Bayesian active learning with model selection for spectral experiments
Scientific Reports(2024)
摘要
Active learning is a common approach to improve the efficiency of spectral experiments. Model selection from the candidates and parameter estimation are often required in the analysis of spectral experiments. Therefore, we proposed an active learning with model selection method using multiple parametric models as learning models. Important points for model selection and its parameter estimation were actively measured using Bayesian posterior distribution. The present study demonstrated the effectiveness of our proposed method for spectral deconvolution and Hamiltonian selection in X-ray photoelectron spectroscopy.
更多查看译文
AI 理解论文
溯源树
样例
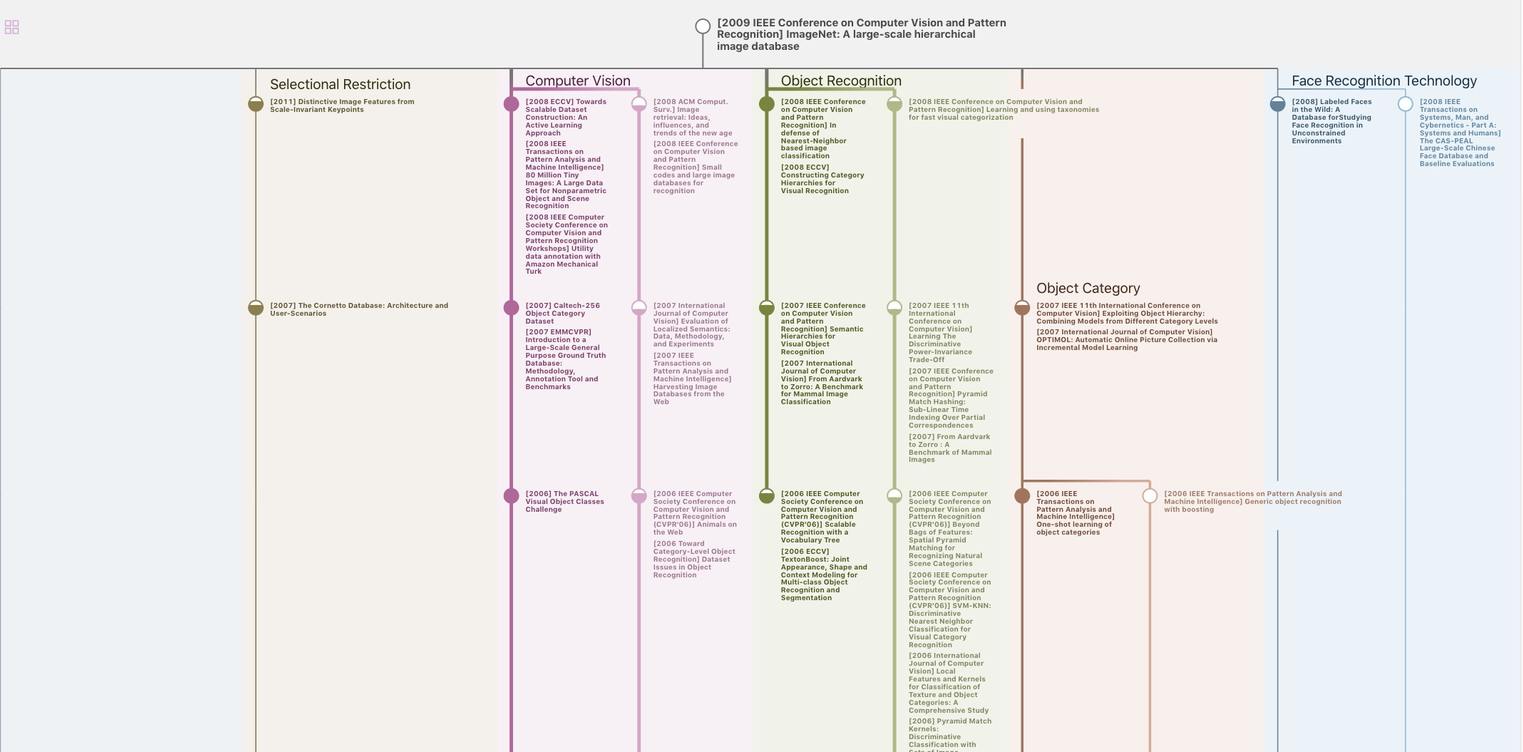
生成溯源树,研究论文发展脉络
Chat Paper
正在生成论文摘要