On the Self-Verification Limitations of Large Language Models on Reasoning and Planning Tasks
CoRR(2024)
摘要
There has been considerable divergence of opinion on the reasoning abilities
of Large Language Models (LLMs). While the initial optimism that reasoning
might emerge automatically with scale has been tempered thanks to a slew of
counterexamples–ranging from multiplication to simple planning–there persists
a wide spread belief that LLMs can self-critique and improve their own
solutions in an iterative fashion. This belief seemingly rests on the
assumption that verification of correctness should be easier than generation–a
rather classical argument from computational complexity–which should be
irrelevant to LLMs to the extent that what they are doing is approximate
retrieval. In this paper, we set out to systematically investigate the
effectiveness of iterative prompting in the context of reasoning and planning.
We present a principled empirical study of the performance of GPT-4 in three
domains: Game of 24, Graph Coloring, and STRIPS planning. We experiment both
with the model critiquing its own answers and with an external correct reasoner
verifying proposed solutions. In each case, we analyze whether the content of
criticisms actually affects bottom line performance, and whether we can ablate
elements of the augmented system without losing performance. We observe
significant performance collapse with self-critique, significant performance
gains with sound external verification, but that the content of critique
doesn't matter to the performance of the system. In fact, merely re-prompting
with a sound verifier maintains most of the benefits of more involved setups.
更多查看译文
AI 理解论文
溯源树
样例
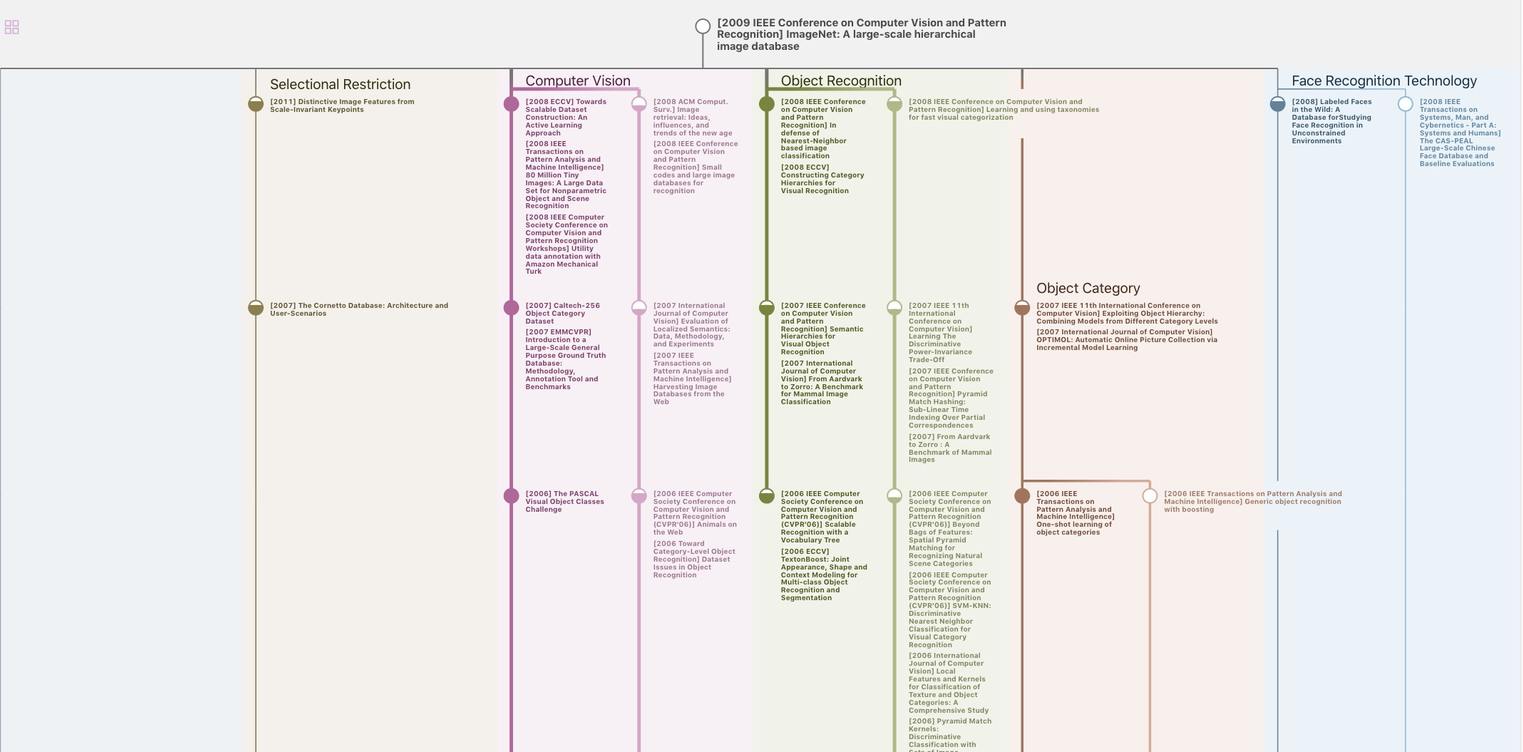
生成溯源树,研究论文发展脉络
Chat Paper
正在生成论文摘要