A deep-learning-based framework for identifying and localizing multiple abnormalities and assessing cardiomegaly in chest X-ray
Nature Communications(2024)
摘要
Accurate identification and localization of multiple abnormalities are crucial steps in the interpretation of chest X-rays (CXRs); however, the lack of a large CXR dataset with bounding boxes severely constrains accurate localization research based on deep learning. We created a large CXR dataset named CXR-AL14, containing 165,988 CXRs and 253,844 bounding boxes. On the basis of this dataset, a deep-learning-based framework was developed to identify and localize 14 common abnormalities and calculate the cardiothoracic ratio (CTR) simultaneously. The mean average precision values obtained by the model for 14 abnormalities reached 0.572-0.631 with an intersection-over-union threshold of 0.5, and the intraclass correlation coefficient of the CTR algorithm exceeded 0.95 on the held-out, multicentre and prospective test datasets. This framework shows an excellent performance, good generalization ability and strong clinical applicability, which is superior to senior radiologists and suitable for routine clinical settings.
更多查看译文
AI 理解论文
溯源树
样例
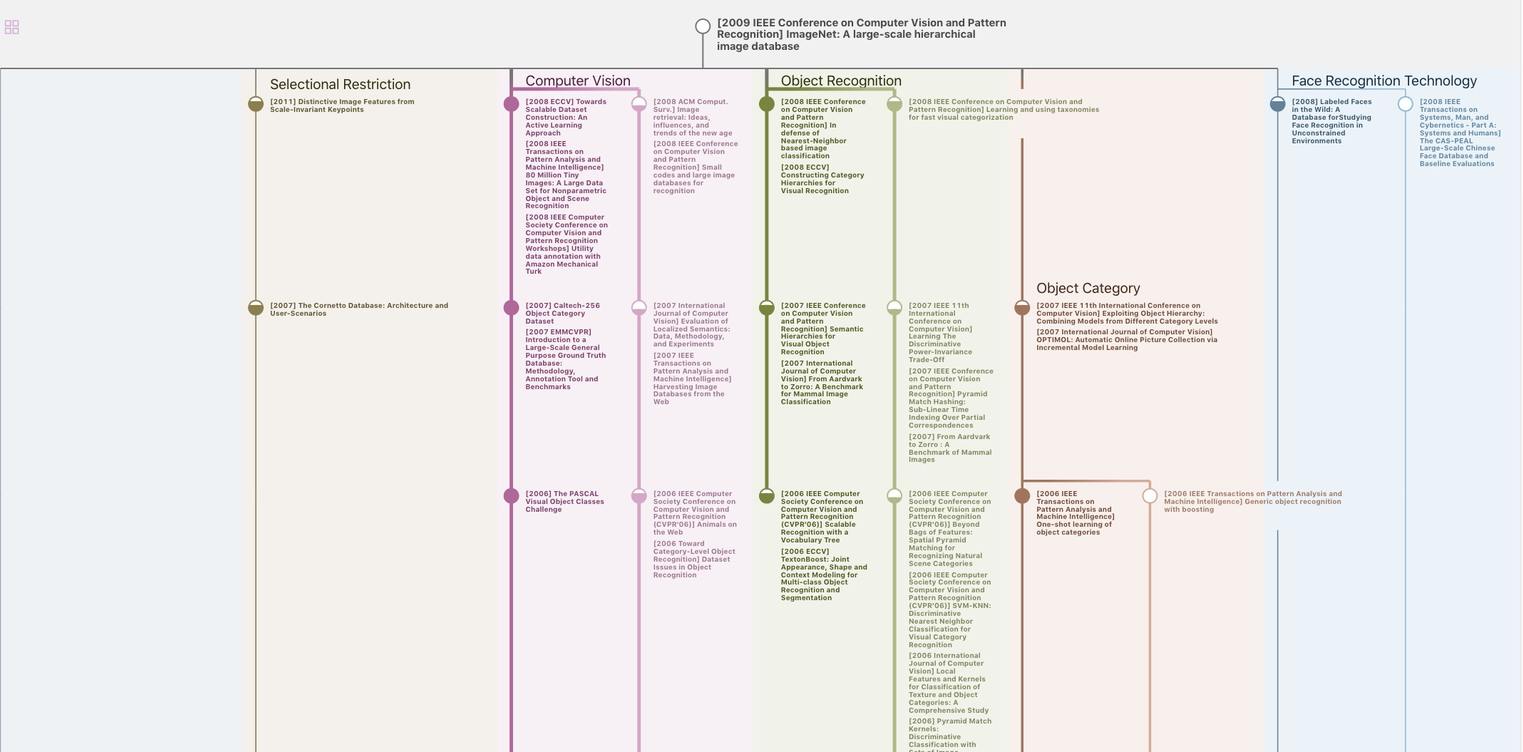
生成溯源树,研究论文发展脉络
Chat Paper
正在生成论文摘要