Few-Shot Fine-Grained Classification With Rotation-Invariant Feature Map Complementary Reconstruction Network
IEEE TRANSACTIONS ON GEOSCIENCE AND REMOTE SENSING(2024)
摘要
Fine-grained classification is of significant importance in the field of remote sensing. However, obtaining valuable and rare target images is often a challenging task, giving rise to the few-shot fine-grained classification problem. In response to this challenge, various meta-learning approaches have been introduced, with the feature map reconstruction network (FRN) emerging as a prominent method. Targets in remote-sensing images exhibit arbitrary orientation, substantial interclass similarity, and intraclass diversity. Nevertheless, the conventional FRN exhibits subpar performance due to its inability to handle rotational variations. Moreover, it only reconstructs features from a single-channel dimension of support features, neglecting the interplay between different dimensions and resulting in inaccurate reconstruction errors. To overcome the challenges of imprecise rotational variation features for reconstruction and inaccurate reconstruction errors, we propose a rotation-invariant feature map complementary reconstruction network (RIFCRN). The RIFCRN involves several key innovations. First, we introduce a novel rotation-invariant module (RIM) based on active rotating filters (ARFs) and oriented response pooling, enabling the extraction of rotation-invariant features for reconstruction. This modification enhances the suitability of the FRN for the few-shot fine-grained classification problem. Second, we put forward a novel feature map complementary reconstruction (CPR) method that calculates the CPR errors (CREs) which effectively captures relationships among different feature map dimensions and results in more accurate reconstruction errors. Finally, extensive experiments have been conducted to validate the effectiveness of the proposed RIFCRN in addressing the few-shot fine-grained classification problem. The code will be available at https://github.com/liyangfan0/RIFCRN.
更多查看译文
关键词
Feature map complementary reconstruction (CPR),few-shot learning,fine-grained classification
AI 理解论文
溯源树
样例
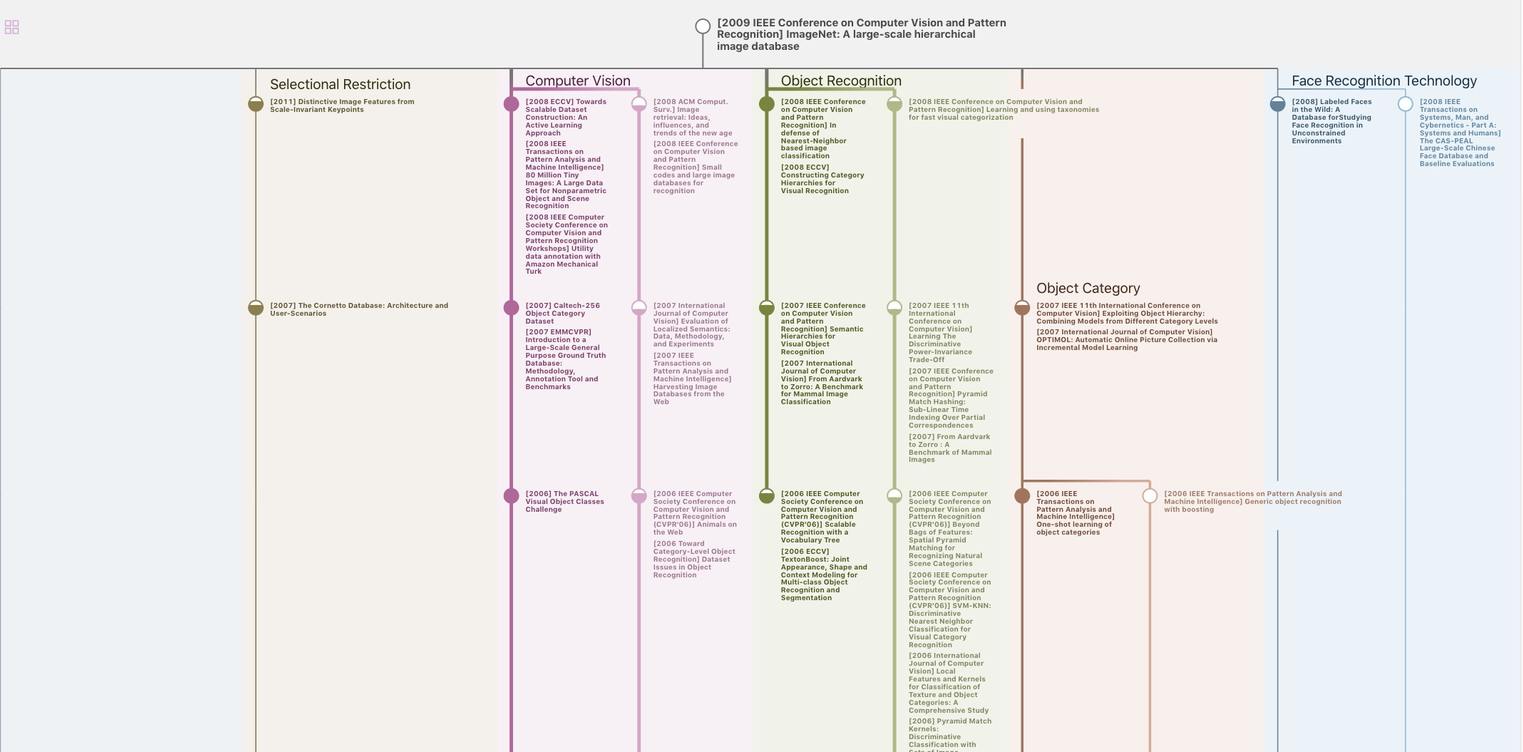
生成溯源树,研究论文发展脉络
Chat Paper
正在生成论文摘要