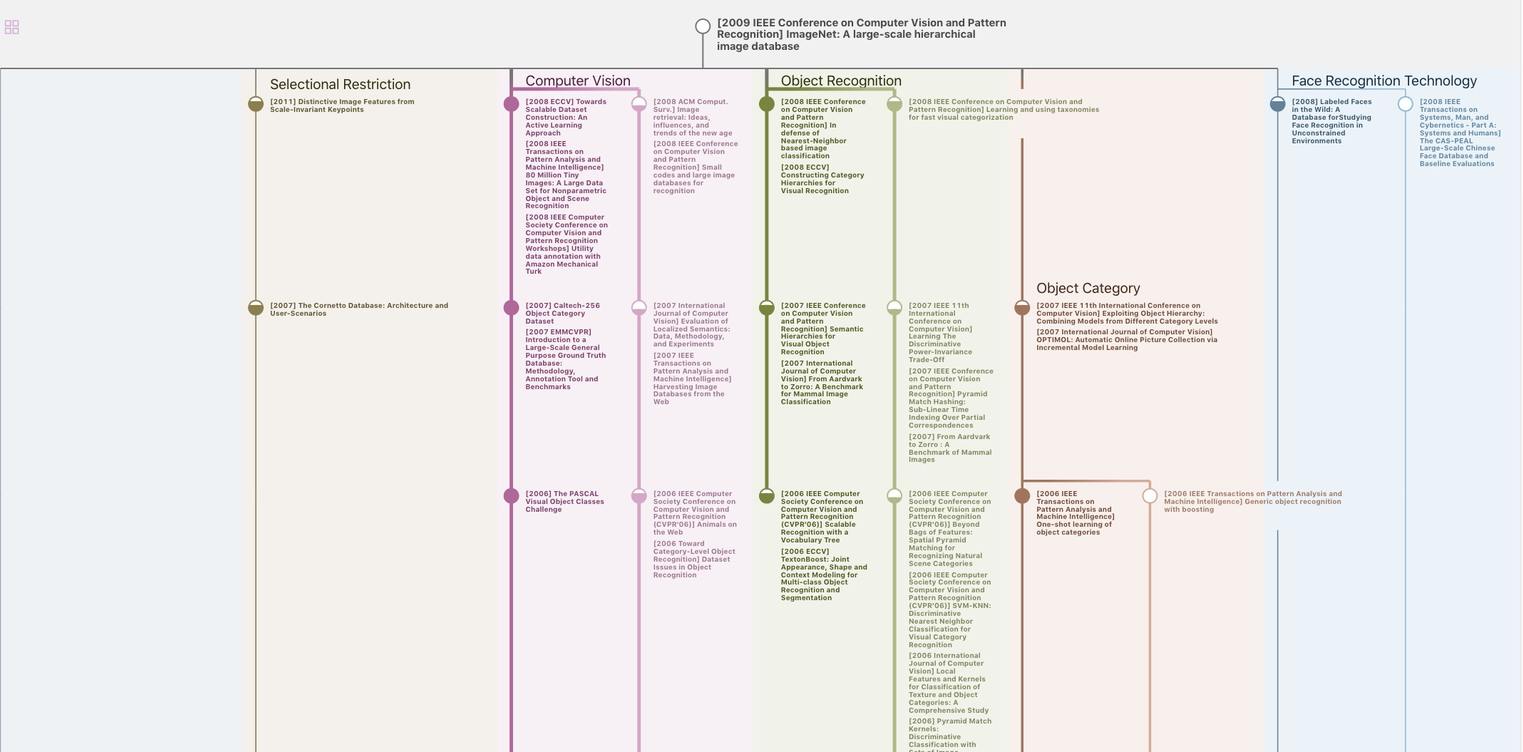
生成溯源树,研究论文发展脉络
Chat Paper
正在生成论文摘要