Learning-Based MPC With Safety Filter for Constrained Deformable Linear Object Manipulation
IEEE ROBOTICS AND AUTOMATION LETTERS(2024)
摘要
Deformable linear object (DLO) manipulation in constrained environments with obstacles has received limited investigations due to DLO's complex intrinsic deformation. In this study, we focus on addressing constrained DLO manipulation problems, especially in the context of avoiding cluttered environment obstacles. Unlike sampling-based planners, which struggle with the high-dimensional state space or require modifications to ensure DLO's kinematic feasibility, we propose a novel obstacle avoidance approach by combining a learning-based predictive control method and an efficient control-theoretic technique. Specifically, we utilize a learning-based model predictive control (MPC) strategy with an attention-based global deformation model to generate low-dimensional reference actions that inherently align with DLO's physics. The attention-based model outperforms multilayer perceptron and bi-directional long short-term memory models by capturing contextual relationships among feature points on DLOs. To mitigate the inevitable modeling errors, a safety-critical filter is designed based on the control barrier function (CBF) principle. An online local linear model is employed in the filter to steer clear of obstacles in close proximity. The proposed approach was validated with extensive simulations and physical experiments on constrained DLO manipulation tasks.
更多查看译文
关键词
Deformation,Deformable models,Task analysis,Predictive models,Safety,Collision avoidance,Robots,Deformable object manipulation,model learning,predictive control,dexterous manipulation
AI 理解论文
溯源树
样例
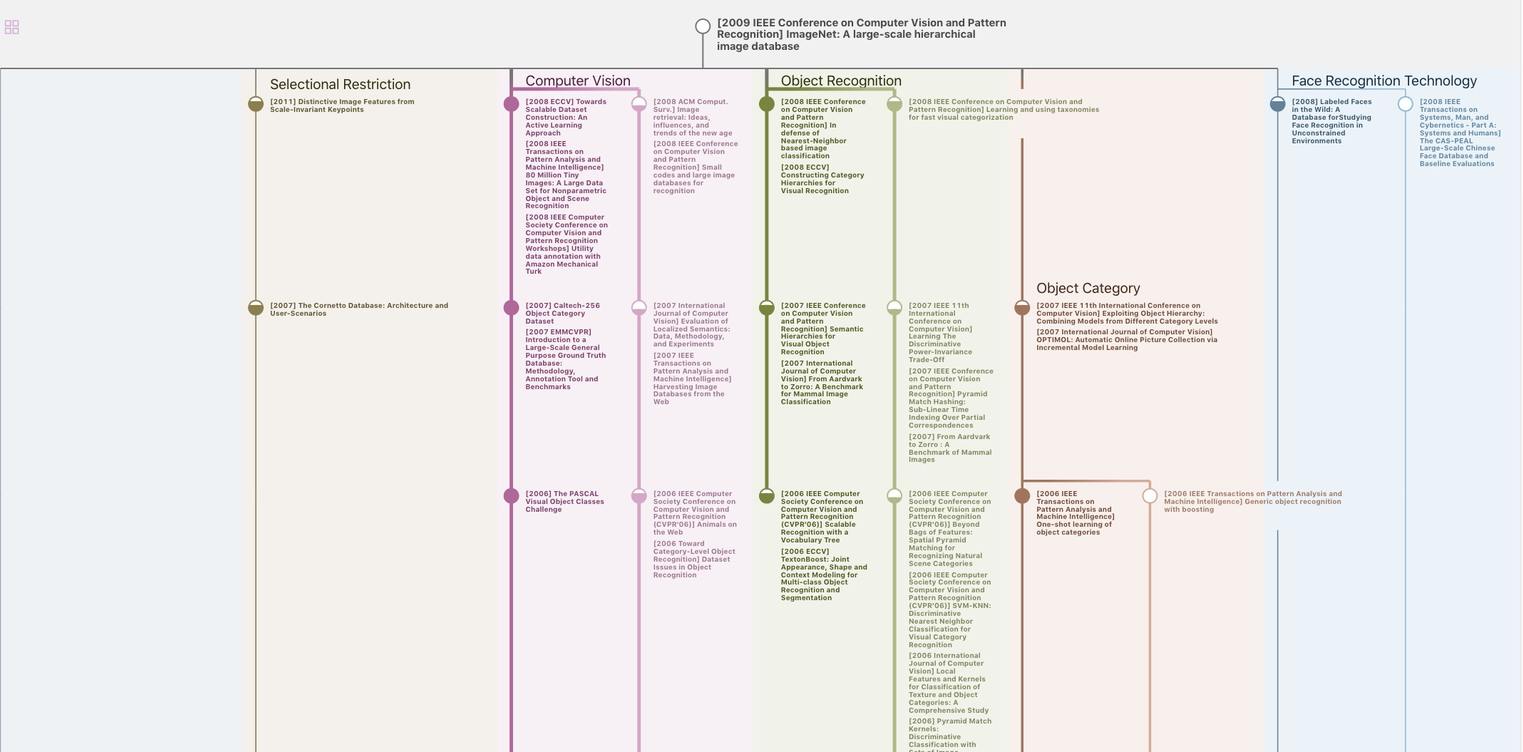
生成溯源树,研究论文发展脉络
Chat Paper
正在生成论文摘要