Comparing MoCA Performance in Parkinson's disease: Age and Education-Adjusted Cutoffs vs. Machine Learning.
Journal of movement disorders(2024)
摘要
Background and Objectives:The Montreal Cognitive Assessment (MoCA) is recommended for general cognitive evaluation in Parkinson's Disease (PD). However, age- and education-adjusted cutoffs specifically for PD have not been developed and systematically validated across PD cohorts with diverse education levels.
Methods:This retrospective analysis utilized data from 1,293 Korean patients with PD, where cognitive diagnoses were determined through comprehensive neuropsychological assessments. Age- and education-adjusted cutoffs were formulated based on 1,202 patients with PD. To identify the optimal machine learning model, clinical parameters and MoCA domain scores from 416 patients with PD were used. Comparative analyses between machine learning and different cutoffs were conducted on an additional 91 consecutive patients with PD.
Results:The cutoffs for cognitive impairment decrease with advancing age within the same education level. Similarly, lower education levels within the same age group correspond to lower cutoffs. For individuals aged 60-80, cutoffs were set as follows: 25 or 24 for those with over 12 years of education, 23 or 22 for 10-12 years, and 21 or 20 for 7-9 years. Comparisons between age- and education-adjusted cutoffs and the machine learning method showed comparable accuracies. The cutoff method demonstrated higher sensitivity (0.8627), whereas machine learning achieved higher specificity (0.8250).
Conclusions:Both the age- and education-adjusted cutoff method and machine learning demonstrated high effectiveness in detecting cognitive impairment in PD. This study highlights the necessity of tailored cutoffs and suggests the potential of machine learning to enhance cognitive assessments in PD.
更多查看译文
AI 理解论文
溯源树
样例
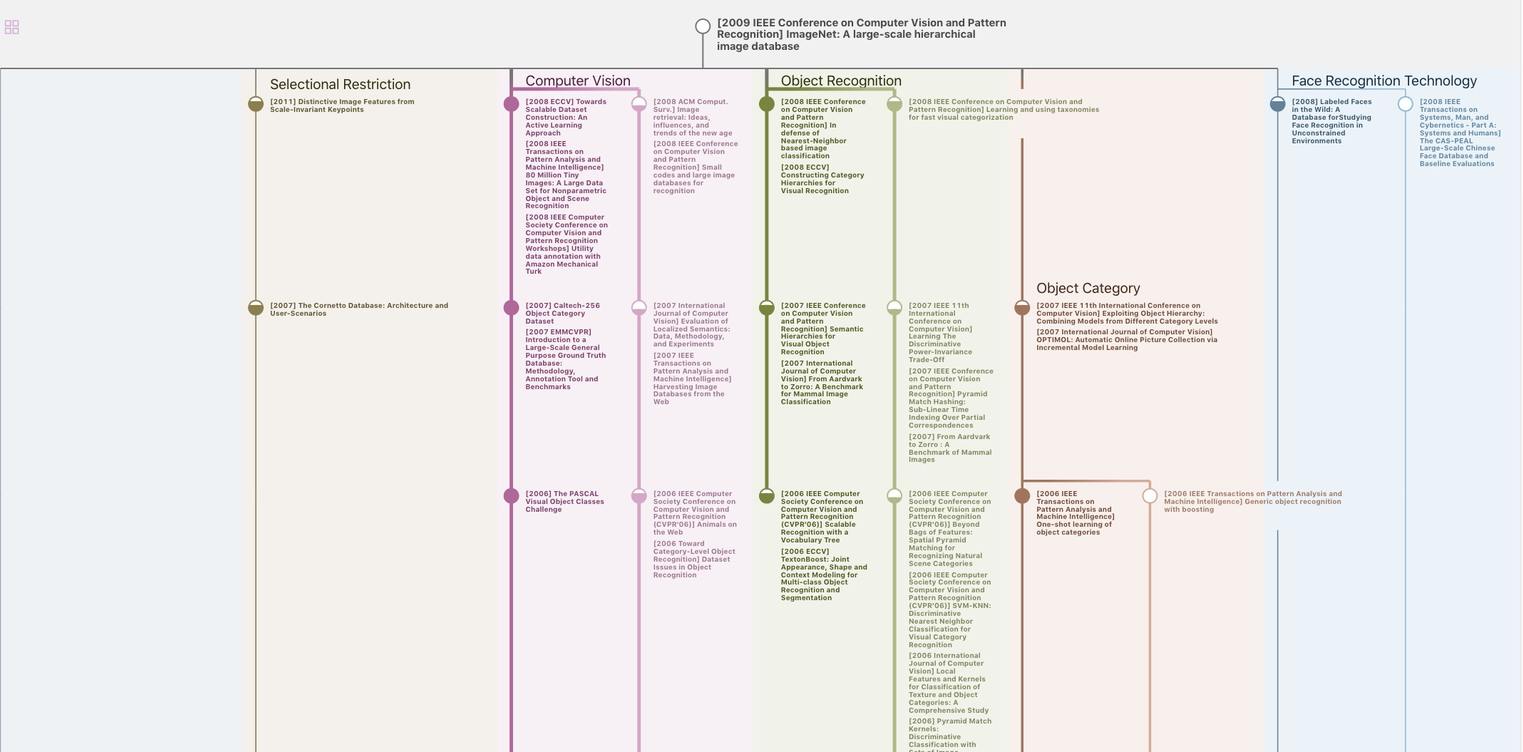
生成溯源树,研究论文发展脉络
Chat Paper
正在生成论文摘要