Transformer based Multi-modal Memory-augmented Masked Network for Air Crisis Event Detection.
2023 IEEE 26th International Conference on Intelligent Transportation Systems (ITSC)(2023)
摘要
In the social media era where the public is living surrounded by information in multiple modalities (e.g., images, videos, and texts), any air crisis event has the potential to draw global attention and provoke panic and anxiety among the public, making air crisis detection the core module of air accident management. This paper introduces the air crisis detection issue with a novel multi-modal formulation and proposes a Transformer based multi-modal memory-augmented masked network. Firstly, we leverage fine-grained features from both images and texts while filtering out redundant information across the modalities without solely relying on pairwise similarities, so as to achieve better event classification performance. Secondly, a specific multi-modal dataset with texts and images collected from Twitter for this issue is constructed, named Air-CrisisMMD, which could be used for benchmark testing. The experimental results on the two datasets (the Air-CrisisMMD and the publicly available CrisisMMD) showed that the proposed achieves 93.39% and 96.67% detection accuracy, outperforming the state-of-the-art baseline methods for crisis event detection, which demonstrates the potential of the proposed to provide an effective tool for improving accident management strategies and supporting aviation safety.
更多查看译文
关键词
accident management,air crisis event detection,deep multi-modal learning,social media
AI 理解论文
溯源树
样例
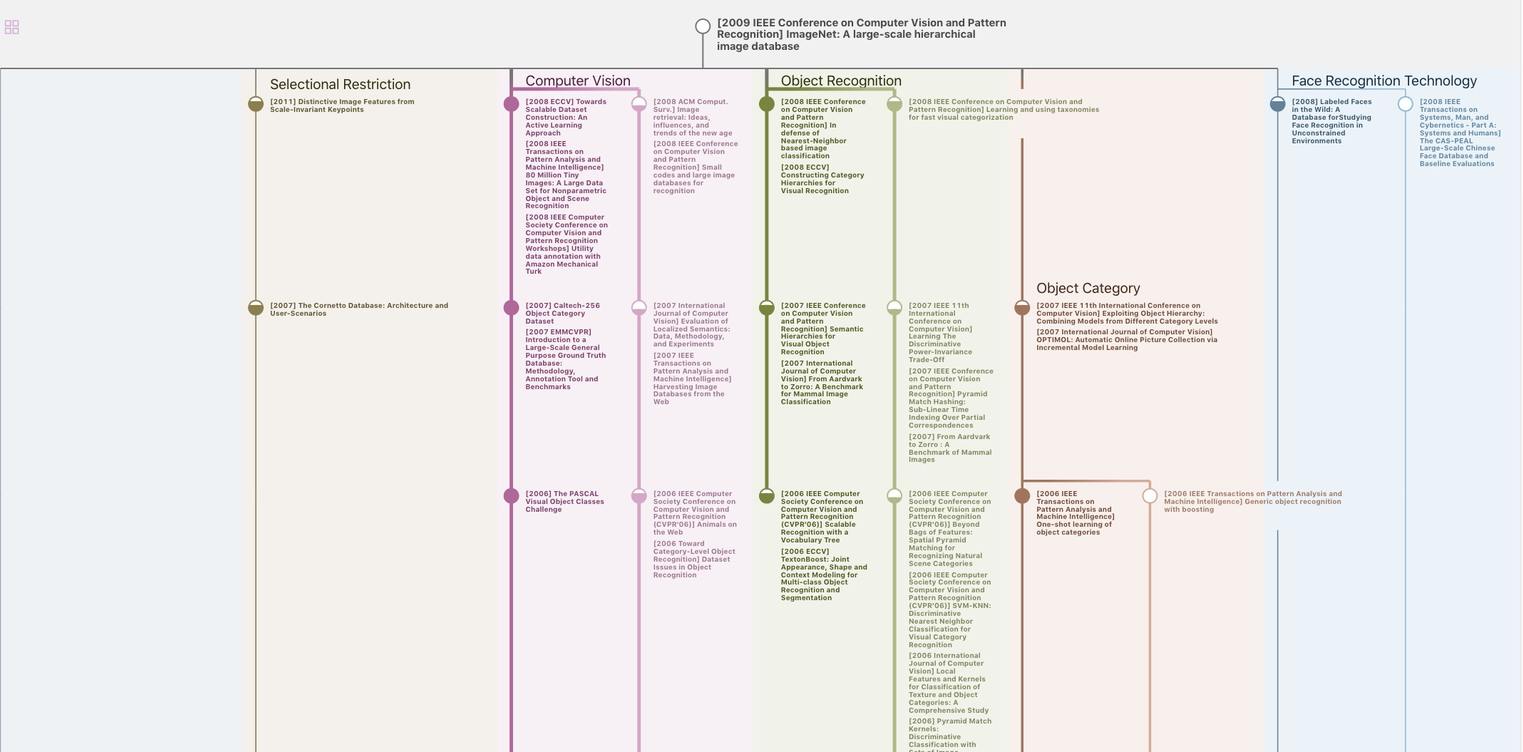
生成溯源树,研究论文发展脉络
Chat Paper
正在生成论文摘要