TP-GDM: Trajectory Prediction-based Game Decision-Making Model and A Case Study on Merging.
2023 IEEE 26th International Conference on Intelligent Transportation Systems (ITSC)(2023)
摘要
The driving task of autonomous vehicles in urban poses a significant challenge, especially in the merging scenarios with strong interaction and high requirement of real-time. However, the existing driving decision-making methods generally separate the motion prediction of surrounding vehicles from the decision-making of ego vehicle, which is not aligned with human drivers and may generate unreasonable merging behavior. To address this challenge, a merging decision-making framework is proposed, which involves a Mixer based Trajectory Prediction Model (Mixer-TPM) and a Trajectory Prediction-based Game-theory Decision-Making model (TP-GDM). The decision-making model takes into account the future trajectory of interactive vehicles as an input feature and ensures future safety. Finally, both Mixer-TPM and TP-GDM are validated using public naturalistic driving datasets. The results indicate that the Mixer-TPM can improves prediction accuracy significantly compared to the state-of-the-arts and has better computational efficiency. Additionally, TP-GDM shows a high level of similarity to human drivers.
更多查看译文
关键词
trajectory prediction,decision making,merging behavior,M-Mixer,game theory
AI 理解论文
溯源树
样例
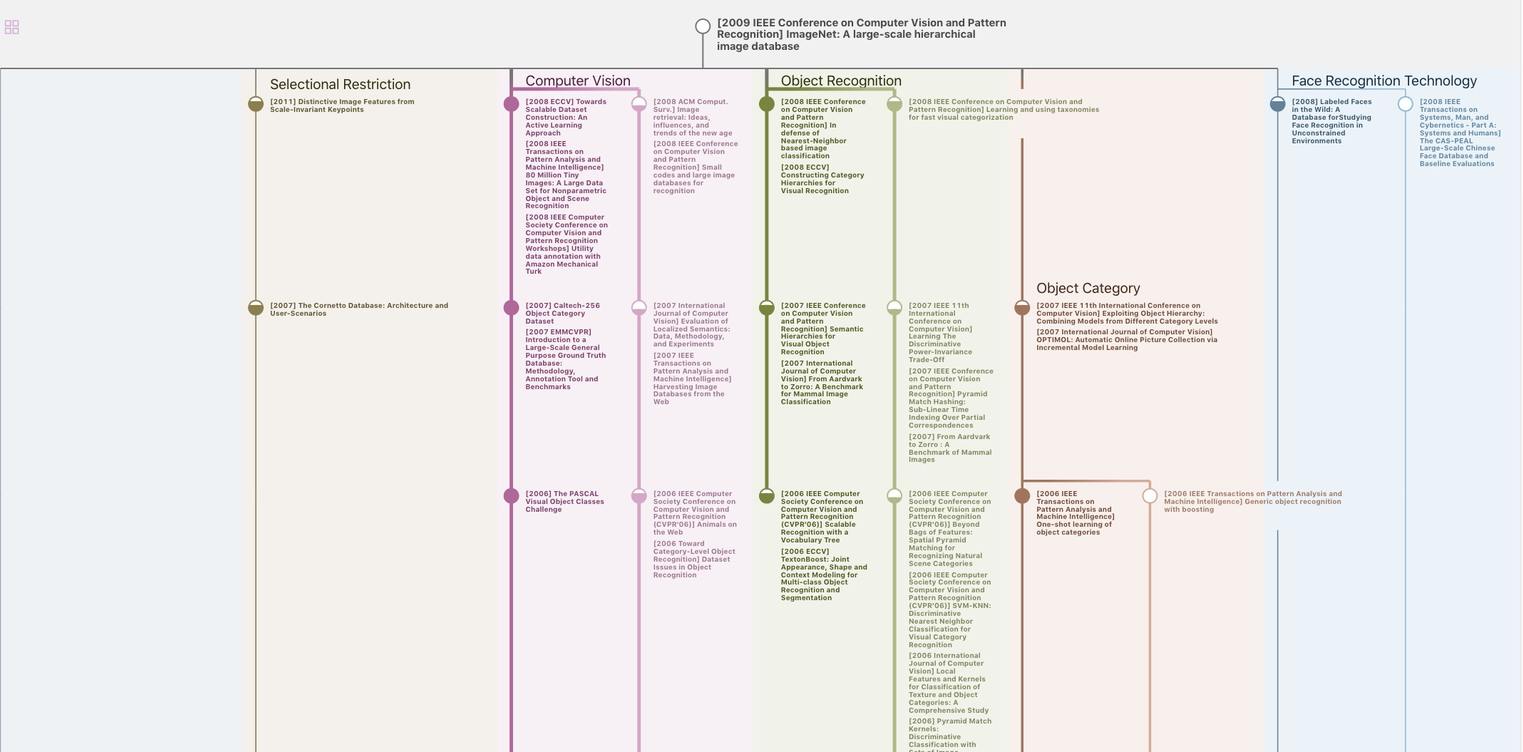
生成溯源树,研究论文发展脉络
Chat Paper
正在生成论文摘要