Uncertainty Quantification via Stable Distribution Propagation
CoRR(2024)
摘要
We propose a new approach for propagating stable probability distributions
through neural networks. Our method is based on local linearization, which we
show to be an optimal approximation in terms of total variation distance for
the ReLU non-linearity. This allows propagating Gaussian and Cauchy input
uncertainties through neural networks to quantify their output uncertainties.
To demonstrate the utility of propagating distributions, we apply the proposed
method to predicting calibrated confidence intervals and selective prediction
on out-of-distribution data. The results demonstrate a broad applicability of
propagating distributions and show the advantages of our method over other
approaches such as moment matching.
更多查看译文
关键词
propagating distributions,uncertainty,uncertainties,aleatoric,epistemic,moment matching,total variation,sampling-free,deterministic,variational inference,propagation,probabilistic neural networks,variance propagation,Cauchy,Cauchy distribution,Gaussian,analytical,data uncertainty,alpha stable
AI 理解论文
溯源树
样例
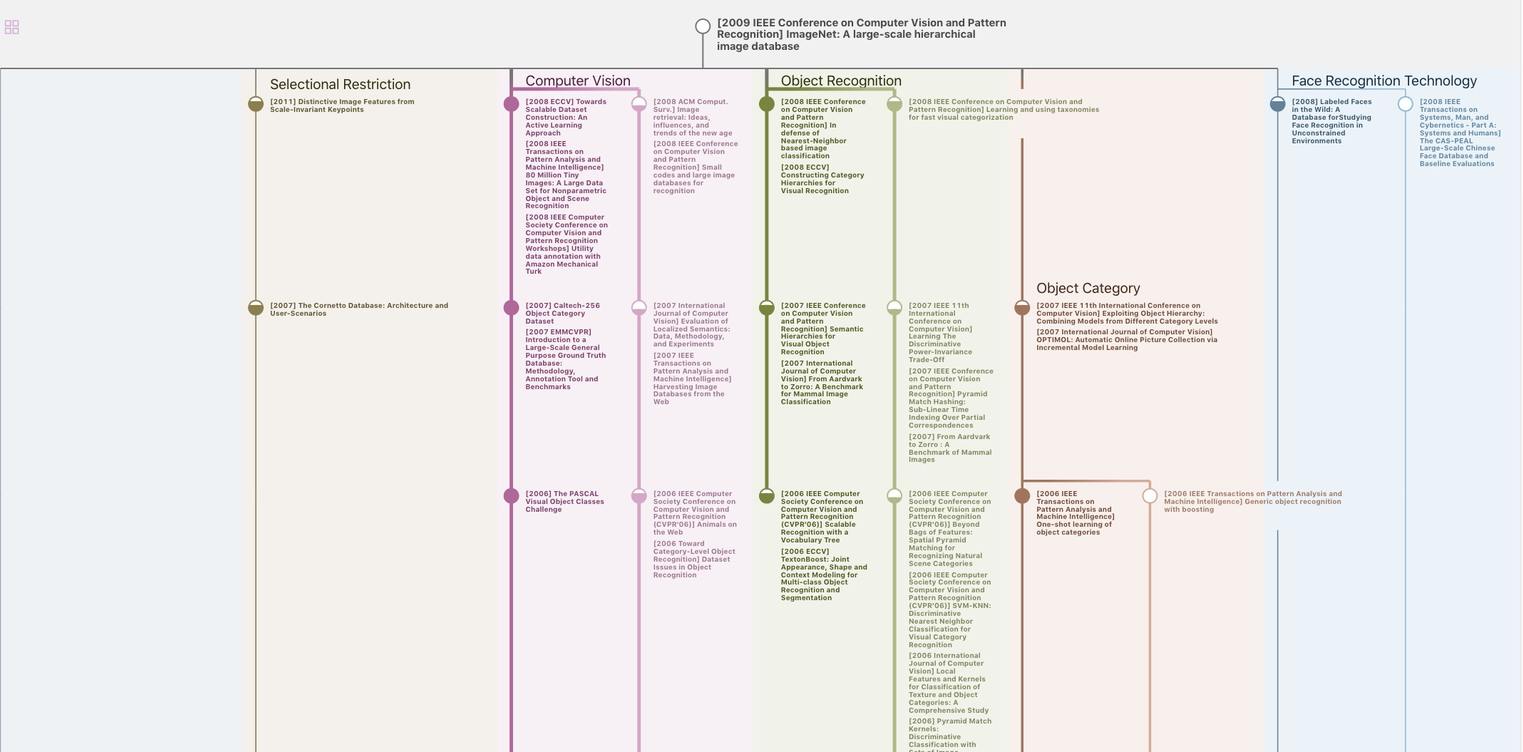
生成溯源树,研究论文发展脉络
Chat Paper
正在生成论文摘要